Revealing the working mechanism of quantum neural networks by mutual information
arxiv(2024)
摘要
Quantum neural networks (QNNs) is a parameterized quantum circuit model,
which can be trained by gradient-based optimizer, can be used for supervised
learning, regression tasks, combinatorial optimization, etc. Although many
works have demonstrated that QNNs have better learnability, generalizability,
etc. compared to classical neural networks. However, as with classical neural
networks, we still can't explain their working mechanism well. In this paper,
we reveal the training mechanism of QNNs by mutual information. Unlike
traditional mutual information in neural networks, due to quantum computing
remains information conserved, the mutual information is trivial of the input
and output of U operator. In our work, in order to observe the change of mutual
information during training, we divide the quantum circuit (U operator) into
two subsystems, discard subsystem (D) and measurement subsystem (M)
respectively. We calculate two mutual information, I(Di : Mo) and I(Mi : Mo) (i
and o means input or output of the corresponding subsystem), and observe their
behavior during training. As the epochs increases, I(Di : Mo) gradually
increases, this may means some information of discard subsystem is continuously
pushed into the measurement subsystem, the information should be label-related.
What's more, I(Mi : Mo) exist two-phase behavior in training process, this
consistent with the information bottleneck anticipation. The first phase, I(Mi
: Mo) is increasing, this means the measurement subsystem perform feature
fitting. The second phase, I(Mi : Mo) is decreasing, this may means the system
is generalizing, the measurement subsystem discard label-irrelevant information
into the discard subsystem as many as possible. Our work discussed the working
mechanism of QNNs by mutual information, further, it can be used to analyze the
accuracy and generalization of QNNs.
更多查看译文
AI 理解论文
溯源树
样例
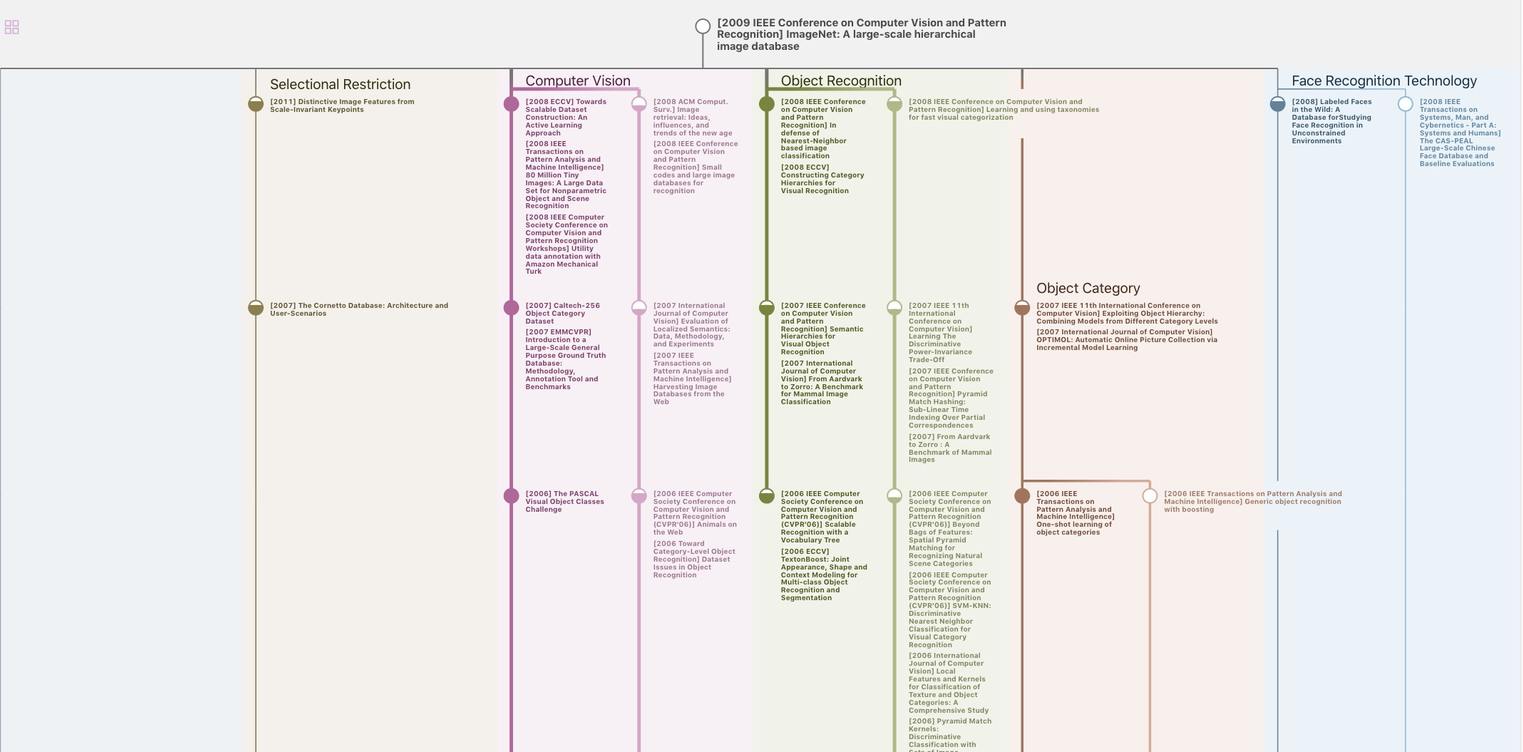
生成溯源树,研究论文发展脉络
Chat Paper
正在生成论文摘要