Deep learning for prediction of energy consumption: an applied use case in an office building
Applied Intelligence(2024)
摘要
Non-residential buildings are responsible for more than a third of global energy consumption. Estimating building energy consumption is the first step towards identifying inefficiencies and optimizing energy management policies. This paper presents a study of Deep Learning techniques for time series analysis applied to building energy prediction with real environments. We collected multisource sensor data from an actual office building under normal operating conditions, pre-processed them, and performed a comprehensive evaluation of the accuracy of feed-forward and recurrent neural networks to predict energy consumption. The results show that memory-based architectures (LSTMs) perform better than stateless ones (MLPs) even without data aggregation (CNNs), although the lack of ample usable data in this type of problem avoids making the most of recent techniques such as sequence-to-sequence (Seq2Seq).
更多查看译文
关键词
Buildings,Energy consumption forecasting,Time series,Deep learning,XGBoost,Multilayer perceptron,Recurrent neural networks,Convolutional neural networks,Sequence to sequence networks
AI 理解论文
溯源树
样例
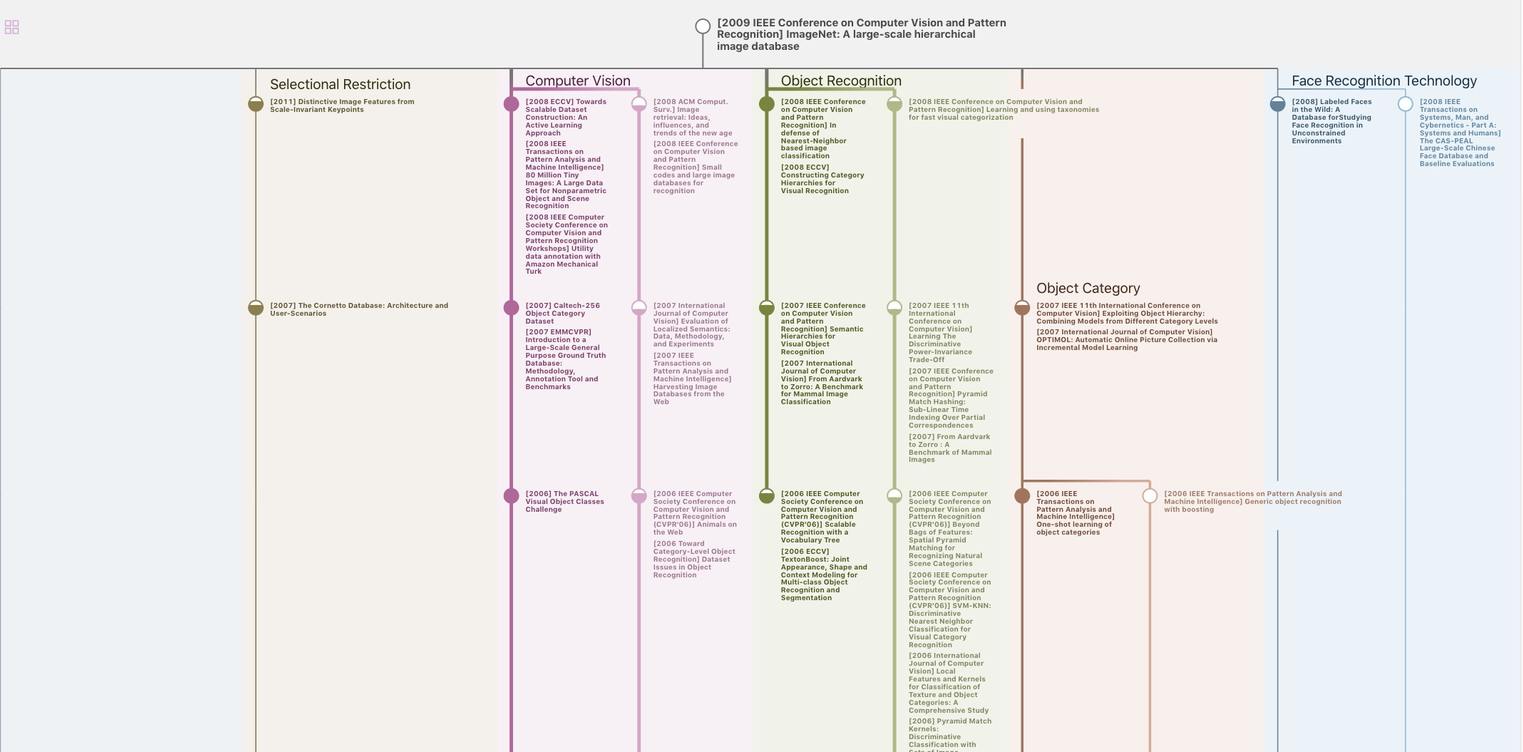
生成溯源树,研究论文发展脉络
Chat Paper
正在生成论文摘要