A trustworthy, data-driven, decision-making framework for global infectious disease mitigation (Preprint)
crossref(2024)
摘要
UNSTRUCTURED Infectious diseases (ID) have a significant detrimental impact on global health. Timely and accurate infectious disease forecasting can result in more informed implementation of control measures and prevention policies. However, for operational decision-making in real-world circumstances, a standardized, reliable, and trustworthy platform that can forecast multiple diseases across different geographic locations is largely lacking. To meet this need, we built an ID forecasting pipeline and visualization dashboard generalized across a wide range of modeling techniques, IDs, and global locations. We included a wide range of statistical, machine learning, and deep learning models and trained them on a multitude of features (e.g., demography, landscape, climate, and socioeconomic factors) within the One Health landscape. The dashboard was built to report crucial operational metrics - prediction accuracy, computational efficiency, spatio-temporal generalizability, uncertainty quantification, and interpretability - which are essential to strategic data-driven decisions. While no single best model was suitable for all disease, region, and country combinations, our ensemble technique selects the best model for any given scenario, achieving peak forecasting performance. For new or emerging diseases in a region, the ensemble model can predict how the disease may behave in the new region using a pre-trained model from a similar region with a history of that disease. The data visualization dashboard provides an interactive, clean interface of important analytical metrics, such as ID temporal patterns, forecasts, prediction uncertainties, and model feature importance across geographic locations and disease combinations. As the need for real-time, operational ID forecasting capabilities increases, this standardized and automated platform for overall data collection, analysis, and reporting is a major step forward in enabling evidence-based public health decisions and policies for prevention and mitigation of future ID outbreaks.
更多查看译文
AI 理解论文
溯源树
样例
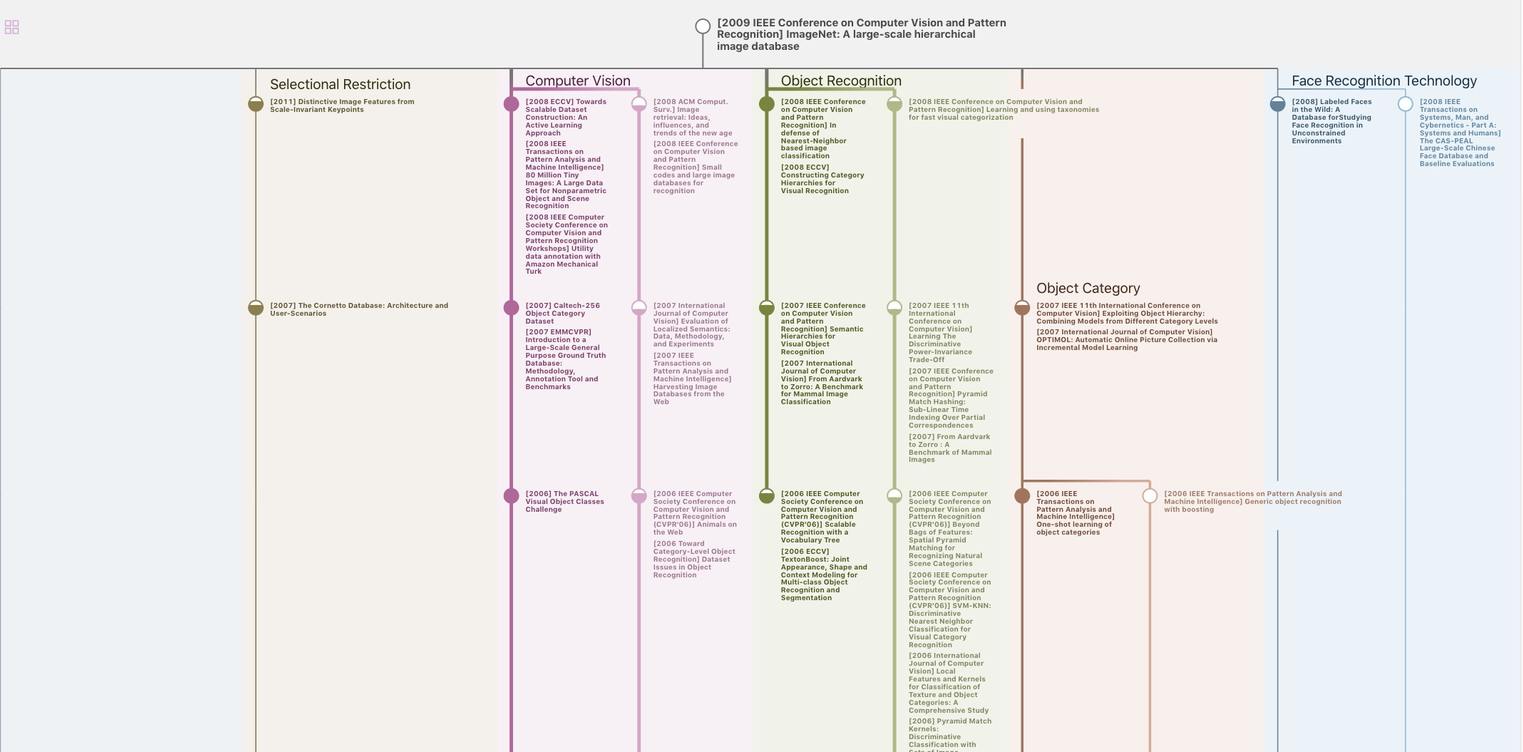
生成溯源树,研究论文发展脉络
Chat Paper
正在生成论文摘要