The Improved Intrinsic Time-Scale Analysis for Multidimensional Signal-to-Noise Feature Separation of Borehole Seismic Data.
IEEE Trans. Geosci. Remote. Sens.(2024)
摘要
Distributed acoustic sensing (DAS) technology has been applied in vertical seismic profiling to provide high-precision seismic records for oil and gas exploration in recent years. However, these records are often contaminated by various noises due to the limitations of acquisition instrument and borehole environment, resulting in an unclear reflection of the geologic structure. The object of this paper is to remove the severe noise contamination through signal decomposition, time-frequency description and feature classification. Firstly, we design an improved decomposition method to avoid signal distortion and reduce frequency aliasing during the decomposition process. A flexible sifting iterative stop condition is applied to sift through unevenly distributed noises in the DAS records. Secondly, we construct a high-dimensional attribute space using time and frequency factors to represent the confused decomposed components. This ascending-dimensional feature mapping facilitates the description of differences between seismic signal and noise. Finally, we establish a two-level ensemble framework based on tree-structure learners to complete the classification tasks in the high-dimensional feature space. Experiments have proved that this method effectively recovers seismic signal waves while accurately suppressing the complex noises. The improved decomposition method overcomes signal distortion and reduces frequency aliasing, providing clearer information for feature extraction. The tree-structure ensemble model exhibits high accuracy and strong generalization, ensuring the low-attenuation recovery of effective signals. Furthermore, this research reveals the mechanism by which noise interferes with signals and identifies the dominant frequency of random noise.
更多查看译文
关键词
borehole seismic record filtering,improved intrinsic time-scale decomposition algorithm,feature description
AI 理解论文
溯源树
样例
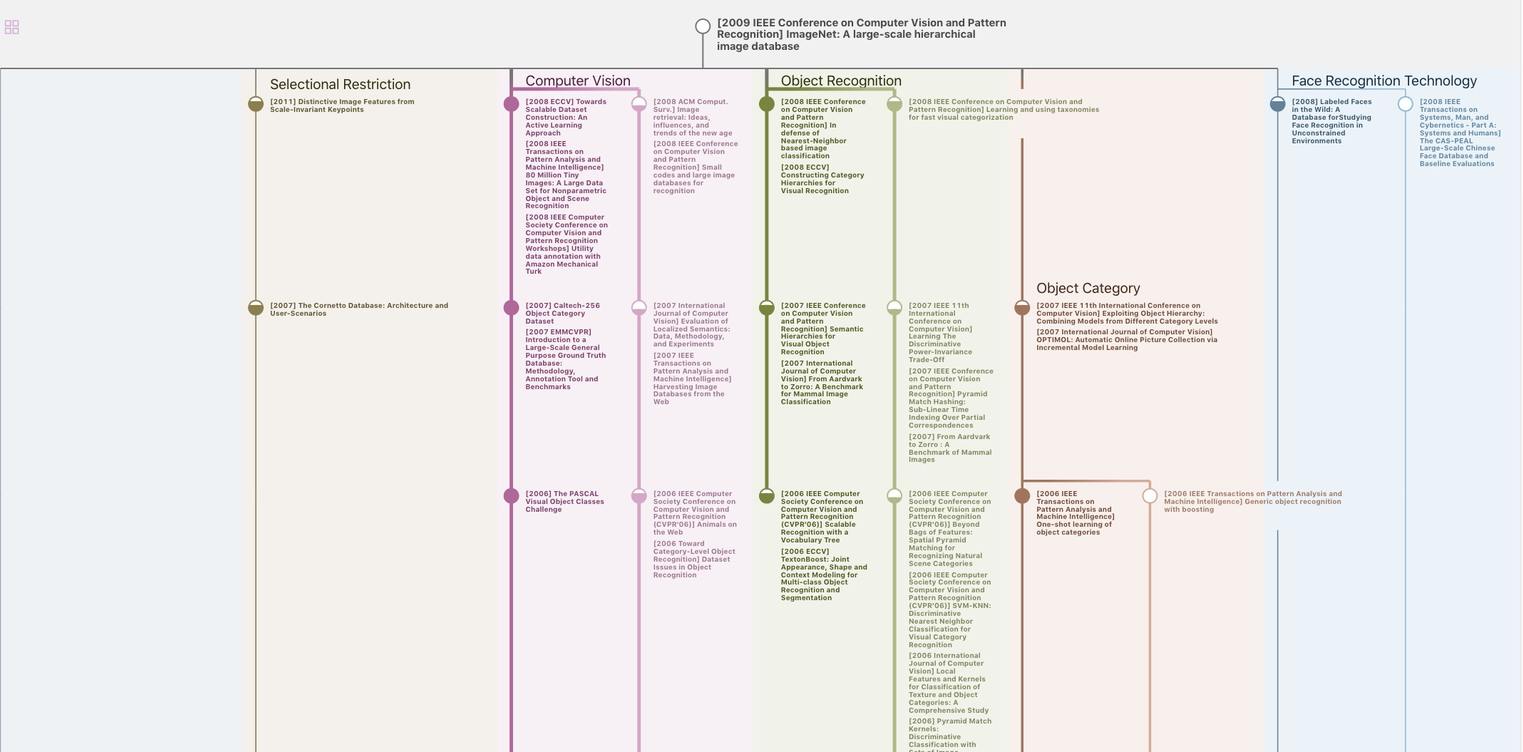
生成溯源树,研究论文发展脉络
Chat Paper
正在生成论文摘要