A Hybrid CNN-GRU model for Session-based Recommender Systems
2023 9th International Conference on Computer and Communications (ICCC)(2023)
摘要
In many e-commerce recommendation systems, user profiles are mostly not available. In such cases, the recommendation task considers user behaviors like click sessions or browsing history. Traditional recommender methods often rely on users’ past interactions for recommending user interests, which is difficult to apply to session-based recommender systems. However, these methods perform very poorly on this task. Recently, deep learning techniques such as recurrent neural networks (RNNs) and convolutional neural networks (CNNs) showed outstanding performance in session-based recommendation problems. In this paper, we propose a hybrid CNN and gated recurrent unit (GRU) model that exploits the advantages of both CNN and GRU in modeling the rigid order requirement for interactions inside sessions and modeling the sequential long-term dependencies in session data, respectively. Our proposed hybrid model has two architectures: the first part consists of the CNN layers that take input from Item ID to learn local features and relationships in sessions, and the second part is a GRU layer that takes the output from the CNN layer to learn the long-term dependencies in that session. This hybrid technique enables the architecture for modeling prominent features in the session to provide accurate recommendations. Extensive experiments over a real-world dataset demonstrate that the proposed hybrid model performs well and outperforms those GRU and CNN-based models in the state-of-the-art recommendation task on both metrics used.
更多查看译文
AI 理解论文
溯源树
样例
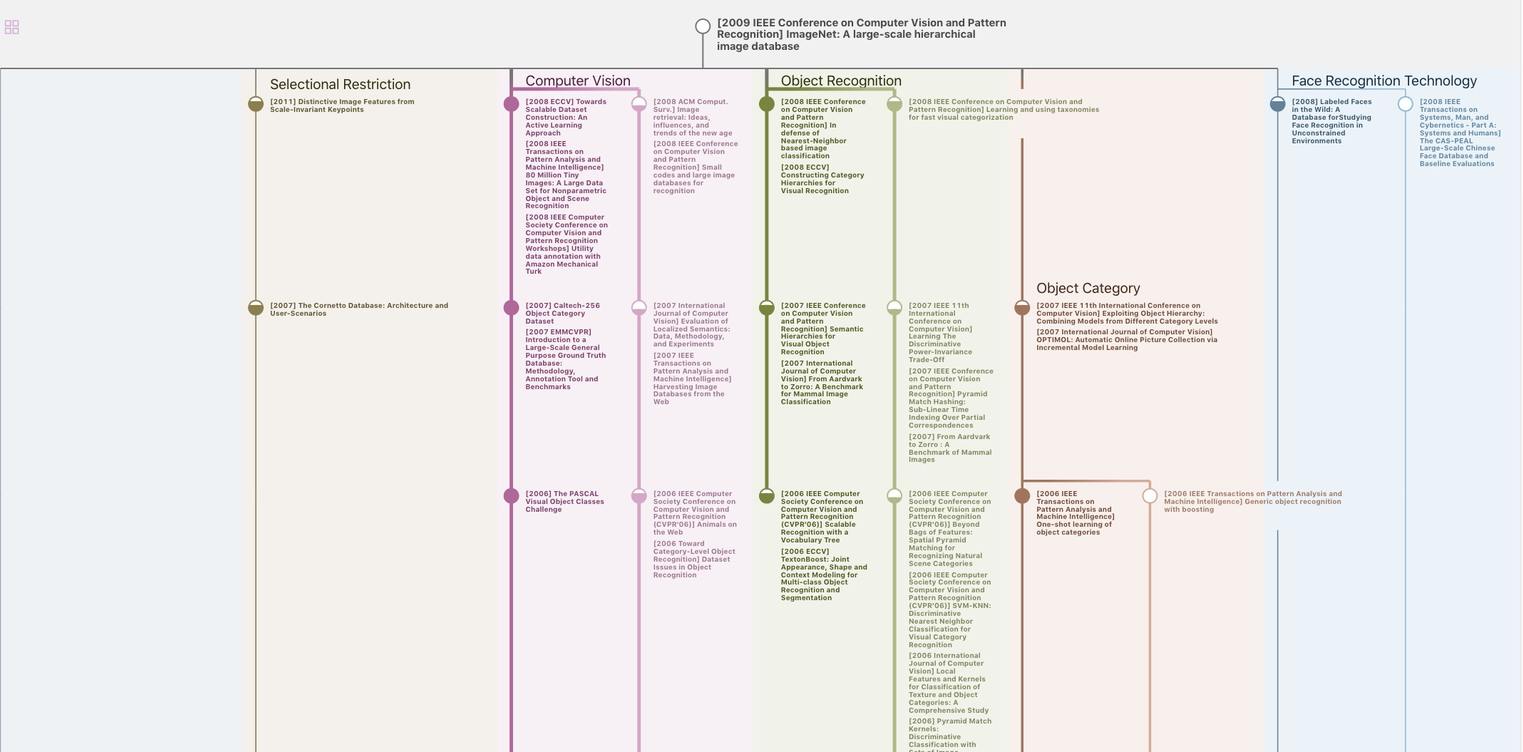
生成溯源树,研究论文发展脉络
Chat Paper
正在生成论文摘要