DNA-T: Deformable Neighborhood Attention Transformer for Irregular Medical Time Series
IEEE Journal of Biomedical and Health Informatics(2024)
摘要
The real-world Electronic Health Records (EHRs) present irregularities due to changes in the patient's health status, resulting in various time intervals between observations and different physiological variables examined at each observation point. There have been recent applications of Transformer-based models in the field of irregular time series. However, the full attention mechanism in Transformer overly focuses on distant information, ignoring the short-term correlations of the condition. Thereby, the model is not able to capture localized changes or short-term fluctuations in patients' conditions. Therefore, we propose a novel end-to-end Deformable Neighborhood Attention Transformer (DNA-T) for irregular medical time series. The DNA-T captures local features by dynamically adjusting the receptive field of attention and aggregating relevant deformable neighborhoods in irregular time series. Specifically, we design a Deformable Neighborhood Attention (DNA) module that enables the network to attend to relevant neighborhoods by drifting the receiving field of neighborhood attention. The DNA enhances the model's sensitivity to local information and representation of local features, thereby capturing the correlation of localized changes in patients' conditions. We conduct extensive experiments to validate the effectiveness of DNA-T, outperforming existing state-of-the-art methods in predicting the mortality risk of patients. Moreover, we visualize an example to validate the effectiveness of the proposed DNA. Our code is available at
https://github.com/Nekumiya-x/DNA-T
.
更多查看译文
关键词
Deformable Neighborhood Attention,Medical Time Series,Transformer
AI 理解论文
溯源树
样例
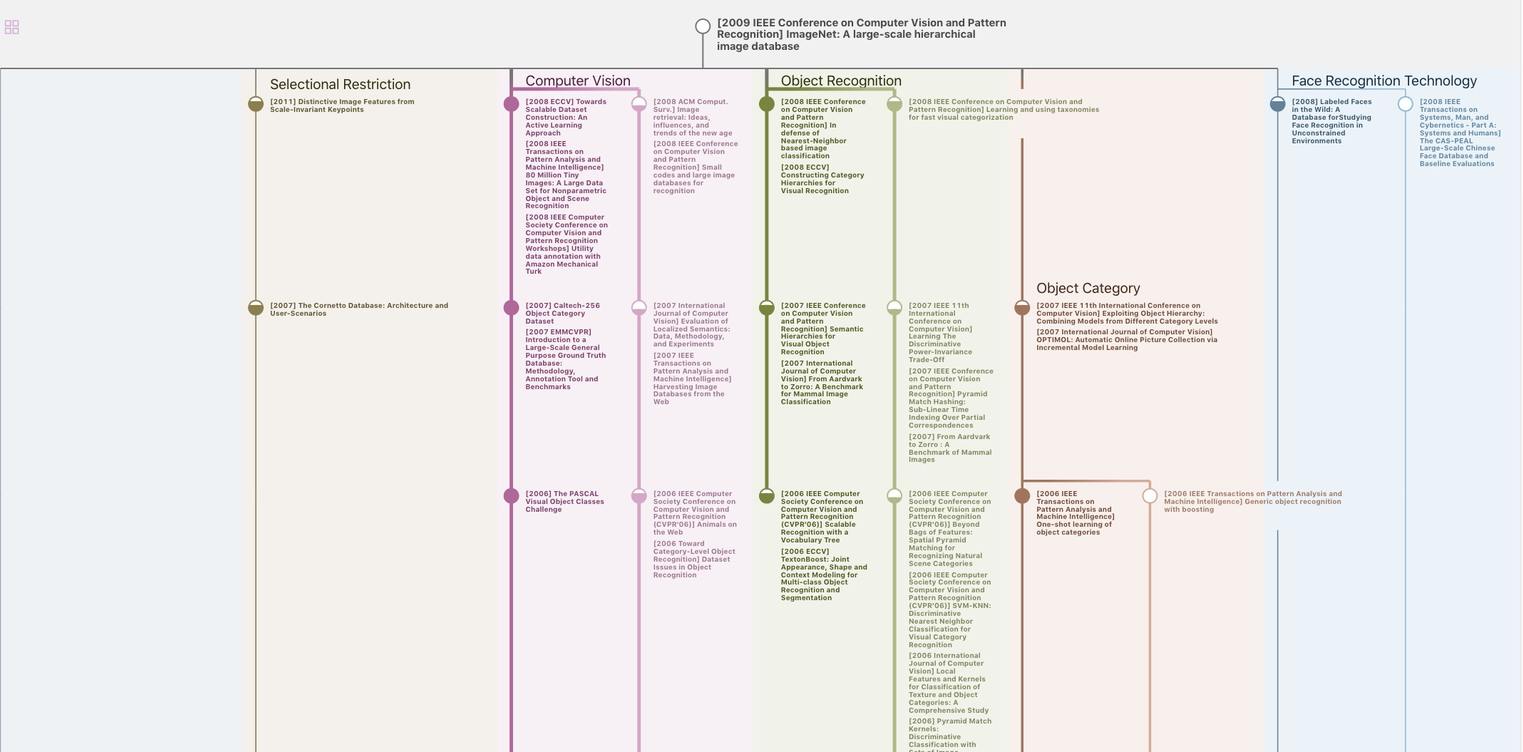
生成溯源树,研究论文发展脉络
Chat Paper
正在生成论文摘要