Learning Efficiency Maximization for Wireless Federated Learning With Heterogeneous Data and Clients
IEEE Transactions on Cognitive Communications and Networking(2024)
摘要
Federated learning is a promising distributed learning paradigm for protecting data privacy by delegating learning tasks to local clients and aggregating local models, instead of raw data, to a server. However, heterogeneous data and clients slow down learning performance and cause significant communication overheads, which hinder the application of federated learning to wireless networks. To address this issue, in this paper, we develop a novel federated learning framework with contribution-aware client participation and batch size selection to maximize learning efficiency in each round, which is equivalent to achieving a global optimal model using minimum time. We first analyze the impact of the client contribution-aware participation on the convergence rate. Then a learning efficiency maximization problem is formulated by jointly optimizing the contribution threshold and the data batch size in each round. Due to the fractional structure of the objective function whose Hessian matrix is not positive semidefinite, the formulated problem is non-convex. We propose a two-layer iterative algorithm to optimally solve this non-convex problem. The effectiveness of the proposed scheme is evaluated using public datasets by comparing it with conventional benchmark schemes. Experimental results show that the proposed scheme achieves improvements in learning efficiency by up to 19.11% on the MNIST dataset and 13.64% on the CIFAR-10 dataset, respectively, compared to benchmark schemes. These results demonstrate that the proposed scheme can effectively mitigate the influence of data and clients heterogeneity for learning efficiency maximization compared to benchmark schemes.
更多查看译文
关键词
Wireless federated learning,client contribution,learning efficiency
AI 理解论文
溯源树
样例
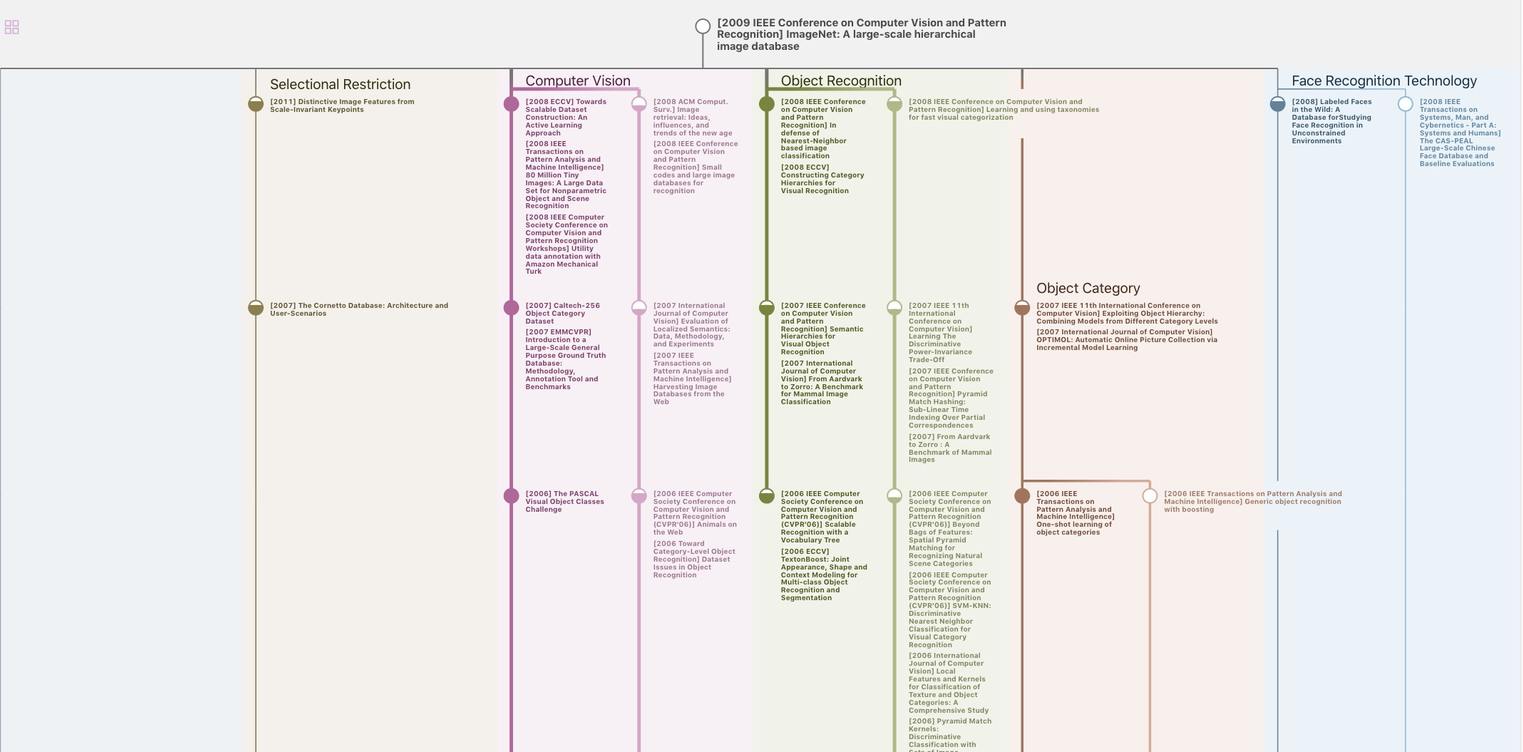
生成溯源树,研究论文发展脉络
Chat Paper
正在生成论文摘要