DPO Meets PPO: Reinforced Token Optimization for RLHF
CoRR(2024)
摘要
In the classical Reinforcement Learning from Human Feedback (RLHF) framework,
Proximal Policy Optimization (PPO) is employed to learn from sparse,
sentence-level rewards – a challenging scenario in traditional deep
reinforcement learning. Despite the great successes of PPO in the alignment of
state-of-the-art closed-source large language models (LLMs), its open-source
implementation is still largely sub-optimal, as widely reported by numerous
research studies. To address these issues, we introduce a framework that models
RLHF problems as a Markov decision process (MDP), enabling the capture of
fine-grained token-wise information. Furthermore, we provide theoretical
insights that demonstrate the superiority of our MDP framework over the
previous sentence-level bandit formulation. Under this framework, we introduce
an algorithm, dubbed as Reinforced Token Optimization (), which
learns the token-wise reward function from preference data and performs policy
optimization based on this learned token-wise reward signal. Theoretically,
is proven to have the capability of finding the near-optimal
policy sample-efficiently. For its practical implementation,
innovatively integrates Direct Preference Optimization (DPO) and PPO. DPO,
originally derived from sparse sentence rewards, surprisingly provides us with
a token-wise characterization of response quality, which is seamlessly
incorporated into our subsequent PPO training stage. Extensive real-world
alignment experiments verify the effectiveness of the proposed approach.
更多查看译文
AI 理解论文
溯源树
样例
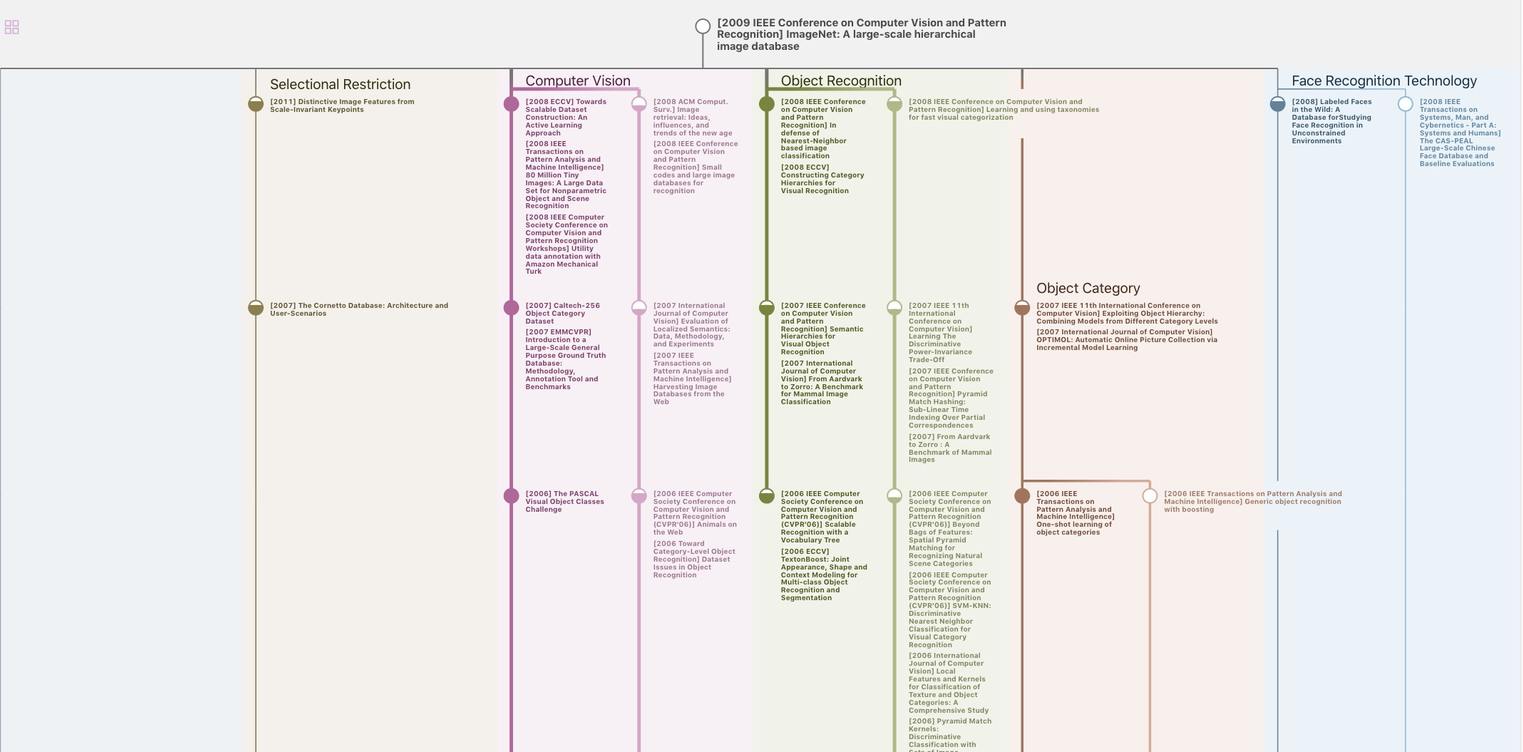
生成溯源树,研究论文发展脉络
Chat Paper
正在生成论文摘要