Generating Robust Counterfactual Witnesses for Graph Neural Networks
arxiv(2024)
摘要
This paper introduces a new class of explanation structures, called robust
counterfactual witnesses (RCWs), to provide robust, both counterfactual and
factual explanations for graph neural networks. Given a graph neural network M,
a robust counterfactual witness refers to the fraction of a graph G that are
counterfactual and factual explanation of the results of M over G, but also
remains so for any "disturbed" G by flipping up to k of its node pairs. We
establish the hardness results, from tractable results to co-NP-hardness, for
verifying and generating robust counterfactual witnesses. We study such
structures for GNN-based node classification, and present efficient algorithms
to verify and generate RCWs. We also provide a parallel algorithm to verify and
generate RCWs for large graphs with scalability guarantees. We experimentally
verify our explanation generation process for benchmark datasets, and showcase
their applications.
更多查看译文
AI 理解论文
溯源树
样例
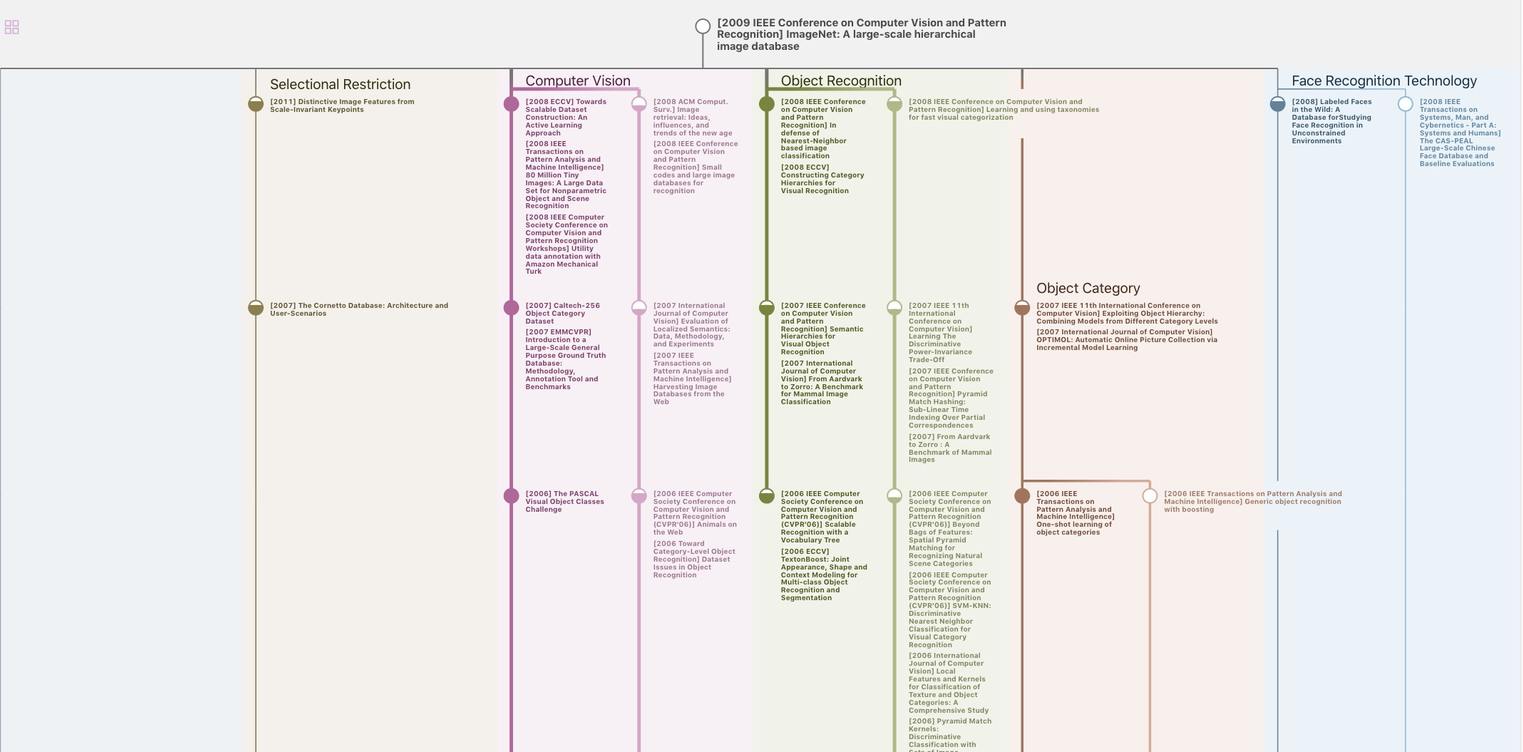
生成溯源树,研究论文发展脉络
Chat Paper
正在生成论文摘要