OoD-Control: Generalizing Control in Unseen Environments.
IEEE transactions on pattern analysis and machine intelligence(2024)
摘要
Generalizing out-of-distribution (OoD) is critical but challenging in real applications such as unmanned aerial vehicle (UAV) flight control. Previous machine learning-based control has shown promise in dealing with complex real-world environments but suffers huge performance degradation facing OoD scenarios, posing risks to the stability and safety of UAVs. In this paper, we found that the introduced random noises during training surprisingly yield theoretically guaranteed performances via a proposed functional optimization framework. More encouragingly, this framework does not involve common Lyapunov assumptions used in this field, making it more widely applicable. With this framework, the upperbound for control error is induced. We also proved that the induced random noises can lead to lower OoD control errors. Based on our theoretical analysis, we further propose OoD-Control to generalize control in unseen environments. Numerical experiments demonstrate the superiority of the proposed algorithm, surpassing previous state-of-the-art by 65% under challenging unseen environments. We further extend to outdoor real-world experiments and found that the control error is reduced by 50% approximately.
更多查看译文
关键词
Data-driven UAV control,out-of-distribution generalization,theoretical guaranteed performance
AI 理解论文
溯源树
样例
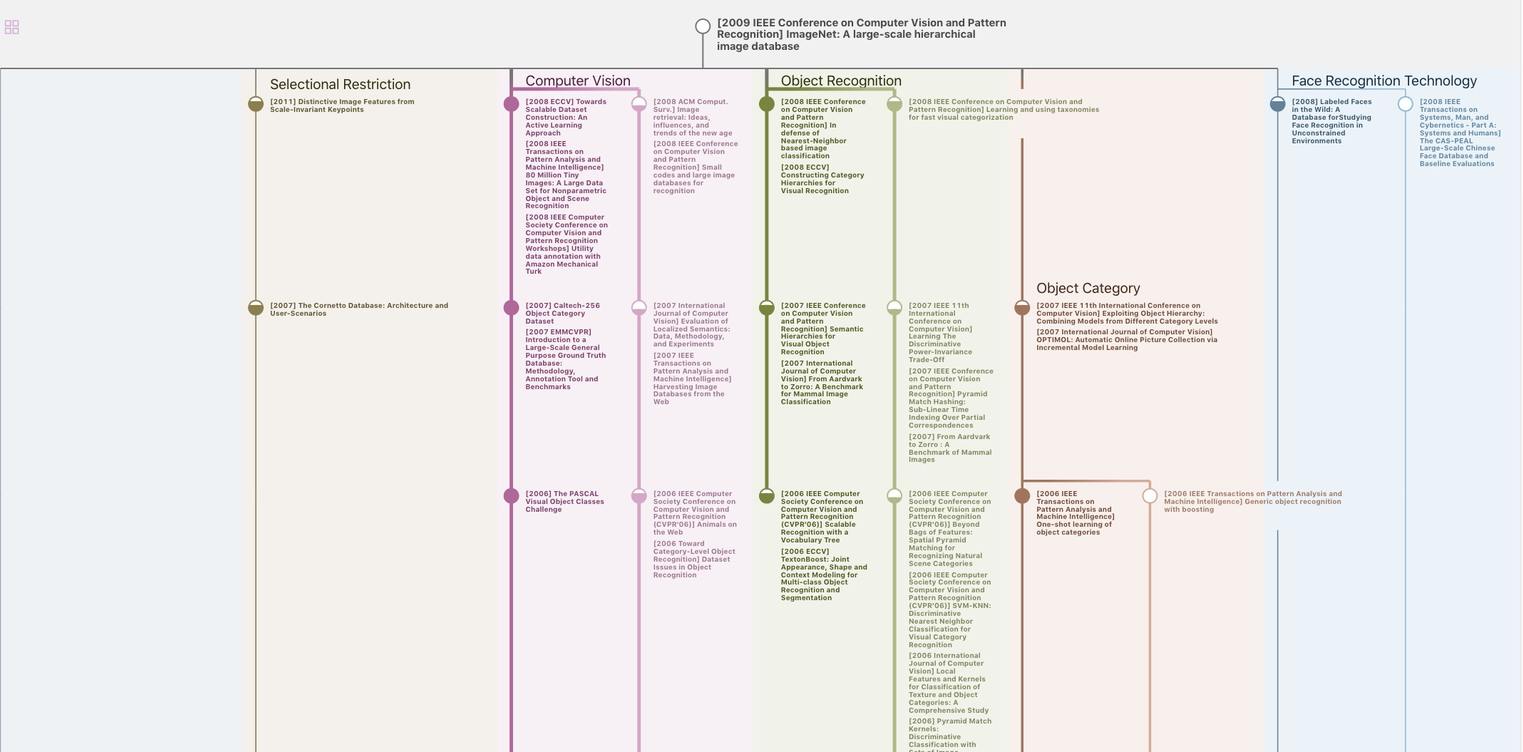
生成溯源树,研究论文发展脉络
Chat Paper
正在生成论文摘要