Deciphering and predicting changes in antibiotic resistance genes during pig manure aerobic composting via machine learning model
Environmental Science and Pollution Research(2024)
摘要
Livestock manure is one of the most important pools of antibiotic resistance genes (ARGs) in the environment. Aerobic composting can effectively reduce the spread of antibiotic resistance risk in livestock manure. Understanding the effect of aerobic composting process parameters on manure-sourced ARGs is important to control their spreading risk. In this study, the effects of process parameters on ARGs during aerobic composting of pig manure were explored through data mining based on 191 valid data collected from literature. Machine learning (ML) models (XGBoost and Random Forest) were utilized to predict the rate of ARGs changes during pig manure composting. The model evaluation index of the XGBoost model (R2 = 0.651) was higher than that of the Random Forest (R2 = 0.490), indicating that XGBoost had better prediction performance. Feature importance was further calculated for the XGBoost model, and the XGBoost black box model was interpreted by Shapley additive explanations analysis. Results indicated that the influencing factors on the ARGs variation in pig manure were sequentially divided into thermophilic period, total composting period, composting real time, and thermophilic stage average temperature. The findings gave an insight into the application of ML models to predict and decipher the ARG changes during manure composting and provided suggestions for better composting manipulation and optimization of process parameters.
更多查看译文
关键词
Thermophilic composting,Swine manure,Antibiotic resistance,Machine learning,Model interpretation
AI 理解论文
溯源树
样例
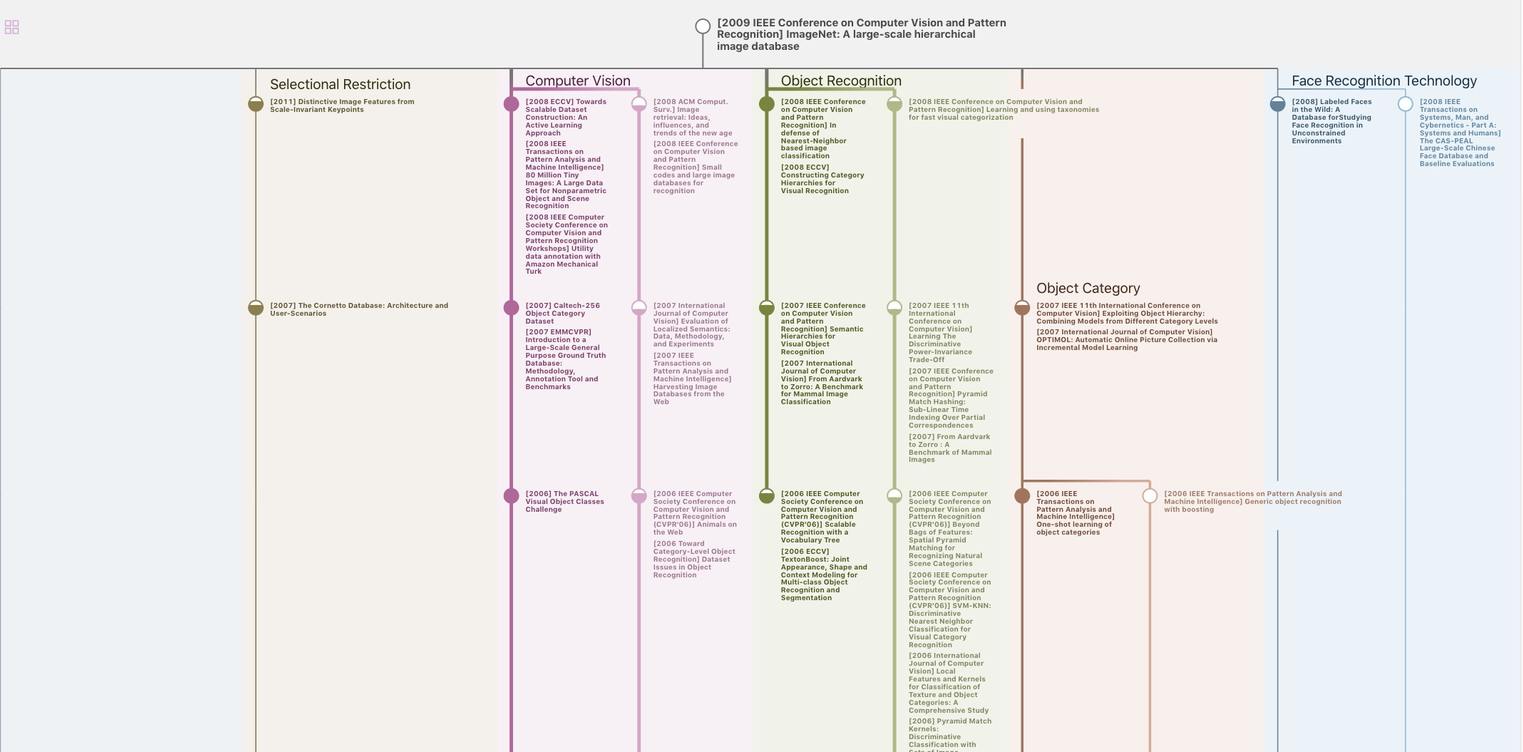
生成溯源树,研究论文发展脉络
Chat Paper
正在生成论文摘要