Flood Water Depth Prediction with Convolutional Temporal Attention Networks
Water(2024)
摘要
Robust and accurate flood hazard maps are essential for early warning systems and flood risk management. Although physically based models are effective in estimating pluvial flooding, the computational burden makes them difficult to use for real-time flood prediction. In contrast, data-driven models can provide faster flood predictions if trained offline. While most studies have focused on predicting maximum water depth, in this study, we predict pixel-wise water depth maps for entire catchments at a lead time of 2 h. To that end, we propose a deep learning approach that uses a sequence encoding network with temporal self-attention. We also adapt the popular hydrological performance metric Nash–Sutcliffe efficiency (NSE) as our loss function. We test the effectiveness and generalizability of our method using a new dataset called SwissFlood, which consists of 100 catchments and 1500 rainfall events extracted from real observations in Switzerland. Our method produces 2 m spatial resolution flood maps with absolute error as low as 27 cm for water depth exceeding 1 m.
更多查看译文
关键词
flood estimation,pluvial flooding,deep learning,flood forecasting
AI 理解论文
溯源树
样例
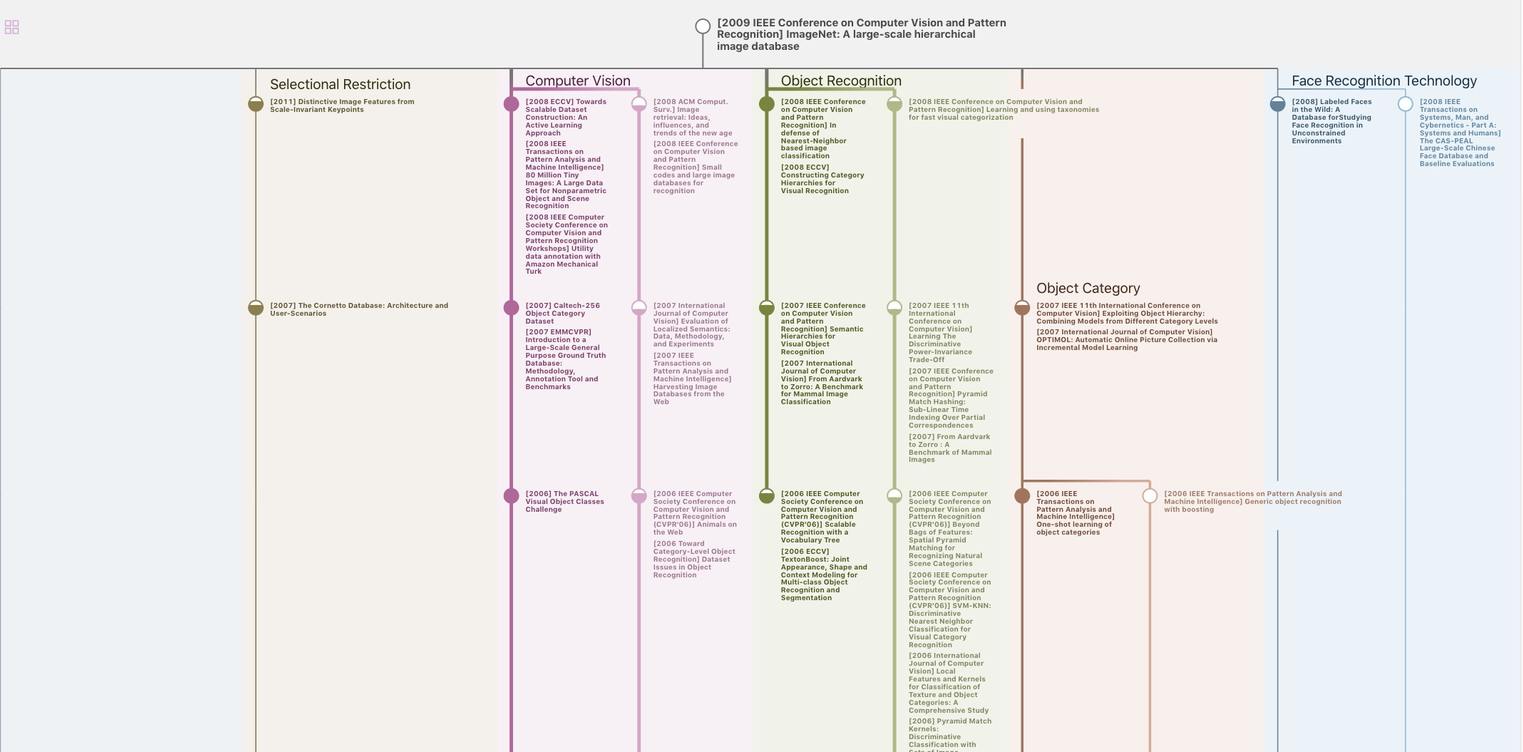
生成溯源树,研究论文发展脉络
Chat Paper
正在生成论文摘要