Fusing Depthwise and Pointwise Convolutions for Efficient Inference on GPUs
arxiv(2024)
摘要
Depthwise and pointwise convolutions have fewer parameters and perform fewer
operations than standard convolutions. As a result, they have become
increasingly used in various compact DNNs, including convolutional neural
networks (CNNs) and vision transformers (ViTs). However, they have a lower
compute-to-memory-access ratio than standard convolutions, making their memory
accesses often the performance bottleneck. This paper explores fusing depthwise
and pointwise convolutions to overcome the memory access bottleneck. The focus
is on fusing these operators on GPUs. The prior art on GPU-based fusion suffers
from one or more of the following: (1) fusing either a convolution with an
element-wise or multiple non-convolutional operators, (2) not explicitly
optimizing for memory accesses, (3) not supporting depthwise convolutions. This
paper proposes Fused Convolutional Modules (FCMs), a set of novel fused
depthwise and pointwise GPU kernels. FCMs significantly reduce pointwise and
depthwise convolutions memory accesses, improving execution time and energy
efficiency. To evaluate the trade-offs associated with fusion and determine
which convolutions are beneficial to fuse and the optimal FCM parameters, we
propose FusePlanner. FusePlanner consists of cost models to estimate the memory
accesses of depthwise, pointwise, and FCM kernels given GPU characteristics.
Our experiments on three GPUs using representative CNNs and ViTs demonstrate
that FCMs save up to 83
3.7x compared to cuDNN. Complete model implementations of various CNNs using
our modules outperform TVMs' achieving speedups of up to 1.8x and saving up to
two-thirds of the energy.
更多查看译文
AI 理解论文
溯源树
样例
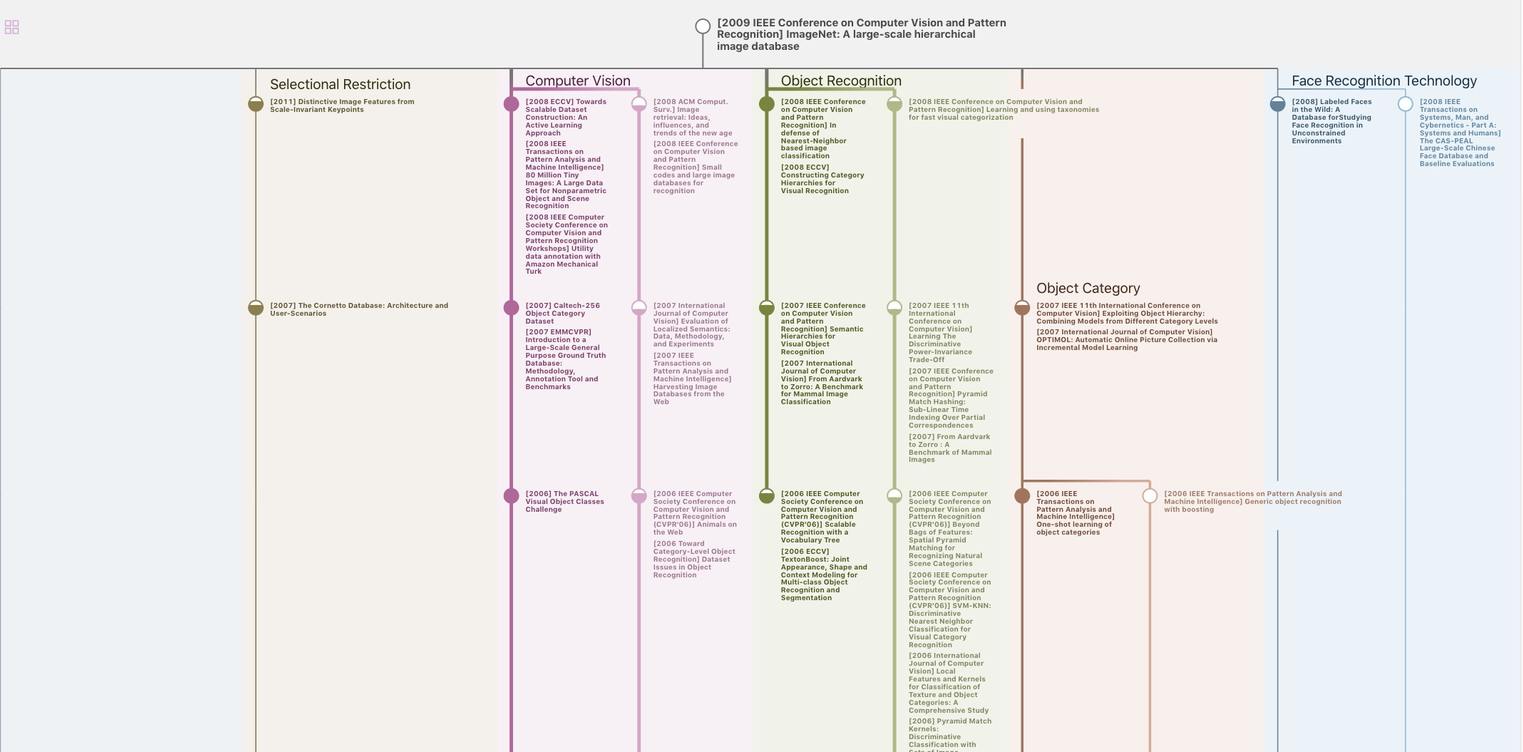
生成溯源树,研究论文发展脉络
Chat Paper
正在生成论文摘要