Deep Learning of a 200-member Ensemble with a Limited Historical Training to Improve the Prediction of Extreme Precipitation Events
Monthly Weather Review(2024)
摘要
Abstract This study introduces a deep learning (DL) scheme to generate reliable and skillful probabilistic quantitative precipitation forecasts (PQPFs) in a postprocessing framework. Enhanced machine learning model architecture and training mechanisms are proposed to improve reliability and skill of PQPFs while permitting computationally efficient model fitting using a short training dataset. The methodology is applied to postprocessing of 24h accumulated PQPFs from an ensemble forecast system recently introduced by the Center for Western Weather and Water Extremes (CW3E) and for lead times from one to six days. The ensemble system was designed based on a high-resolution version of the Weather Research and Forecasting (WRF) model, named West-WRF, to produce a 200-member ensemble in near real-time (NRT) over the Western U.S. during the boreal cool seasons to support forecastinformed reservoir operations (FIRO) and studies of prediction of heavy-to-extreme events. Postprocessed PQPFs are compared with those from the raw West-WRF ensemble, the operational Global Ensemble Forecast System (GEFSv12) as well as the ensemble from the European Centre for Medium-Range Weather Forecasts (ECMWF). As an additional baseline, we provide PQPFs verification metrics from a recently developed neural network postprocessing scheme proposed by Ghazvinian et al. (2022). Results demonstrate that skill of postprocessed forecasts significantly outperform PQPFs and deterministic forecasts from raw ensembles and the recently developed algorithm. The resulting PQPFs broadly improve upon the reliability and skill of baselines in predicting heavy to extreme precipitation (e.g., > 75 mm) across all lead times while maintaining the spatial structure of the high-resolution raw ensemble.
更多查看译文
AI 理解论文
溯源树
样例
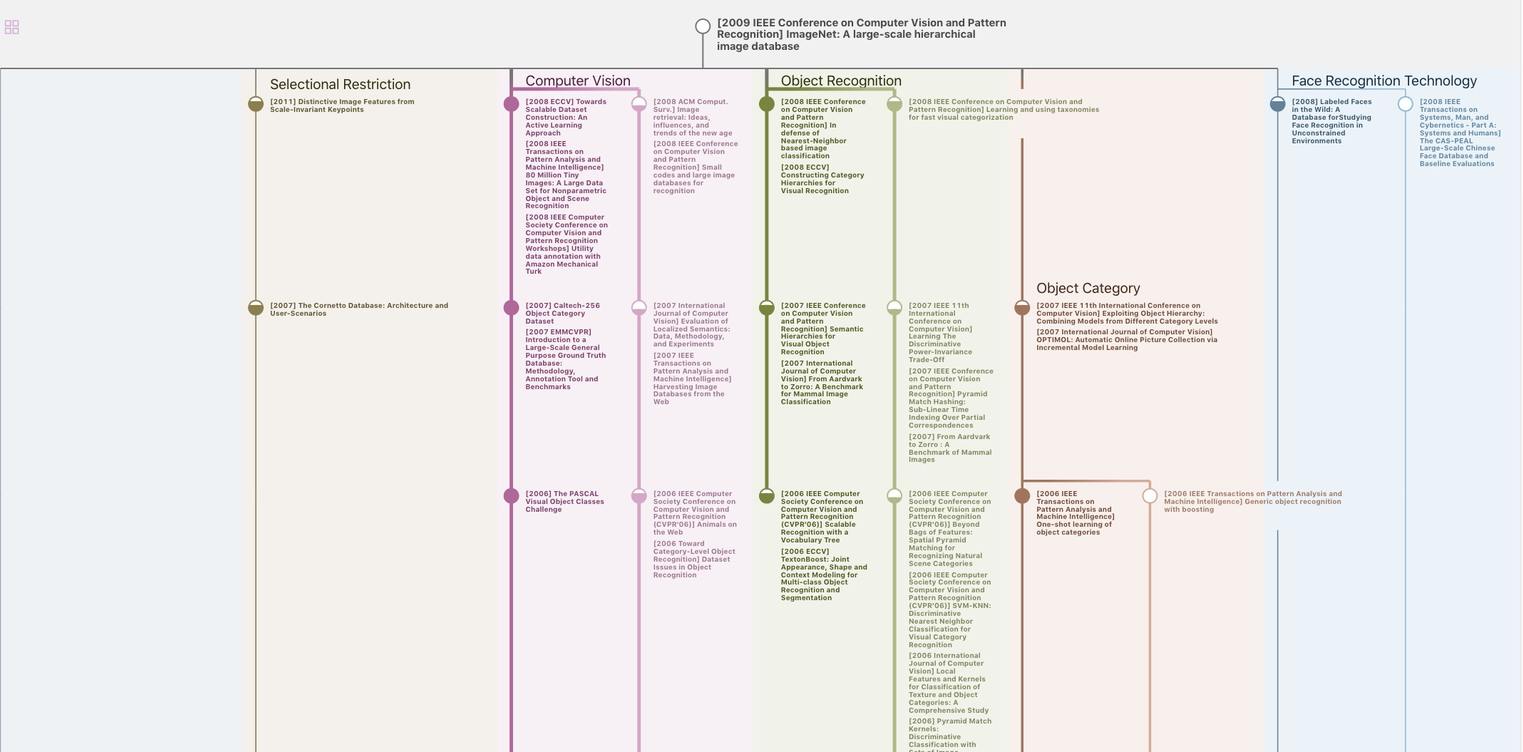
生成溯源树,研究论文发展脉络
Chat Paper
正在生成论文摘要