Nonlinear Slow-varying Dynamics-assisted Temporal Graph Transformer Network for Remaining Useful Life Prediction
Reliability Engineering & System Safety(2024)
摘要
Remaining useful life (RUL) plays an important role in the prognostics and health management of mechanical systems. Recently, deep learning-based methods have been widely applied in the field of RUL prediction. However, there still suffer from two limitations. One is that the existing RUL prediction methods cannot capture spatial dependencies and long-term temporal dependencies. The other is that nonlinear slow-varying dynamics related to the degradation behavior have not been explored in the RUL prediction. To break these limitations, a nonlinear slow-varying dynamics-assisted temporal graph Transformer network (NSD-TGTN) is proposed in this paper for RUL prediction. NSD-TGTN can simultaneously capture and model spatiotemporal graphs and nonlinear slow-varying dynamics to achieve RUL prediction. Herein, the TGTN is developed to mine both spatial and long-term temporal dependencies for constructing the spatiotemporal features. And, nonlinear slow-varying features are built and introduced into the TGTN to enhance the RUL prediction capacity. Two datasets are utilized to validate the effectiveness and superiority of the proposed method. Compared with existing advanced methods, the average prediction accuracies of the NSD-TGTN on the C-MAPSS dataset and the wear dataset are improved by 1.70% and 8.22%, respectively.
更多查看译文
关键词
Spatiotemporal graphs,RUL prediction,Transformer network,Attention mechanism,Nonlinear slow-varying dynamics
AI 理解论文
溯源树
样例
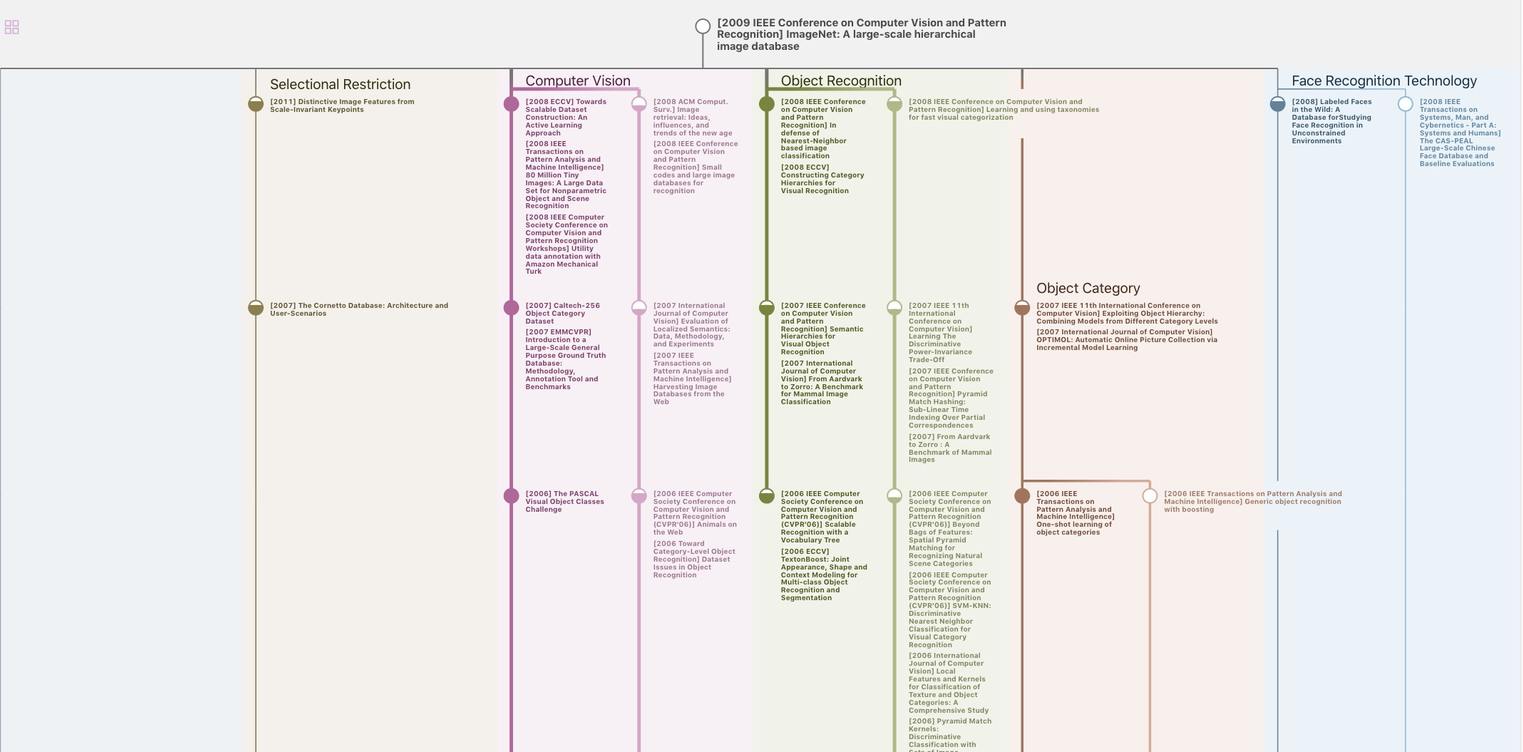
生成溯源树,研究论文发展脉络
Chat Paper
正在生成论文摘要