Learning to Improve Out-of-Distribution Generalization via Self-adaptive Language Masking
IEEE/ACM Transactions on Audio, Speech, and Language Processing(2024)
摘要
Although the pre-trained Transformers learned general linguistic knowledge from large-scale corpus, they still overfit on the lexical biases when fine-tuning on specific datasets. This problem limits the generalizability of pre-trained models, particularly when learning over out-of-distribution (OOD) data. To address this issue, this paper proposes a self-adaptive language masking (AdaLMask) paradigm to fine-tune the pre-trained Transformers. AdaLMask obviates lexical biases by eliminating the dependence on semantically inessential words. Specifically, AdaLMask learns a Gumbel-Softmax distribution to determine the desired masking positions, and the distribution parameters are optimized via a representation-invariant (RInv) objective to ensure the masked positions are semantically lossless. Four natural language processing tasks are chosen to evaluate the effectiveness of the proposed method on the robustness of lexical biases and OOD generalization. All empirical results demonstrate that the AdaLMask paradigm substantially improves the OOD generalization of pre-trained Transformers.
更多查看译文
关键词
Pre-trained Transformers,Lexical Bias,Generalizability,Out-of-Distribution,Self-Adaptive Language Masking,Representation Invariant
AI 理解论文
溯源树
样例
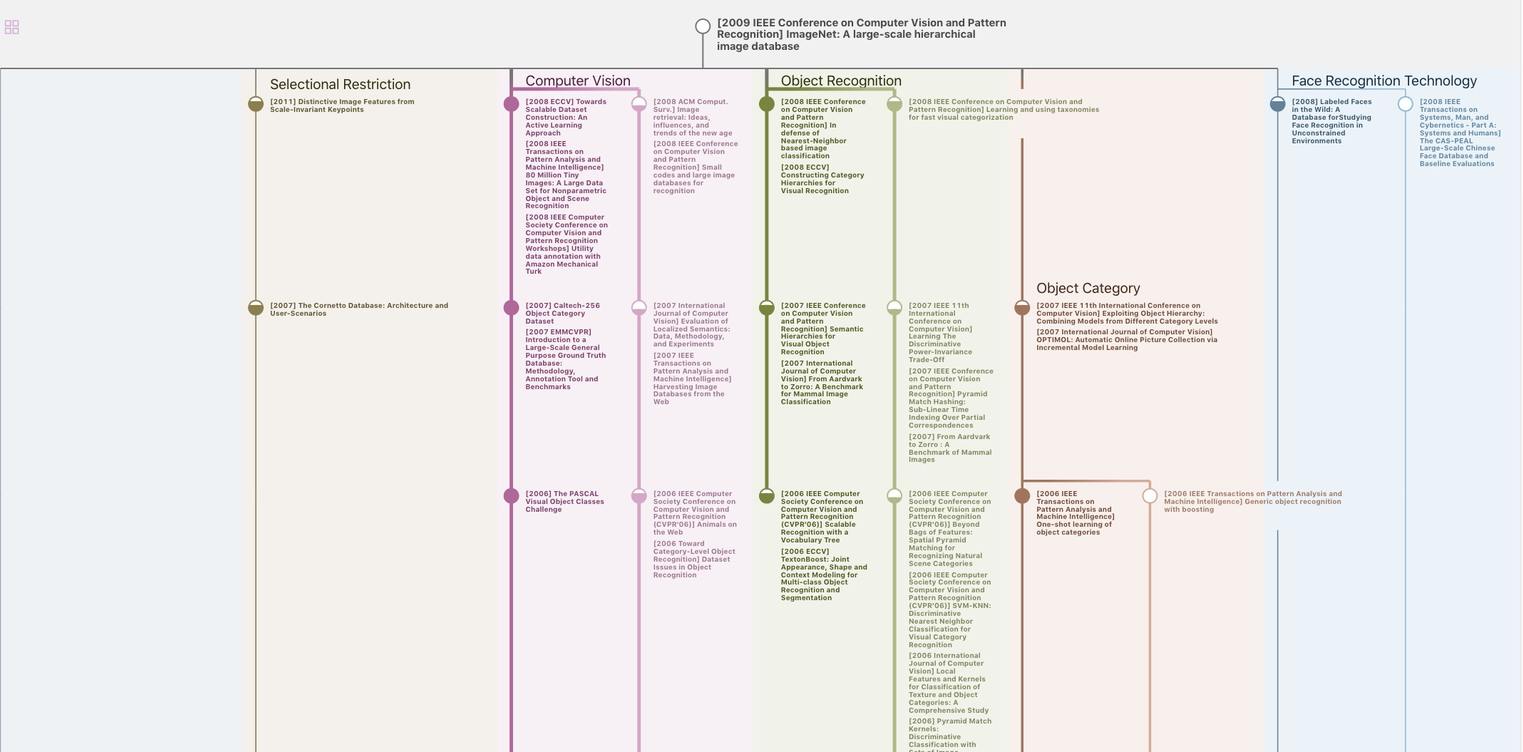
生成溯源树,研究论文发展脉络
Chat Paper
正在生成论文摘要