Low-rank Matrix Bandits with Heavy-tailed Rewards
arxiv(2024)
摘要
In stochastic low-rank matrix bandit, the expected reward of an arm is equal
to the inner product between its feature matrix and some unknown d_1 by d_2
low-rank parameter matrix Θ^* with rank r ≪ d_1∧ d_2. While all
prior studies assume the payoffs are mixed with sub-Gaussian noises, in this
work we loosen this strict assumption and consider the new problem of
low-rank matrix bandit with heavy-tailed
rewards (LowHTR), where the rewards only have finite (1+δ)
moment for some δ∈ (0,1]. By utilizing the truncation on observed
payoffs and the dynamic exploration, we propose a novel algorithm called LOTUS
attaining the regret bound of order Õ(d^3/2r^1/2T^1/1+δ/D̃_rr) without
knowing T, which matches the state-of-the-art regret bound under sub-Gaussian
noises with δ = 1. Moreover, we
establish a lower bound of the order Ω(d^δ/1+δ
r^δ/1+δ T^1/1+δ) = Ω(T^1/1+δ)
for LowHTR, which indicates our LOTUS is nearly optimal in the order of T. In
addition, we improve LOTUS so that it does not require knowledge of the rank
r with Õ(dr^3/2T^1+δ/1+2δ) regret bound,
and it is efficient under the high-dimensional scenario. We also conduct
simulations to demonstrate the practical superiority of our algorithm.
更多查看译文
AI 理解论文
溯源树
样例
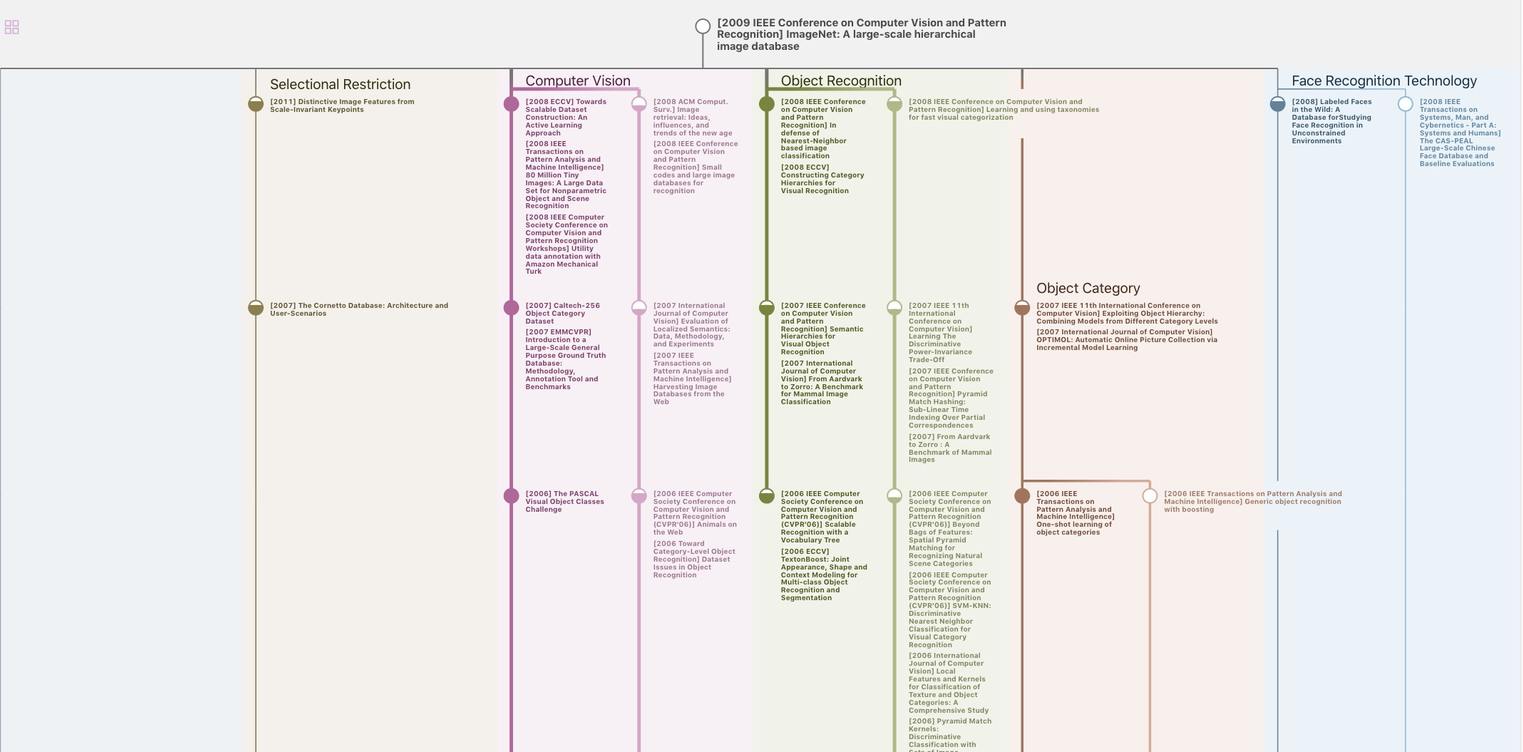
生成溯源树,研究论文发展脉络
Chat Paper
正在生成论文摘要