Learning Abstraction Selection for Bayesian Program Analysis
Proceedings of the ACM on Programming Languages(2024)
摘要
We propose a learning-based approach to select abstractions for Bayesian program analysis. Bayesian program analysis converts a program analysis into a Bayesian model by attaching probabilities to analysis rules. It computes probabilities of analysis results and can update them by learning from user feedback, test runs, and other information. Its abstraction heavily affects how well it learns from such information. There exists a long line of works in selecting abstractions for conventional program analysis but they are not effective for Bayesian program analysis. This is because they do not optimize for generalization ability. We propose a data-driven framework to solve this problem by learning from labeled programs. Starting from an abstraction, it decides how to change the abstraction based on analysis derivations. To be general, it considers graph properties of analysis derivations; to be effective, it considers the derivations before and after changing the abstraction. We demonstrate the effectiveness of our approach using a datarace analysis and a thread-escape analysis.
更多查看译文
关键词
Bayesian network,Static analysis,abstract interpretation,alarm ranking,machine learning for program analysis
AI 理解论文
溯源树
样例
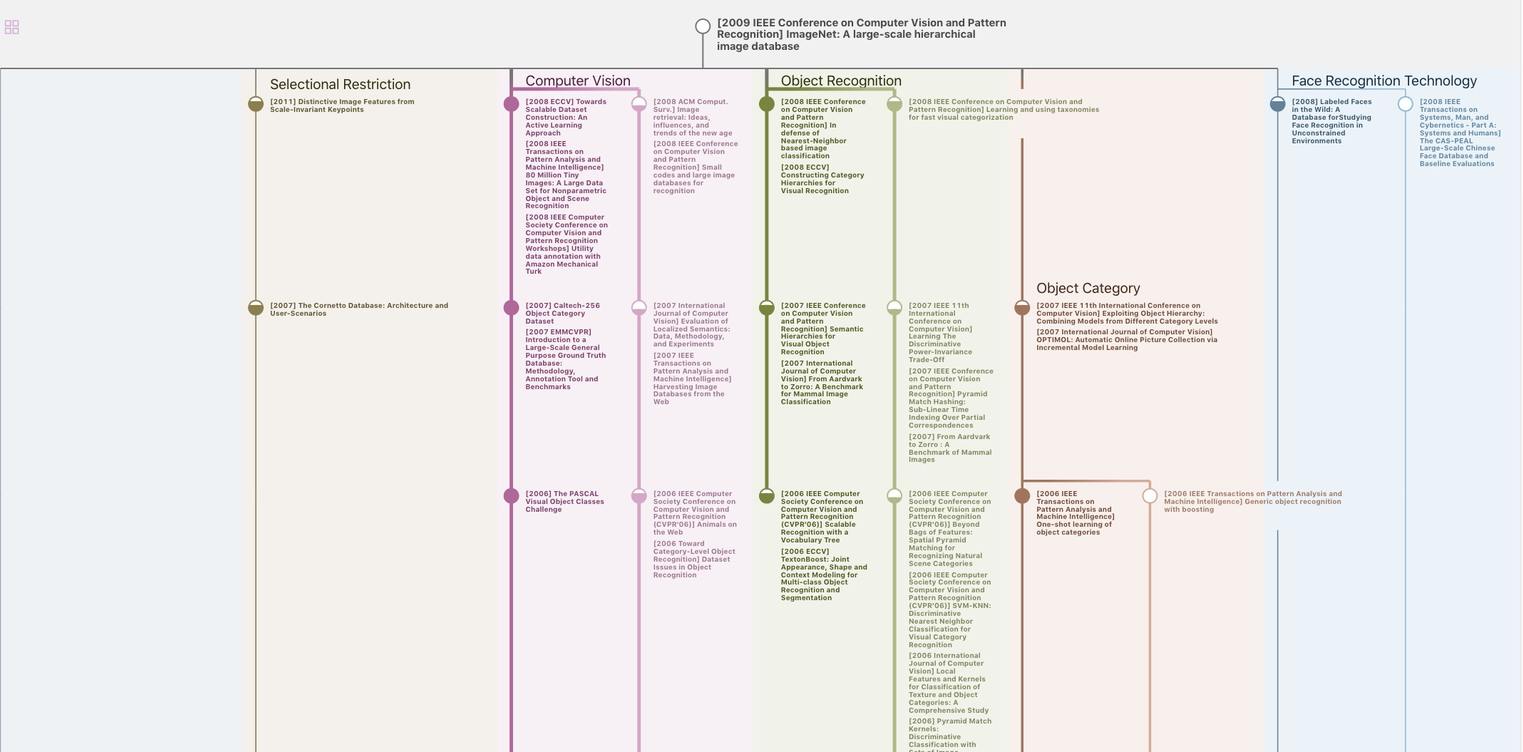
生成溯源树,研究论文发展脉络
Chat Paper
正在生成论文摘要