Fast and practical intrusion detection system based on federated learning for VANET
Computers & Security(2024)
摘要
VANET facilitates communication between vehicles and roadside units, thereby enhancing traffic safety and efficiency. However, it also introduces several attack vectors, compromising security and privacy. To enhance the practicality and precision of existing VANET intrusion detection systems, we propose a novel hybrid algorithm named VAN-IDS. This algorithm combines packet analysis and physical feature analysis. Temporal properties of packets are analyzed using Bi-LSTM, while physical attributes of vehicles are evaluated using LightGBM. Predictive outputs from both models are merged using the Dempster-Shafer theory. Data is collected from the F2MD simulation environment, and experiments are conducted to evaluate the efficacy of VAN-IDS. Results show that VAN-IDS achieves an accuracy exceeding 98.5% in detecting DoS and Sybil attacks, surpassing existing VANET IDSs and meeting real-time detection requirements. Federated learning is introduced, with VAN-FED-IDS designed to accelerate model training and updates, while protecting the privacy of the model and dataset. Roadside units (RSUs) serve as edge computing nodes for local model training, while cloud services aggregate models for collaborative training of detection algorithms. In these experiments, the open-source federated learning frameworks Flower and FedTree are used to assess communication overhead and training duration in both single-machine simulation and multi-machine deployment scenarios. This demonstrates the viability of federated learning, which can protect local data privacy, expedite model training and updates within the IDS, and maintain the original model’s detection accuracy.
更多查看译文
关键词
VANET,Intrusion detection,Machine learning,DST,Federated learning
AI 理解论文
溯源树
样例
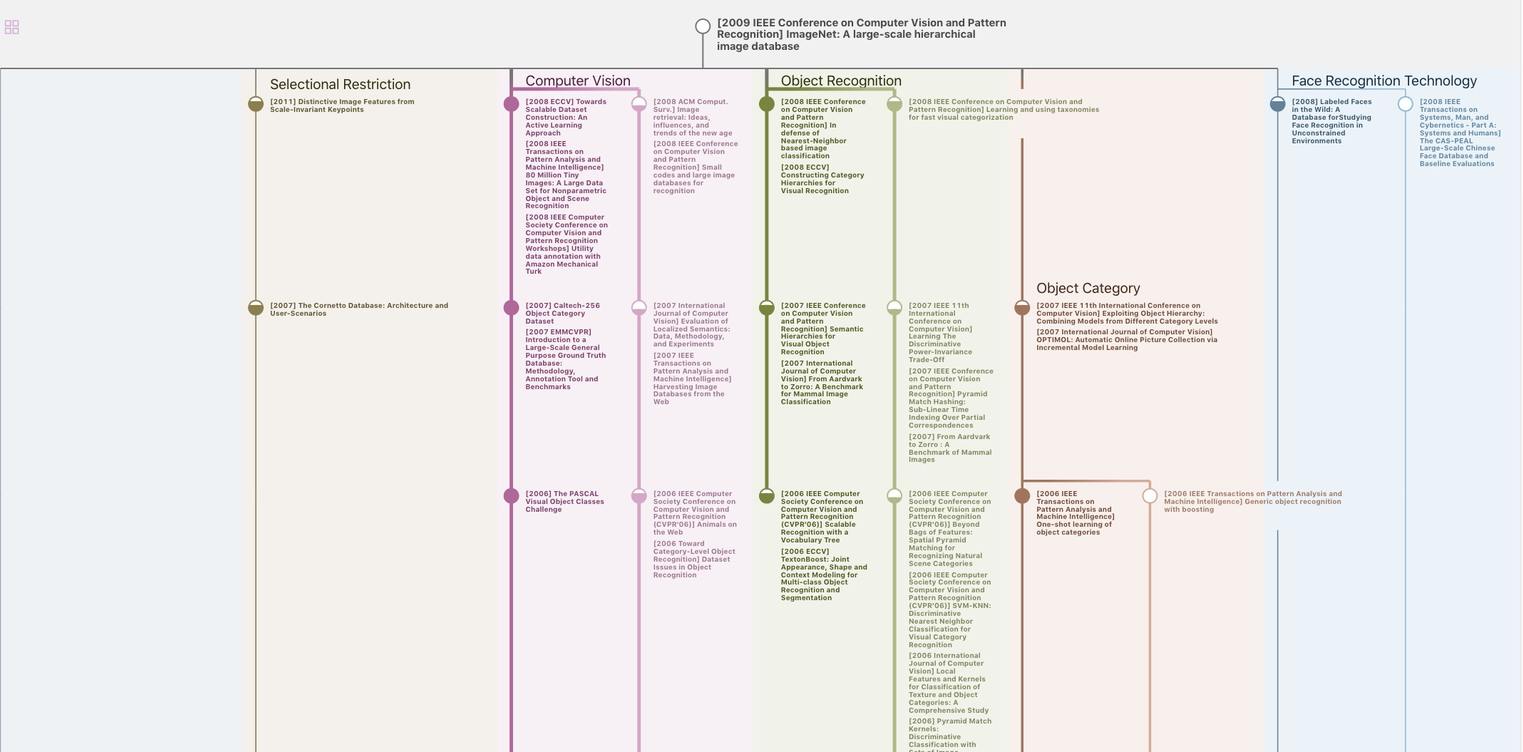
生成溯源树,研究论文发展脉络
Chat Paper
正在生成论文摘要