AI and machine learning in medical imaging: key points from development to translation
BJR|Artificial Intelligence(2024)
摘要
Abstract Innovation in medical imaging artificial intelligence (AI)/machine learning (ML) demands extensive data collection, algorithmic advancements, and rigorous performance assessments encompassing aspects such as generalizability, uncertainty, bias, fairness, trustworthiness, and interpretability. Achieving widespread integration of AI/ML algorithms into diverse clinical tasks will demand a steadfast commitment to overcoming issues in model design, development, and performance assessment. The complexities of AI/ML clinical translation present substantial challenges, requiring engagement with relevant stakeholders, assessment of cost-effectiveness for user and patient benefit, timely dissemination of information relevant to robust functioning throughout the AI/ML lifecycle, consideration of regulatory compliance, and feedback loops for real-world performance evidence. This commentary addresses several hurdles for the development and adoption of AI/ML technologies in medical imaging. Comprehensive attention to these underlying and often subtle factors is critical not only for tackling the challenges but also for exploring novel opportunities for the advancement of AI in radiology.
更多查看译文
AI 理解论文
溯源树
样例
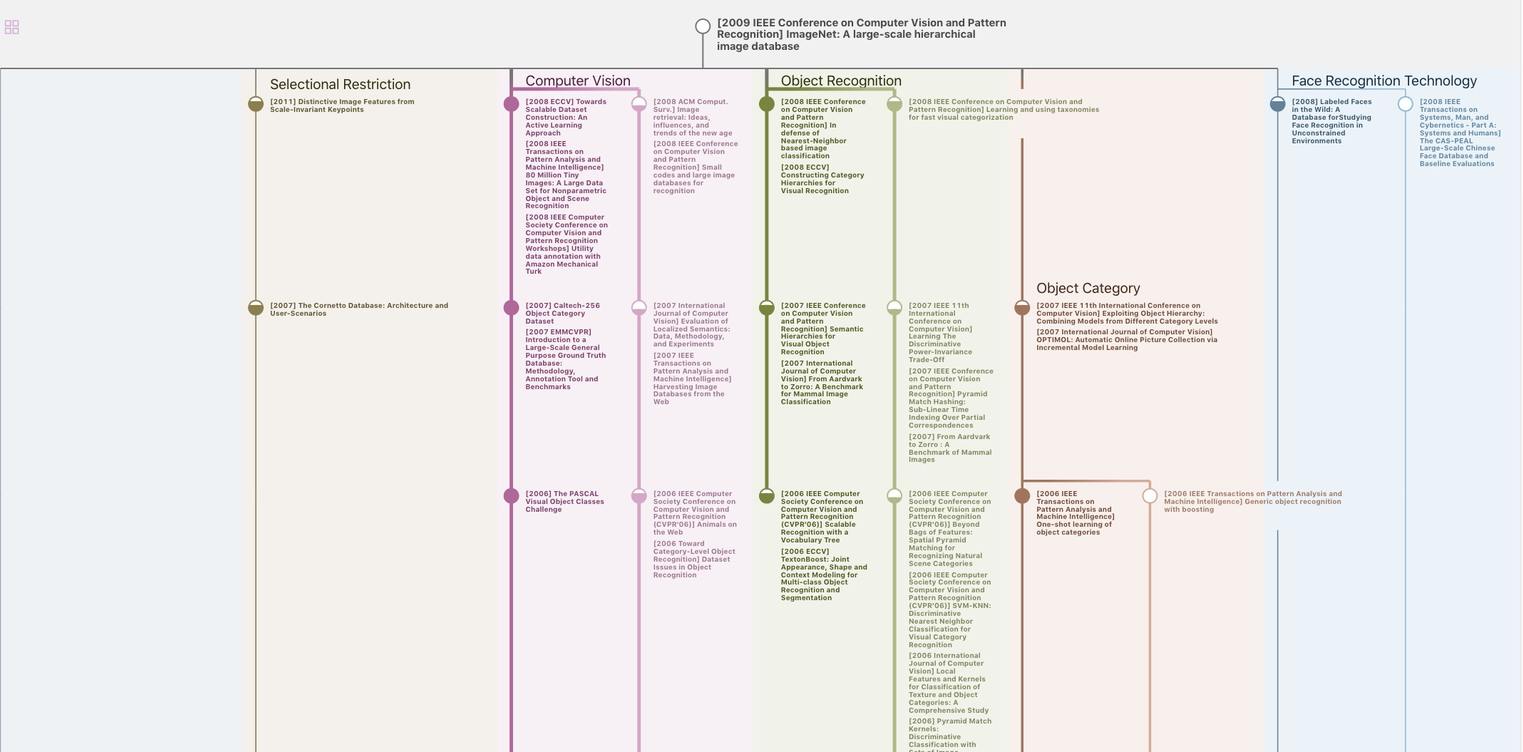
生成溯源树,研究论文发展脉络
Chat Paper
正在生成论文摘要