Machine Learning Data Augmentation Strategy for Electron Energy Loss Spectroscopy: Generative Adversarial Networks
MICROSCOPY AND MICROANALYSIS(2024)
摘要
Recent advances in machine learning (ML) have highlighted a novel challenge concerning the quality and quantity of data required to effectively train algorithms in supervised ML procedures. This article introduces a data augmentation (DA) strategy for electron energy loss spectroscopy (EELS) data, employing generative adversarial networks (GANs). We present an innovative approach, called the data augmentation generative adversarial network (DAG), which facilitates data generation from a very limited number of spectra, around 100. Throughout this study, we explore the optimal configuration for GANs to produce realistic spectra. Notably, our DAG generates realistic spectra, and the spectra produced by the generator are successfully used in real-world applications to train classifiers based on artificial neural networks (ANNs) and support vector machines (SVMs) that have been successful in classifying experimental EEL spectra.
更多查看译文
关键词
data augmentation,electron energy loss spectroscopy,generative adversarial networks,machine learning,support vector machines
AI 理解论文
溯源树
样例
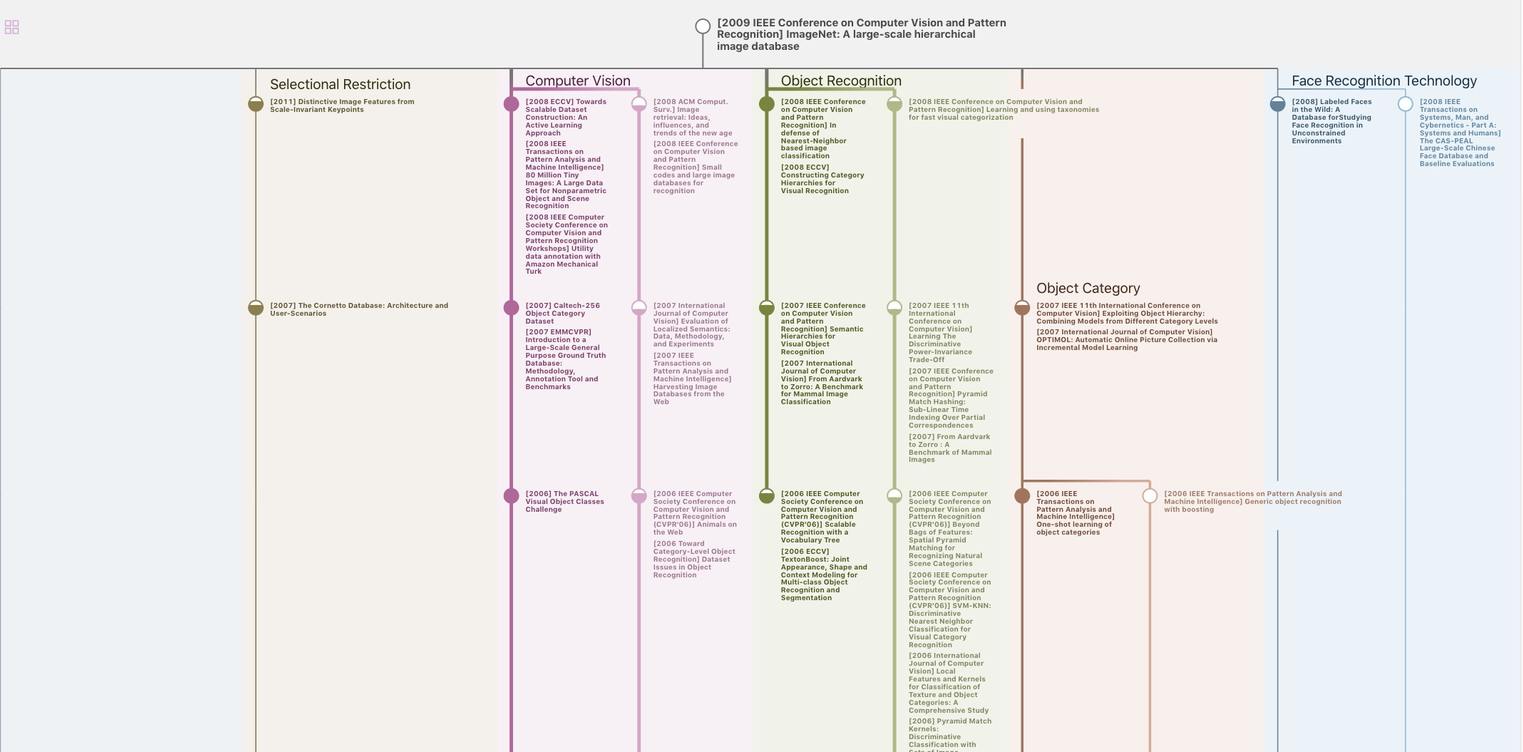
生成溯源树,研究论文发展脉络
Chat Paper
正在生成论文摘要