Evoked Component Analysis (ECA): Decomposing the Functional Ultrasound Signal with GLM-Regularization.
IEEE transactions on bio-medical engineering(2024)
摘要
Analysis of functional neuroimaging data aims to unveil spatial and temporal patterns of interest. Existing analysis methods fall into two categories: fully data-driven approaches and those reliant on prior information, e.g. the stimulus time course. While using the stimulus signal directly can help identify the activated brain areas, it is known that the relationship between stimuli and the brain's response exhibits nonlinear and time-varying characteristics. As such, relying completely on the stimulus signal to describe the brain's temporal response leads to a restricted interpretation of the brain function. In this paper, we present a new technique called Evoked Component Analysis (ECA), which leverages prior information up to a defined extent. This is achieved by including the general linear model (GLM) design matrix as a regulatory term and estimating the factor matrices in both space and time through an alternating minimization approach. We apply ECA to 2D and swept-3D functional ultrasound (fUS) experiments conducted with mice. When decomposing 2D fUS data, we employ GLM regularization at various intensities to emphasize the role of prior information. Furthermore, we show that incorporating multiple hemodynamic response functions within the design matrix can provide valuable insights into region-specific characteristics of evoked activity. Finally, we use ECA to analyze swept-3D fUS data recorded from five mice engaged in two distinct visual tasks. Swept-3D fUS images the 3D brain sequentially using a moving probe, resulting in different slice acquisition time instants. We show that ECA can estimate factor matrices with a fine resolution at each slice acquisition time instant and yield higher t-statistics compared to GLM and correlation analysis for all subjects.
更多查看译文
AI 理解论文
溯源树
样例
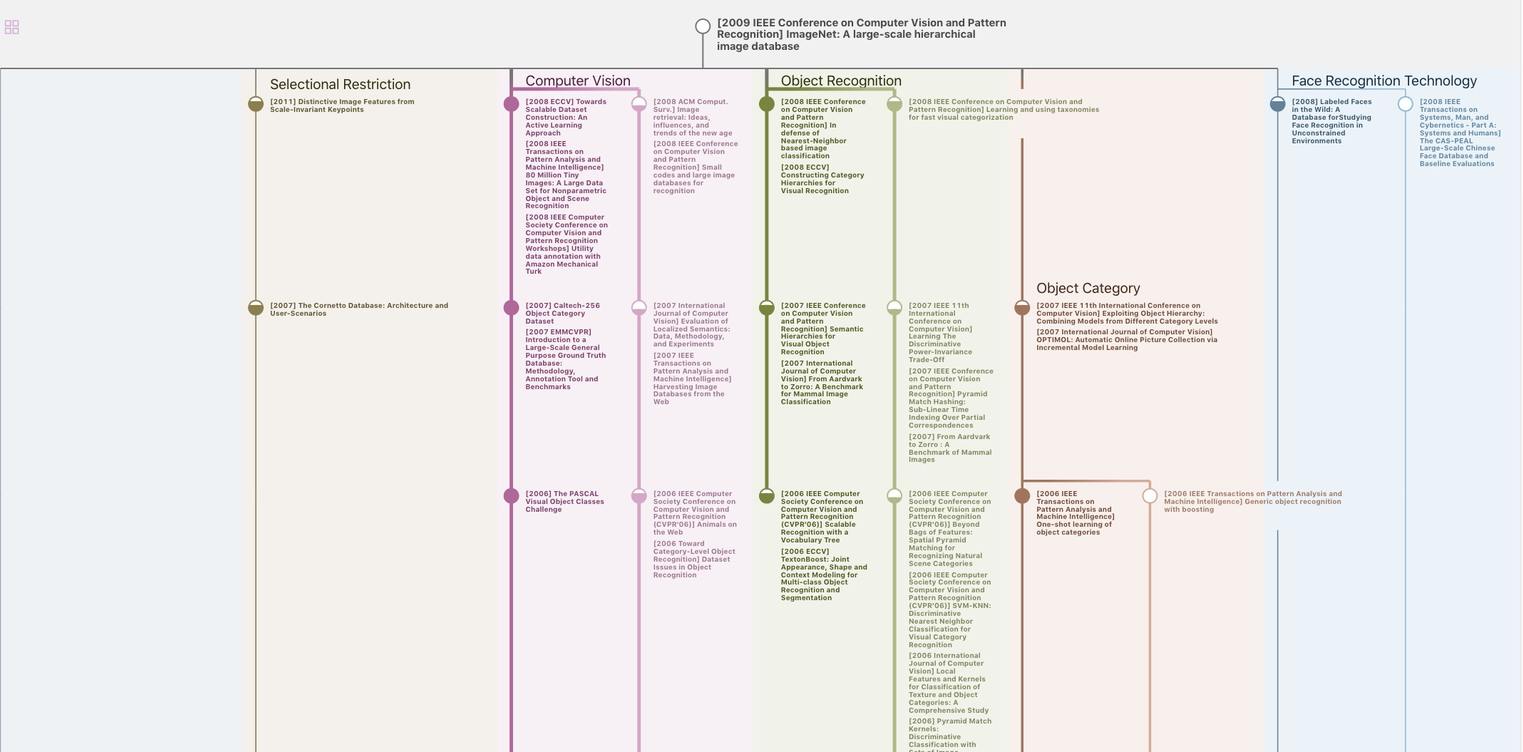
生成溯源树,研究论文发展脉络
Chat Paper
正在生成论文摘要