FTEKFNet: Hybridizing Physical and Data-driven Estimation Algorithms for Vehicle State
IEEE Transactions on Intelligent Vehicles(2024)
摘要
Sideslip angle and vehicle velocity are crucial for both traditional and autonomous vehicles. They play essential roles in chassis stability control, as well as in tasks such as path planning and tracking control. However, these states cannot be directly measured by onboard sensors, therefore various vehicle state estimation algorithms have been developed. Most of these algorithms assume that the noise characteristics are known, ignoring the impact of missing measurement data, and cannot simultaneously handle the effects of colored noise and white noise. To address these issues, we propose a fault-tolerant extended Kalman filter network (FTEKFNet), which integrates both physics-based and data-driven methods for vehicle state estimation. Based on the Fault Tolerant Extended Kalman Filter (FTEKF) iterative framework, a pre-trained artificial neural network is utilized to directly predict the Kalman gain, and it is combined with FTEKF to form FTEKFNet. Experimental results under different conditions demonstrate that FTEKFNet can simultaneously deal with unknown noise and data loss problems and has good adaptability to color noise. The estimation performance of the proposed algorithm is better than the traditional FTEKF and EKF methods.
更多查看译文
关键词
Vehicle state estimation,fault-tolerant extended Kalman filter network,measurement data loss,colored noise
AI 理解论文
溯源树
样例
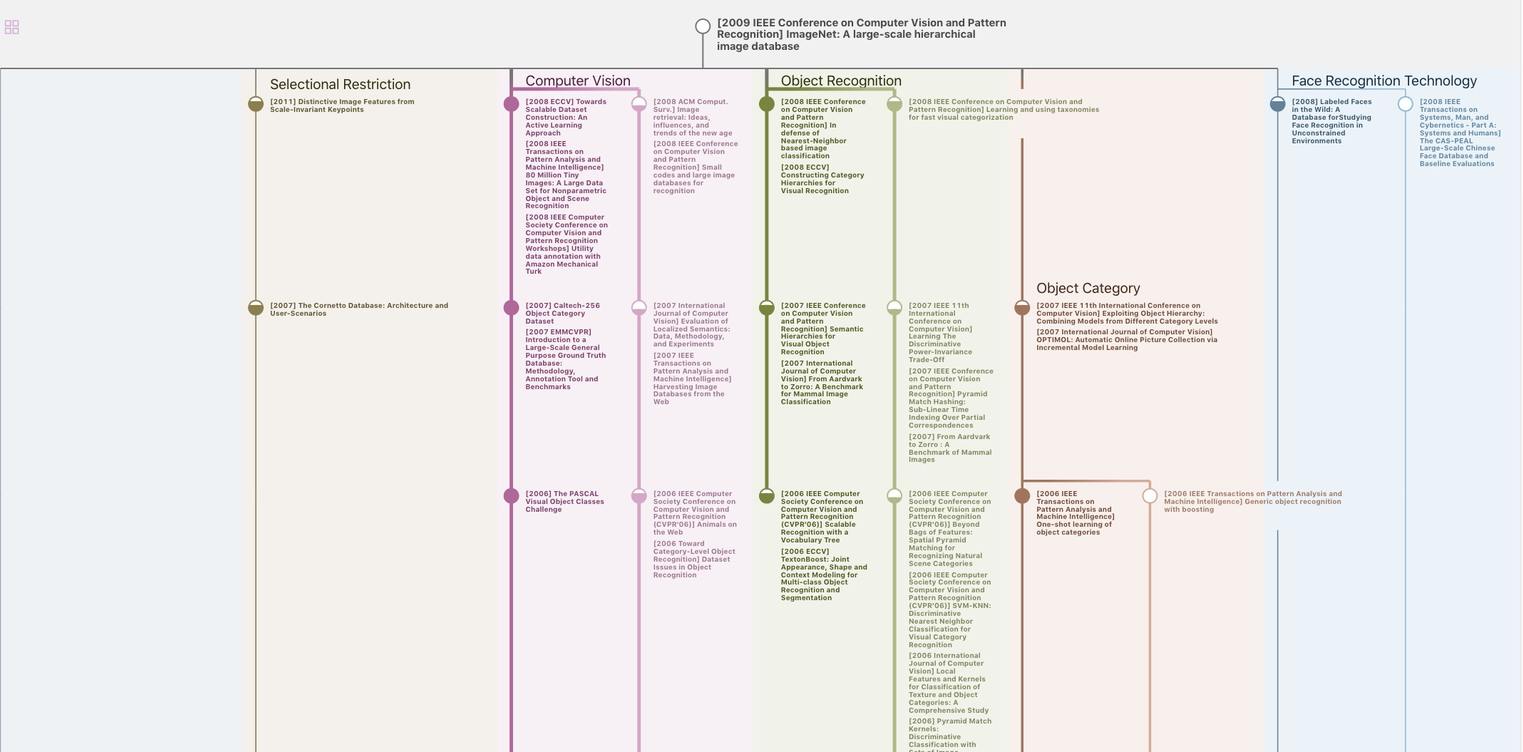
生成溯源树,研究论文发展脉络
Chat Paper
正在生成论文摘要