Bridging Auditory Perception and Natural Language Processing with Semantically Informed Deep Neural Networks
Scientific Reports(2024)
摘要
Sound recognition is effortless for humans but poses a significant challenge for artificial hearing systems. Deep neural networks (DNNs), especially convolutional neural networks (CNNs), have recently surpassed traditional machine learning in sound classification. However, current DNNs map sounds to labels using binary categorical variables, neglecting the semantic relations between labels. Cognitive neuroscience research suggests that human listeners exploit such semantic information besides acoustic cues. Hence, our hypothesis is that incorporating semantic information improves DNN’s sound recognition performance, emulating human behaviour. In our approach, sound recognition is framed as a regression problem, with CNNs trained to map spectrograms to continuous semantic representations from NLP models (Word2Vec, BERT, and CLAP text encoder). Two DNN types were trained: semDNN with continuous embeddings and catDNN with categorical labels, both with a dataset extracted from a collection of 388,211 sounds enriched with semantic descriptions. Evaluations across four external datasets, confirmed the superiority of semantic labeling from semDNN compared to catDNN, preserving higher-level relations. Importantly, an analysis of human similarity ratings for natural sounds, showed that semDNN approximated human listener behaviour better than catDNN, other DNNs, and NLP models. Our work contributes to understanding the role of semantics in sound recognition, bridging the gap between artificial systems and human auditory perception.
更多查看译文
关键词
Sound recognition,Deep neural networks,Semantic embeddings,Natural language processing,Cognitive neuroscience,Auditory perception,Acoustic-to-semantic transformation
AI 理解论文
溯源树
样例
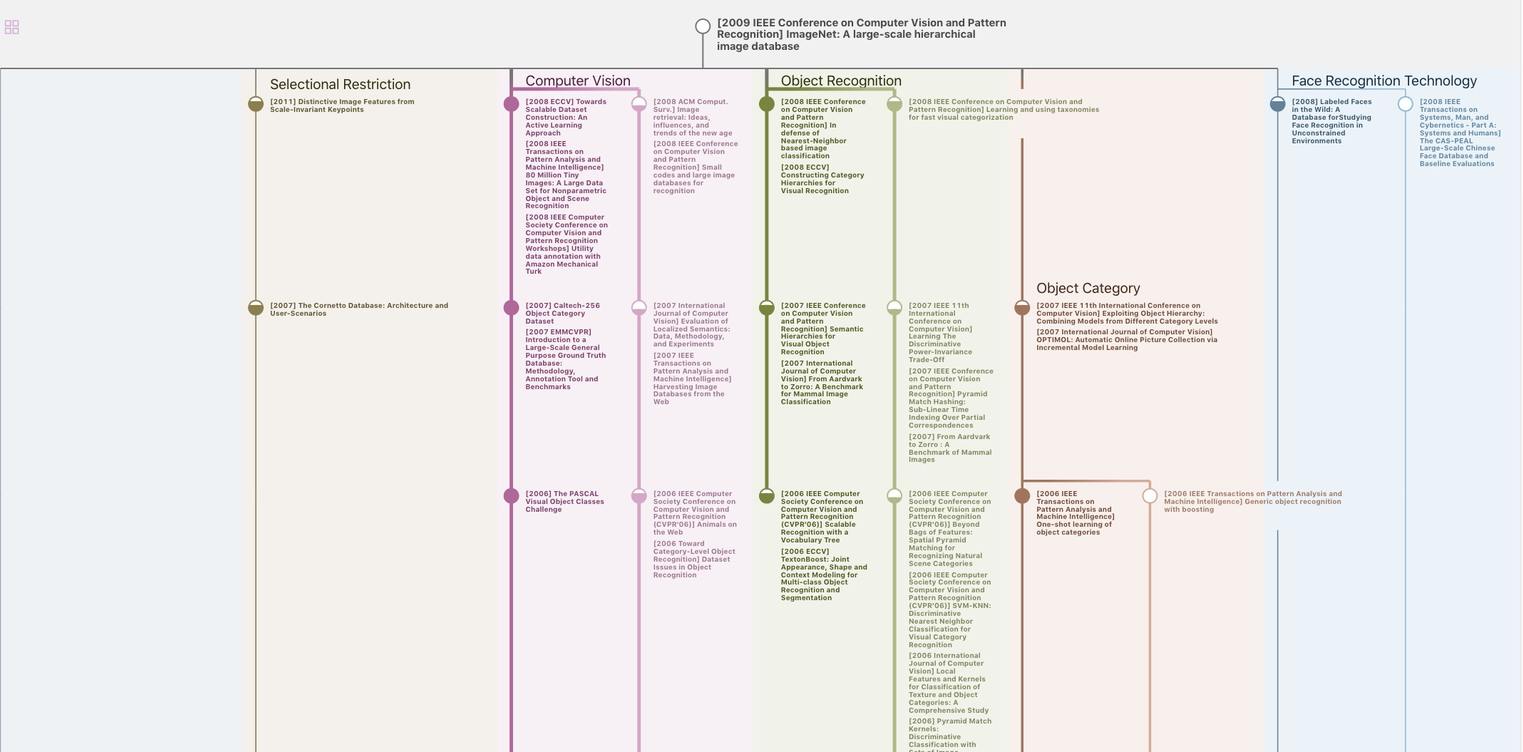
生成溯源树,研究论文发展脉络
Chat Paper
正在生成论文摘要