Computer-aided diagnostic system with automated deep learning method based on the AutoGluon framework improved the diagnostic accuracy of early esophageal cancer.
Journal of gastrointestinal oncology(2024)
摘要
Background:There have been studies on the application of computer-aided diagnosis (CAD) in the endoscopic diagnosis of early esophageal cancer (EEC), but there is still a significant gap from clinical application. We developed an endoscopic CAD system for EEC based on the AutoGluon framework, aiming to explore the feasibility of automatic deep learning (DL) in clinical application.
Methods:The endoscopic pictures of normal esophagus, esophagitis, and EEC were collected from The First Affiliated Hospital of Soochow University (September 2015 to December 2021) and the Norwegian HyperKvasir database. All images of non-cancerous esophageal lesions and EEC in this study were pathologically examined. There were three tasks: task A was normal vs. lesion classification under non-magnifying endoscopy (n=932 vs. 1,092); task B was non-cancer lesion vs. EEC classification under non-magnifying endoscopy (n=594 vs. 429); and task C was non-cancer lesion vs. EEC classification under magnifying endoscopy (n=505 vs. 824). In all classification tasks, we took 100 pictures as the verification set, and the rest comprised as the training set. The CAD system was established based on the AutoGluon framework. Diagnostic performance of the model was compared with that of endoscopists grouped according to years of experience (senior >15 years; junior <5 years). Model evaluation indicators included accuracy, recall rate, precision, F1 value, interpretation time, and the area under the receiver operating characteristic (ROC) curve (AUC).
Results:In tasks A and B, the accuracies of medium-performance CAD and high-performance CAD were lower than those of junior doctors and senior doctors. In task C, the medium-performance and high-performance CAD accuracies were close to those of junior doctors and senior doctors. The high-performance CAD model outperformed the junior doctors in both task A (0.850 vs. 0.830) and task C (0.840 vs. 0.830) in sensitivity comparison, but there was still a large gap between high-performance CAD models and doctors in sensitivity comparison. In task A, with the aid of CAD pre-interpretation, the accuracy of junior and senior physicians were significantly improved (from 0.880 to 0.915 and from 0.920 to 0.945, respectively); the time spent on film reading was significantly shortened (junior: from 11.3 to 8.7 s; senior: from 6.7 to 5.5 s). In task C, with the aid of CAD pre-interpretation, the accuracy of junior and senior physicians were significantly improved (from 0.850 to 0.865 and from 0.915 to 0.935, respectively); the reading time was significantly shortened (junior: from 9.5 to 7.7 s; senior: from 5.6 to 3.0 s).
Conclusions:The CAD system based on the AutoGluon framework can assist doctors to improve the diagnostic accuracy and reading time of EEC under endoscopy. This study reveals that automatic DL methods are promising in clinical application.
更多查看译文
AI 理解论文
溯源树
样例
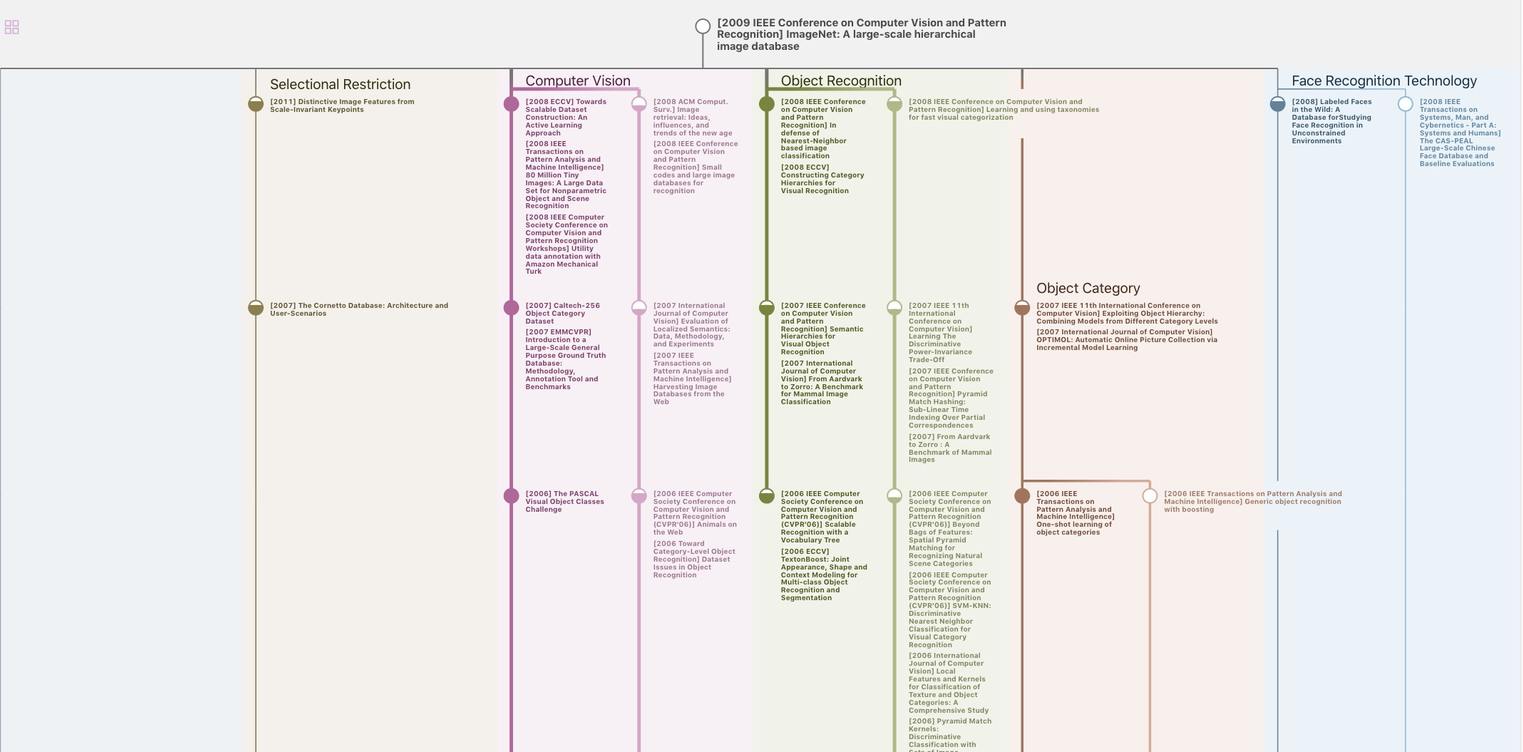
生成溯源树,研究论文发展脉络
Chat Paper
正在生成论文摘要