Solving Partial Differential Equations with Equivariant Extreme Learning Machines
arxiv(2024)
摘要
We utilize extreme-learning machines for the prediction of partial
differential equations (PDEs). Our method splits the state space into multiple
windows that are predicted individually using a single model. Despite requiring
only few data points (in some cases, our method can learn from a single
full-state snapshot), it still achieves high accuracy and can predict the flow
of PDEs over long time horizons. Moreover, we show how additional symmetries
can be exploited to increase sample efficiency and to enforce equivariance.
更多查看译文
AI 理解论文
溯源树
样例
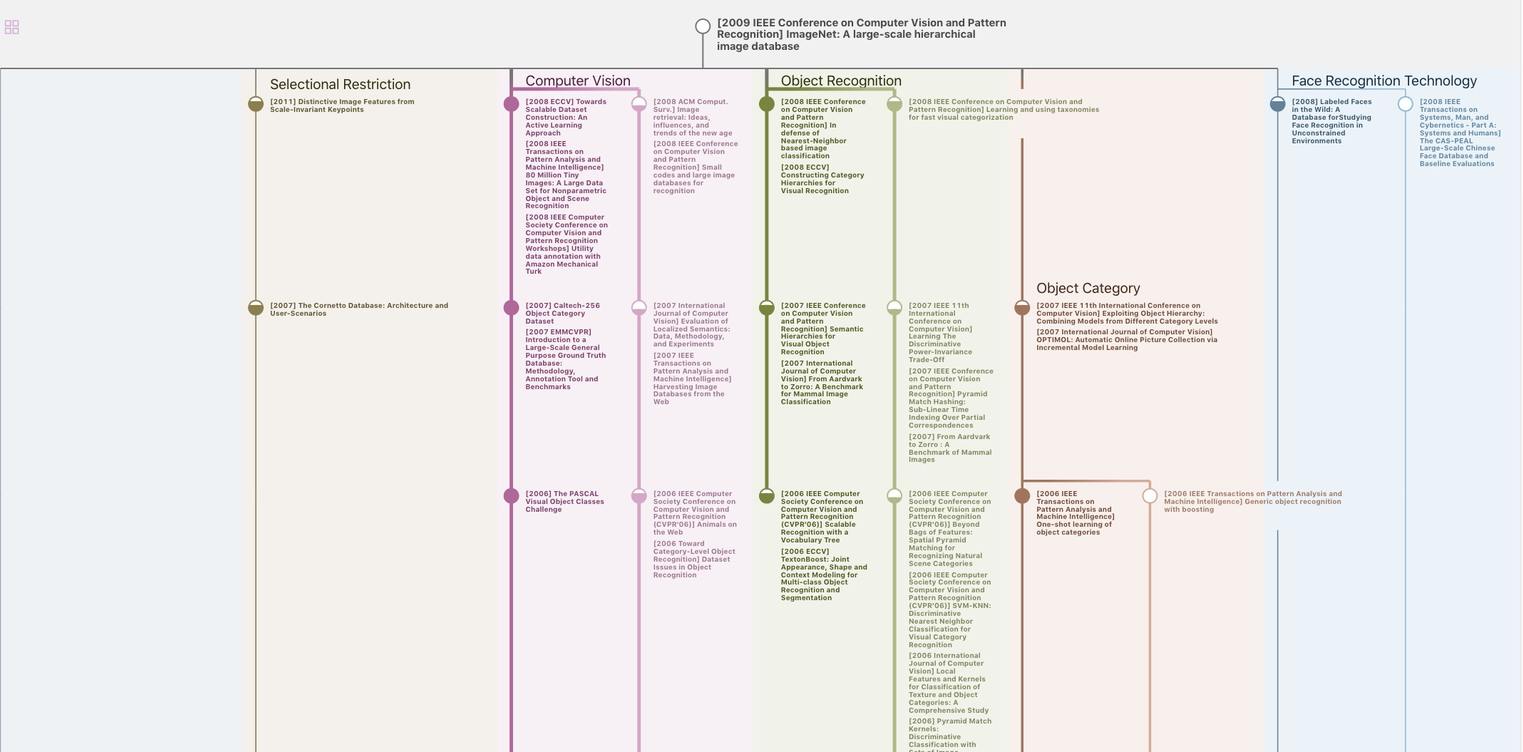
生成溯源树,研究论文发展脉络
Chat Paper
正在生成论文摘要