ADPS: Asymmetric Distillation Postsegmentation for Image Anomaly Detection.
IEEE transactions on neural networks and learning systems(2024)
摘要
Knowledge distillation-based anomaly detection (KDAD) methods rely on the teacher-student paradigm to detect and segment anomalous regions by contrasting the unique features extracted by both networks. However, existing KDAD methods suffer from two main limitations: 1) the student network can effortlessly replicate the teacher network's representations and 2) the features of the teacher network serve solely as a "reference standard" and are not fully leveraged. Toward this end, we depart from the established paradigm and instead propose an innovative approach called asymmetric distillation postsegmentation (ADPS). Our ADPS employs an asymmetric distillation paradigm that takes distinct forms of the same image as the input of the teacher-student networks, driving the student network to learn discriminating representations for anomalous regions. Meanwhile, a customized Weight Mask Block (WMB) is proposed to generate a coarse anomaly localization mask that transfers the distilled knowledge acquired from the asymmetric paradigm to the teacher network. Equipped with WMB, the proposed postsegmentation module (PSM) can effectively detect and segment abnormal regions with fine structures and clear boundaries. Experimental results demonstrate that the proposed ADPS outperforms the state-of-the-art methods in detecting and segmenting anomalies. Surprisingly, ADPS significantly improves average precision (AP) metric by 9% and 20% on the MVTec anomaly detection (AD) and KolektorSDD2 datasets, respectively.
更多查看译文
关键词
Anomaly detection (AD),asymmetric distillation,postsegmentation module (PSM),weight mask block (WMB)
AI 理解论文
溯源树
样例
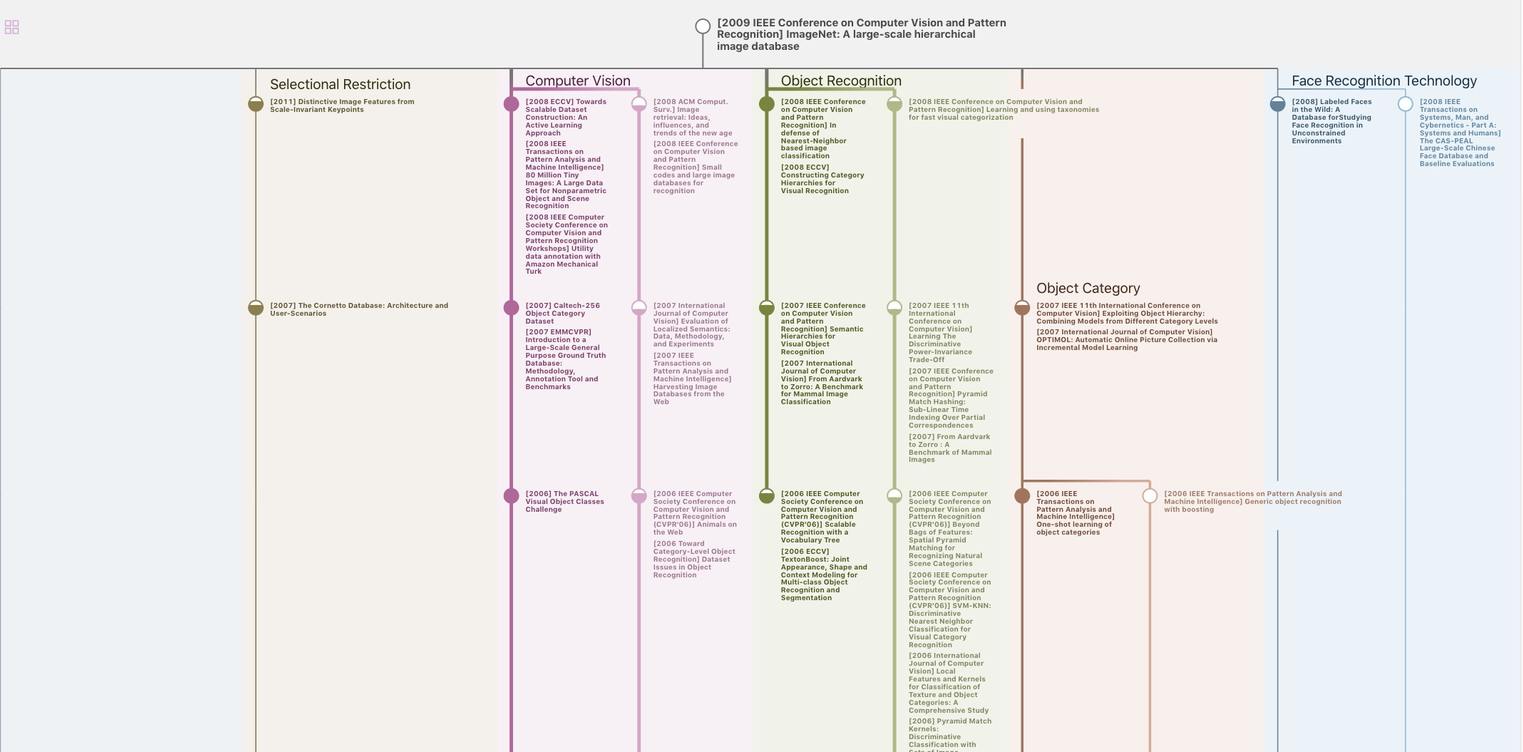
生成溯源树,研究论文发展脉络
Chat Paper
正在生成论文摘要