A Method for Breast Mass Segmentation Using Image Augmentation with SAM and Receptive Field Expansion.
ICCPR '23 Proceedings of the 2023 12th International Conference on Computing and Pattern Recognition(2024)
摘要
With the development of deep learning methods and their wide application in medical image segmentation tasks, mammography, used for early breast cancer screening, can further assist clinicians in diagnosis to a certain extent. Due to the loss of fine-grained features in the downsampling process and the very few available mammograms, the main methods for extracting masses from the whole mammography are relatively ineffective. In this paper, we propose a novel mass segmentation model for mammograms based on two U-Net network models, and integrate the Receptive Field Block (RFB) module in-between to enhance the deep features captured by the model. We augmented the input image using Segment Anything Model (SAM) and evaluated the proposed architecture on two public datasets, namely the Curated Breast Imaging Subset of Digital Database for Screening Mammography (CBIS-DDSM) and INBreast, as well as on a private dataset. The results show that the proposed model for segmenting masses in ROI regions can achieve high Dice scores of 92.18% and 89.85%, and Intersection over Union (IoU) scores of 85.47% and 80.80% on both INBreast and the private dataset. In addition, our model for segmenting masses on whole mammographs in CBIS-DDSM dataset can achieve Dice scores of 58.10% and 41.96% IoU scores. Besides, our model performance improved to Dice score 61.78% and IoU score 43.00% on whole mammographs in private dataset using SAM-augmented input images.
更多查看译文
AI 理解论文
溯源树
样例
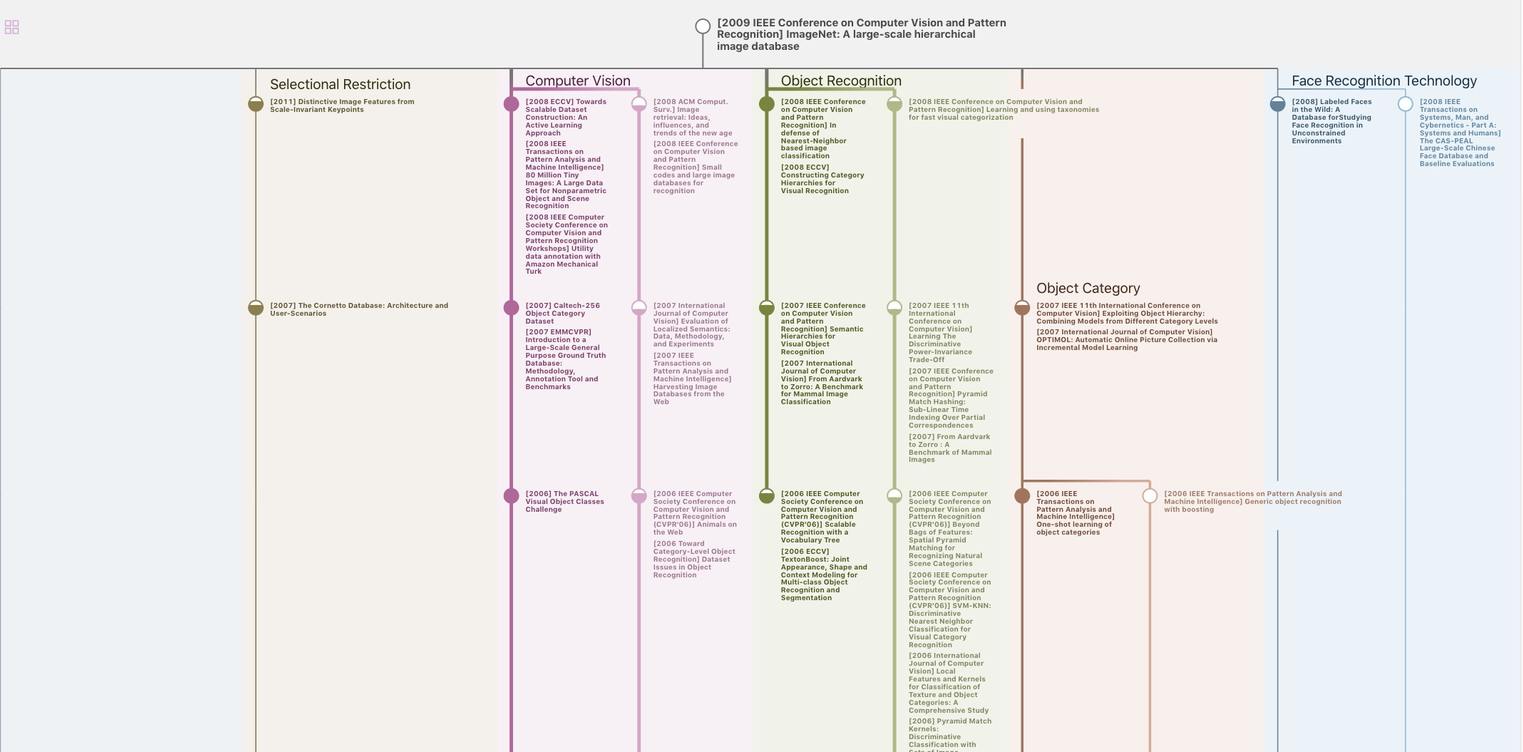
生成溯源树,研究论文发展脉络
Chat Paper
正在生成论文摘要