MFNet: Meta-learning Based on Frequency-Space Mix for MRI Segmentation in Nasopharyngeal Carcinoma
Journal of Cellular and Molecular Medicine(2024)
摘要
Deep learning techniques have been applied to medical image segmentation and demonstrated expert-level performance. Due to the poor generalization abilities of the models in the deployment in different centres, common solutions, such as transfer learning and domain adaptation techniques, have been proposed to mitigate this issue. However, these solutions necessitate retraining the models with target domain data and annotations, which limits their deployment in clinical settings in unseen domains. We evaluated the performance of domain generalization methods on the task of MRI segmentation of nasopharyngeal carcinoma (NPC) by collecting a new dataset of 321 patients with manually annotated MRIs from two hospitals. We transformed the modalities of MRI, including T1WI, T2WI and CE-T1WI, from the spatial domain to the frequency domain using Fourier transform. To address the bottleneck of domain generalization in MRI segmentation of NPC, we propose a meta-learning approach based on frequency domain feature mixing. We evaluated the performance of MFNet against existing techniques for generalizing NPC segmentation in terms of Dice and MIoU. Our method evidently outperforms the baseline in handling the generalization of NPC segmentation. The MF-Net clearly demonstrates its effectiveness for generalizing NPC MRI segmentation to unseen domains (Dice = 67.59%, MIoU = 75.74% T1W1). MFNet enhances the model's generalization capabilities by incorporating mixed-feature meta-learning. Our approach offers a novel perspective to tackle the domain generalization problem in the field of medical imaging by effectively exploiting the unique characteristics of medical images.
更多查看译文
关键词
data augmentation,domain generalization,feature mix,image segmentation,nasopharyngeal carcinoma
AI 理解论文
溯源树
样例
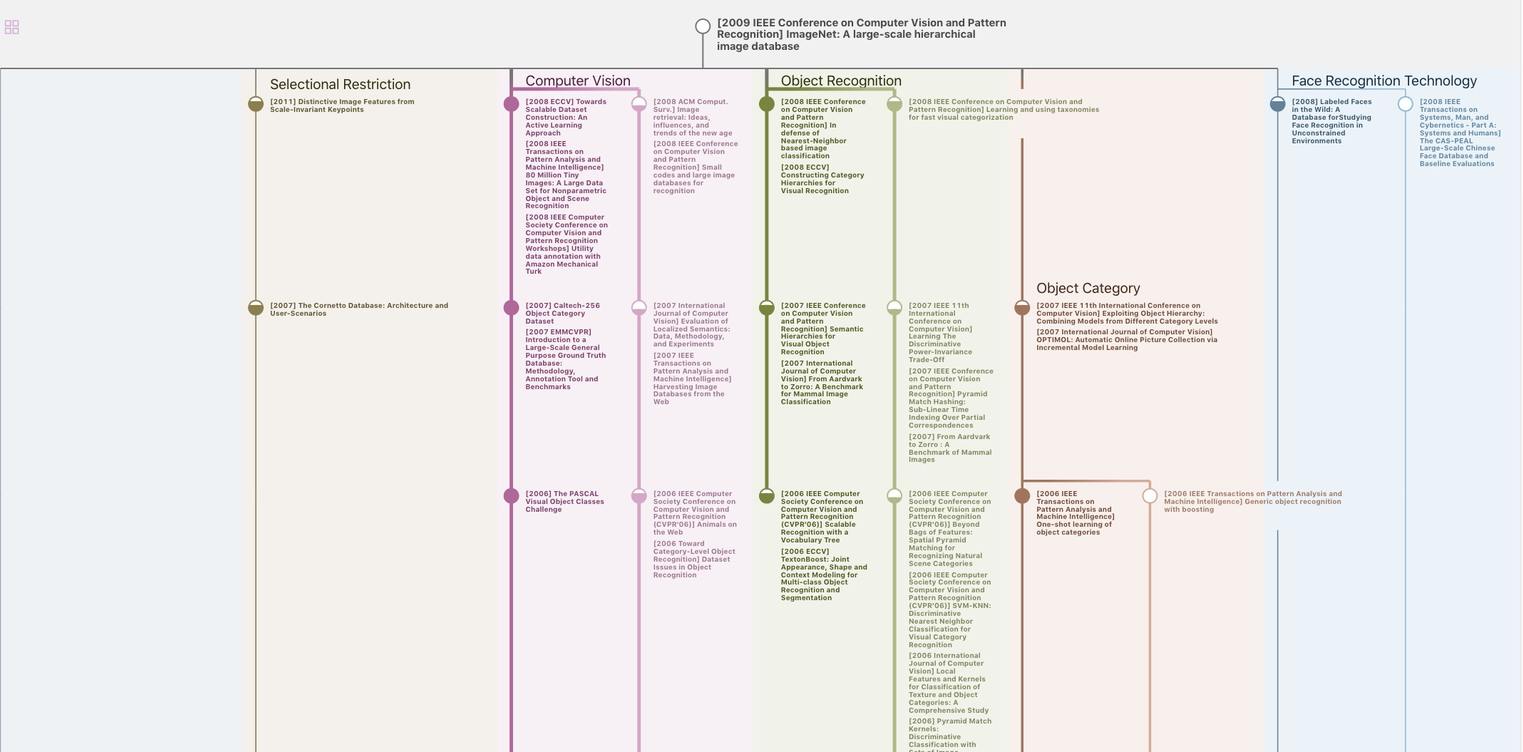
生成溯源树,研究论文发展脉络
Chat Paper
正在生成论文摘要