FADL-Net: Frequency-Assisted Dynamic Learning Network for Oriented Object Detection in Remote Sensing Images
IEEE Transactions on Industrial Informatics(2024)
摘要
In the field of Earth observation and computer vision, oriented object detection for remotesensing images is a crucial task that aims to locate objects more accurately in complex scenes containing a large number of densely arranged, large aspect ratio, and arbitrarily oriented objects. Although recently proposed methods have achieved remarkable performance, there are still several challenges to address: 1) interference from complex backgrounds, 2) imbalanced and mismatched label assignments caused by tiny objects and objects with large aspect ratios, and 3) misalignment between the tasks of classification and localization. In this article, we propose a frequency-assisted dynamic learning network (FADL-Net) to overcome the crucial challenges. Concretely, we introduce a spatial-spectral feature pyramid network to adaptively capture global long-range dependency feature representations containing various frequency domains. Meanwhile, to produce more reliable training samples for objects with extreme shapes, we design a geometric aware dynamic label assignment to dynamically mitigate the imbalance and mismatch in label assignment in a coarse-to-fine manner, thereby achieving more stable optimization during the training process. Moreover, we propose a joint-learning rotated quality loss that addresses the inconsistency between classification and localization by dynamically adapting the weights of different samples in the training stage. Extensive experiments on several public remote sensing datasets demonstrate that our method performs favorably against state-of-the-art detection approaches.
更多查看译文
关键词
Feature representation,label assignment,oriented object detection,remote sensing
AI 理解论文
溯源树
样例
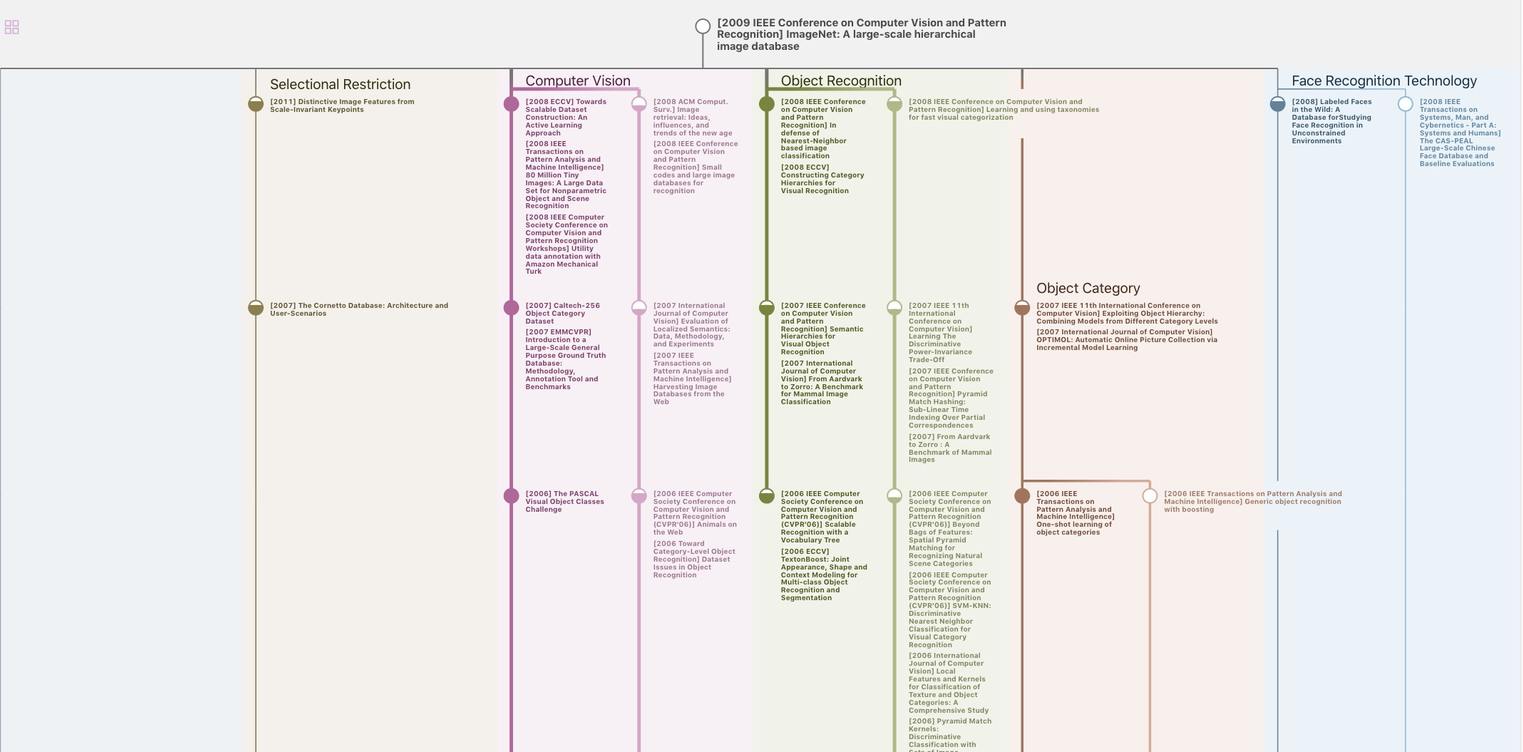
生成溯源树,研究论文发展脉络
Chat Paper
正在生成论文摘要