Transfer learning-assisted computational screening of metal-organic frameworks and covalent-organic frameworks for the separation of Xe/Kr noble gas
Separation and Purification Technology(2024)
摘要
The adsorption separation of xenon/krypton (Xe/Kr) by porous materials has gained significant attention in industrial development. Metal-organic frameworks (MOFs) and covalent-organic frameworks (COFs) featuring permanent porosity, ultra-high surface area, and tunable pore size enable efficient separation of Xe/Kr, which promising structures can be identified by high-throughput computational screening and machine learning. However, the asymmetric development of the structure database between MOFs and other porous materials (including COFs) led to a data imbalance that challenged screening and machine learning for other porous materials, especially for computation-ready experimental (CoRE) databases. Transfer learning (TL) can address this issue by extracting the information from MOFs to improve generalization for other materials. In this work, geometric, energy, and chemical-based descriptors were obtained from MOFs and COFs to train TL models. It was demonstrated that TL with only 300 data sets of hypothetical COFs being used can produce satisfactory accuracy (R2 > 0.85) by predicting CoRE COFs based on CoRE MOFs. Furthermore, we conducted a feature importance analysis that suggested the energy descriptor plays a dominant role in predicting the Xe/Kr separation performance of the ensemble algorithm, whereas the chemical descriptor is an important descriptor for the deep neural network. This study provides an alternative transfer learning route to predict the Xe/Kr separation performance of nanoporous materials database with limited structures.
更多查看译文
关键词
Noble gas separation,Metal-organic frameworks,Covalent-organic frameworks,Transfer Learning,High-throughput computational screening
AI 理解论文
溯源树
样例
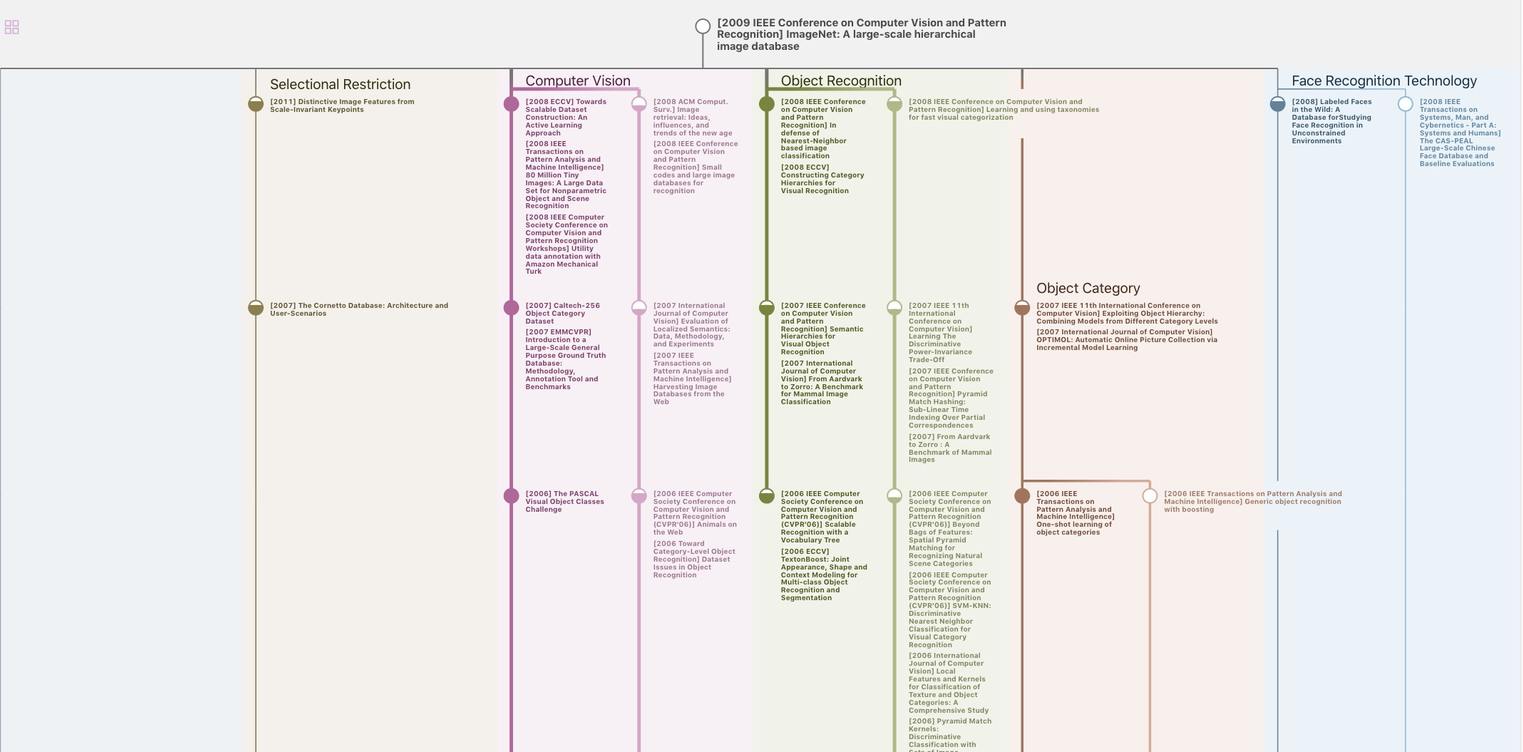
生成溯源树,研究论文发展脉络
Chat Paper
正在生成论文摘要