A CNN-LSTM Phase Compensation Method for Unidirectional Two-way Radio Frequency Transmission System
IEEE Photonics Journal(2024)
摘要
A convolutional neural network combined with long short-term memory (CNN-LSTM) phase compensation method (PCM) is proposed and demonstrated, where CNN is employed to extract spatial features, and LSTM is used to capture temporal features and realize the long-term predictions of residual phase fluctuations. This is the first-time machine learning (ML) has been used to mitigate the effects of optical path asymmetry caused by temperature variations on radio frequency (RF) transmission systems. The performance is verified by experiments on a unidirectional two-way RF transmission system, in which both the two 259-km-long separate fibers are coupled into one optical cable. The results demonstrate the CNN-LSTM model presents better prediction performance than the other eight previously proposed ML models. When the prediction duration is 40,000 s and the ambient temperature variation range is 14.38°C, the coefficient of determination (R Squared, R2) between the predicted value and the actual value is higher than 0.99. In addition, compared to the phase locked loop (PLL) PCM, the proposed CNN-LSTM PCM can reduce the root-mean-square (RMS) phase jitter of the received signal from 219 ps to 19.72 ps, and improve the frequency stability of the system at 10,000 s by 84.5%. Overall, the proposed CNN-LSTM PCM can effectively compensate for residual phase fluctuations generated by the optical path asymmetry, providing a potential option for achieving stable RF transmission in telecommunication networks.
更多查看译文
关键词
convolutional neural network,frequency transmission,long short-term memory network,unidirectional two-way,frequency stability
AI 理解论文
溯源树
样例
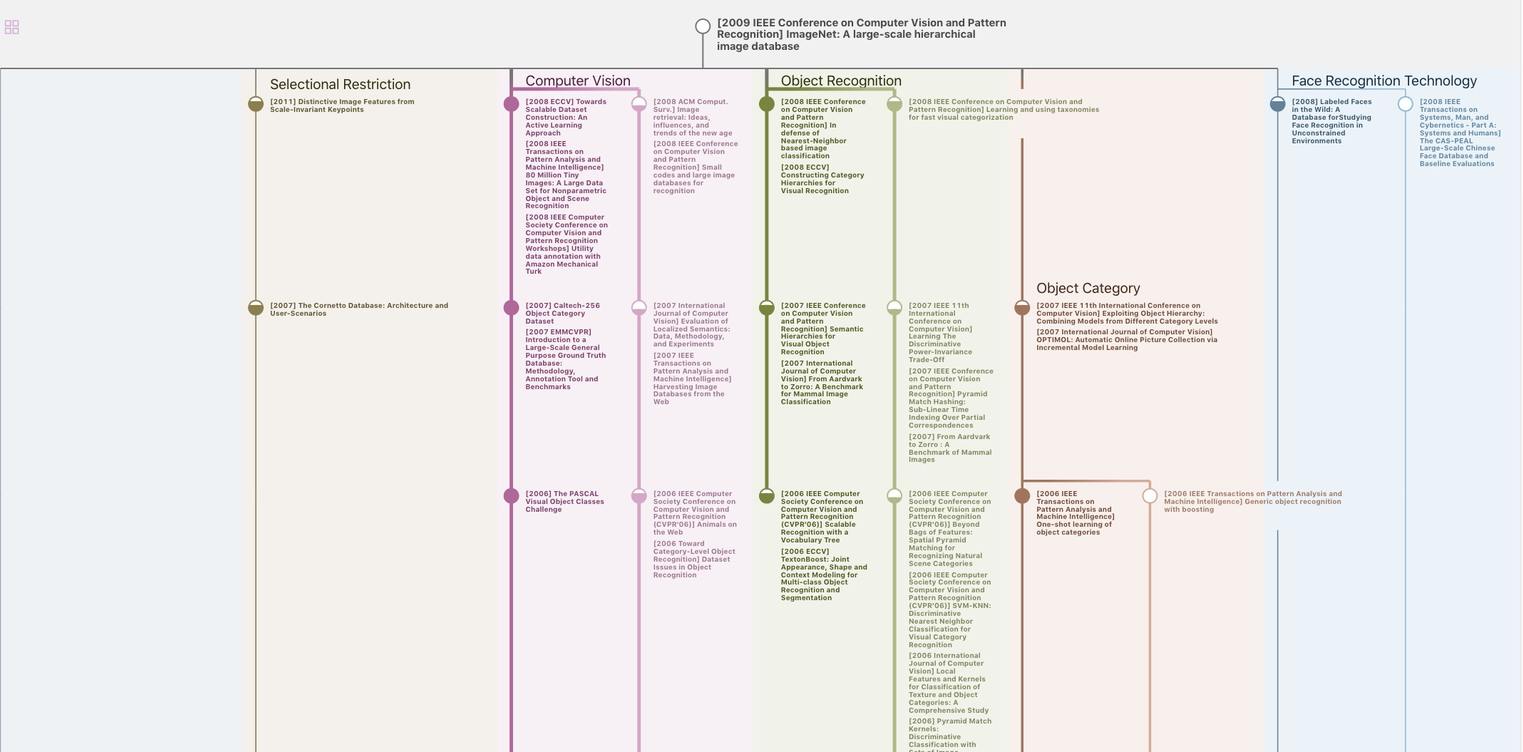
生成溯源树,研究论文发展脉络
Chat Paper
正在生成论文摘要