Toward Efficient and Secure Object Detection With Sparse Federated Training Over Internet of Vehicles
IEEE Transactions on Intelligent Transportation Systems(2024)
摘要
Internet of Vehicles (IoV) plays a vital role in alleviating traffic issues. Object detection is one of the key technologies in IoV, which has been widely used to provide traffic management services by analyzing timely and sensitive vehicle-related information. However, the current object detection methods mostly rely on centralized deep training, that is, the sensitive data obtained by edge devices needs to be uploaded to the server, which raises latency and privacy issues. To tackle these issues, we propose to accomplish object detection through sparse federated training with dynamic model aggregation, namely FedWeg, to reduce the communication cost and privacy leakage induced by data transmission. Specifically, FedWeg performs sparse training in edge devices and uploads the lightweight models to the server. To reduce the unnecessary transmission overhead, we propose a dynamic sparsity adjustment scheme that gradually increases the sparsity ratios. Then, we propose to utilize the inverse ratio of sparsity ratios from different edge devices to calculate aggregate weights to diminish the negative impact of sparse training on learning performance. Moreover, we theoretically analyze the convergence rate of FedWeg, which reveals that the impact of network sparsity on model performance, and higher average sparsity rates result in greater errors. Finally, we conduct extensive experiments on four real-life datasets using YOLOv3 and VGG-16. The results show that our FedWeg algorithm outperforms baselines in terms of communication costs and test accuracy.
更多查看译文
AI 理解论文
溯源树
样例
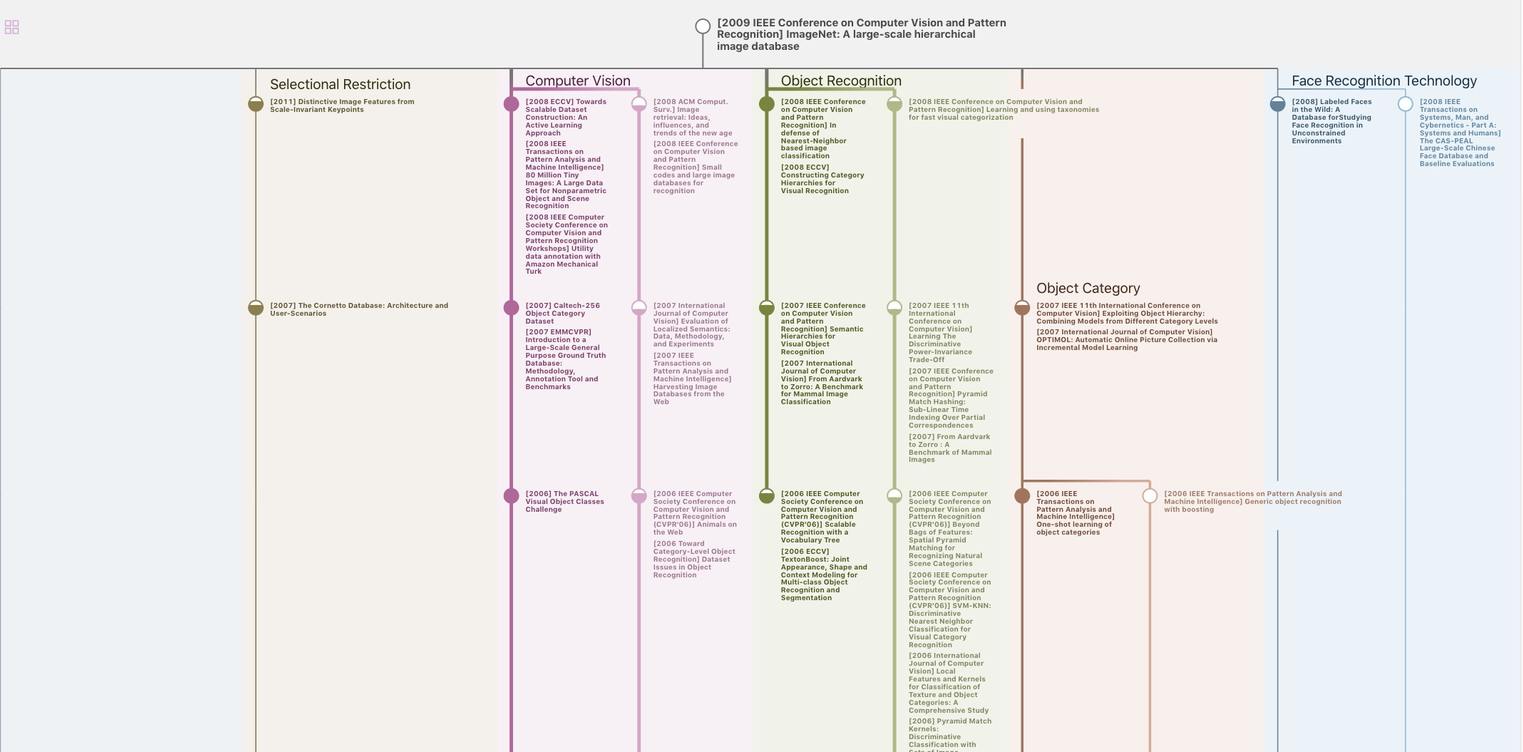
生成溯源树,研究论文发展脉络
Chat Paper
正在生成论文摘要