Insights into Low-Cost Pm Sensors Using Size-Resolved Scattering Intensity of Cooking Aerosols in a Test House
Aerosol science and technology(2024)
摘要
Spatio-temporal particulate matter (PM) measurements are critical to understanding emission profiles to develop effective mitigation strategies. However, high-resolution spatio-temporal measurements using research-grade instruments are cost-prohibitive, making low-cost sensors (LCS) a promising alternative. LCS measures particle light scattering intensity (Isc) and converts it to mass and number concentrations. Such measurements are influenced by particles' physico-optical properties, which make LCS appropriate for measuring PM with similar properties to those used for calibration. This work evaluates the performance of an LCS (PurpleAir) against reference instruments (SMPS + APS) during cooking experiments performed in a test house where PM0.3/PM10 as high as 90% was observed. LCS demonstrated better correlations (R2) for PM1 (0.98-1), PM2.5 (0.96-1), and PM10 (0.88-1) compared to coarser size bins, i.e., PM1-2.5 (0.04-0.99) and PM2.5-10 (0.02-0.93). Measurements from different cooking activities show different linear fits. Isc calculated using size-resolved measurements of reference instruments also had different linear fits with corresponding mass concentrations, even after assuming the same refractive index for all cases, highlighting the dependence of size distribution on LCS measurements. On average, sub-300 nm particles accounted for up to 75 +/- 18% of the total Isc. Such dominance of sub-300 nm particles leads to size misclassification by LCS, whose algorithm assumes the inability to measure sub-300 nm particles. Moreover, the Isc calculated using the size distribution reported by LCS is considerably lower than that of reference instruments. This mismatch can be attributed to truncated angle error and positioning of particles in the sensing zone relative to the photodiode.Copyright (c) 2024 American Association for Aerosol Research
更多查看译文
关键词
Andrea Ferro
AI 理解论文
溯源树
样例
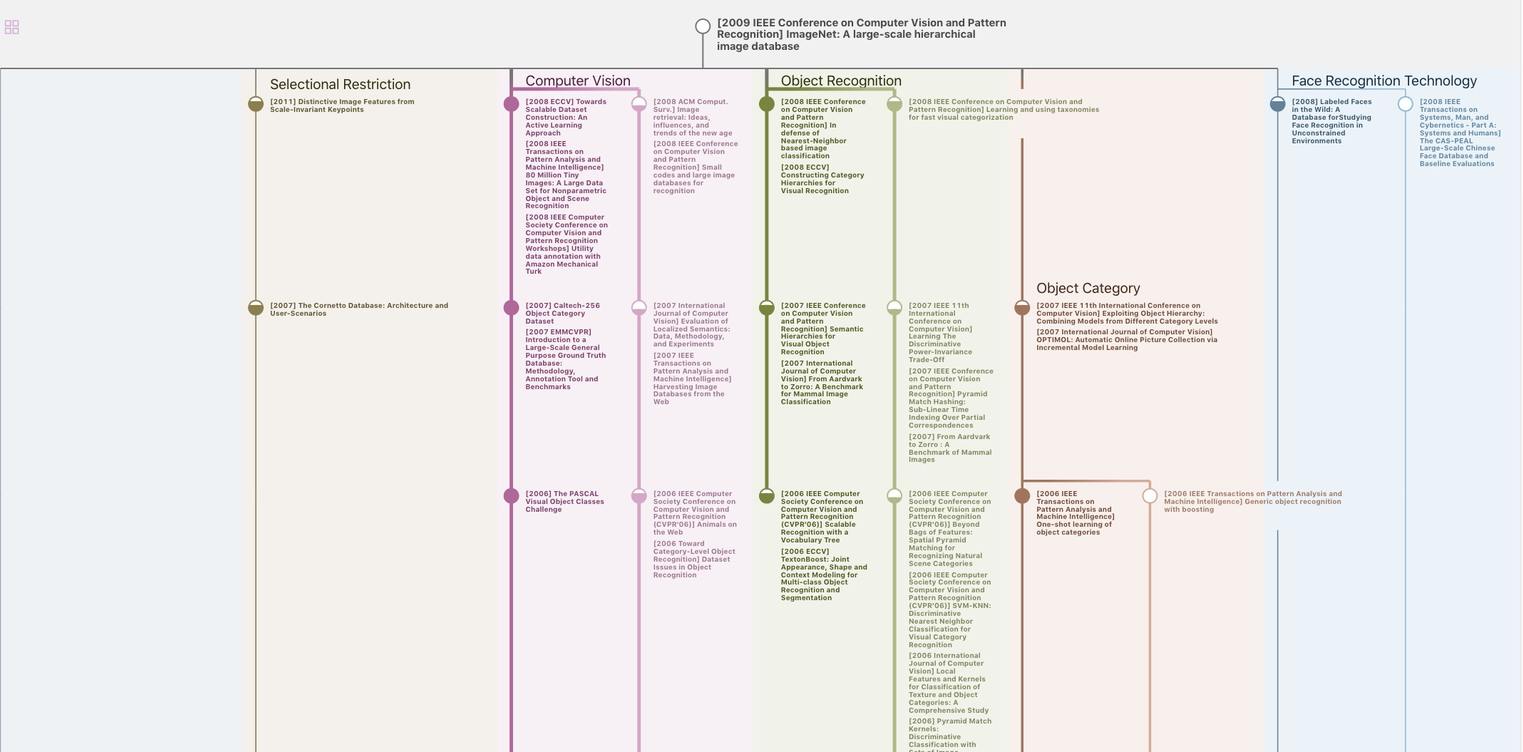
生成溯源树,研究论文发展脉络
Chat Paper
正在生成论文摘要