Timing Matters: A Machine Learning Method for the Prioritization of Drug–Drug Interactions Through Signal Detection in the FDA Adverse Event Reporting System and Their Relationship with Time of Co-exposure
Drug Safety(2024)
摘要
Current drug–drug interaction (DDI) detection methods often miss the aspect of temporal plausibility, leading to false-positive disproportionality signals in spontaneous reporting system (SRS) databases. This study aims to develop a method for detecting and prioritizing temporally plausible disproportionality signals of DDIs in SRS databases by incorporating co-exposure time in disproportionality analysis. The method was tested in the Food and Drug Administration (FDA) Adverse Event Reporting System (FAERS). The CRESCENDDI dataset of positive controls served as the primary source of true-positive DDIs. Disproportionality analysis was performed considering the time of co-exposure. Temporal plausibility was assessed using the flex point of cumulative reporting of disproportionality signals. Potential confounders were identified using a machine learning method (i.e. Lasso regression). Disproportionality analysis was conducted on 122 triplets with more than three cases, resulting in the prioritization of 61 disproportionality signals (50.0
更多查看译文
AI 理解论文
溯源树
样例
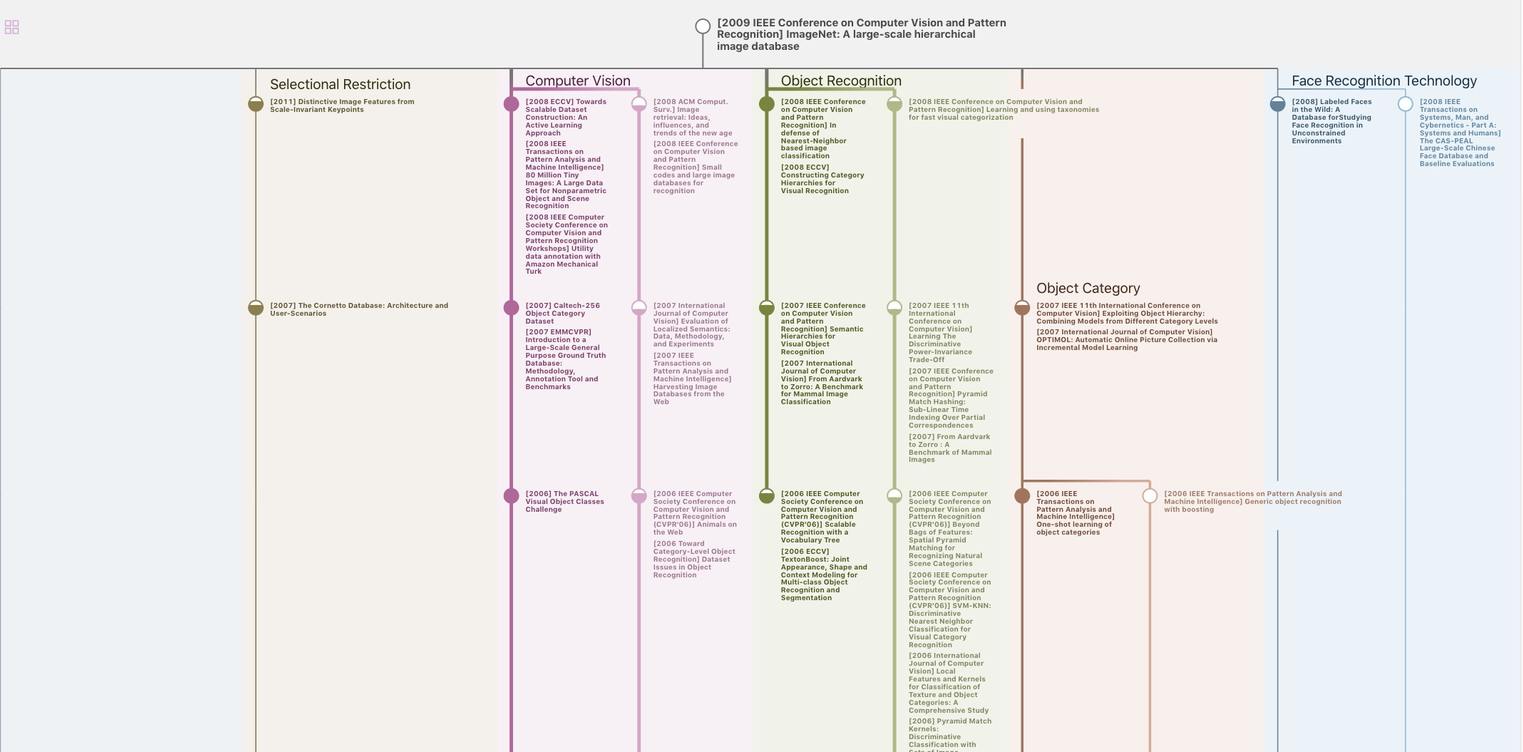
生成溯源树,研究论文发展脉络
Chat Paper
正在生成论文摘要