Unsupervised 3D Array-SAR Imaging Based on Generative Model for Scattering Diagnosis
IEEE Antennas and Wireless Propagation Letters(2024)
摘要
Scattering diagnosis requires the spatial distribution of the target's scattering coefficient, which can be obtained through radar imaging, specifically 3D array synthetic aperture radar (array-SAR), known for its high-quality, flexible measure ments. Recent advancements in sparsity-based imaging methods have addressed traditional method limitations like limited resolution and interferences. However, they present new challenges, like limited generalization ability due to the requirement for manual hyperparameter adjustment for different targets, and reduced performance in low sampling conditions. To overcome these challenges, we propose a new imaging method based on deep learning. This method features three main features: First, it is based on a generative model where the imaging result is generated through a latent variable, which achieves higher imaging quality in low sampling scenarios. Second, it is unsupervised, leveraging the system's physical measurement model to enhance its resilience against various targets and measurement scenarios, thereby increasing generalization. Third, it is model driven, not end-to-end (E2E). The generation process is guided by the system's physical measurement model and the classical target sparsity prior, adhering to the principles of Bayesian estimation, which improves its interpretability. In experiments, our method outperformed other known methods in accuracy and target structure preservation. It remained robust under extreme conditions like 10% sampling, where others failed, and required minimal manual hyperparameter tuning.
更多查看译文
关键词
scattering diagnosis,3D SAR,unsupervised learning,generative model,model-driven
AI 理解论文
溯源树
样例
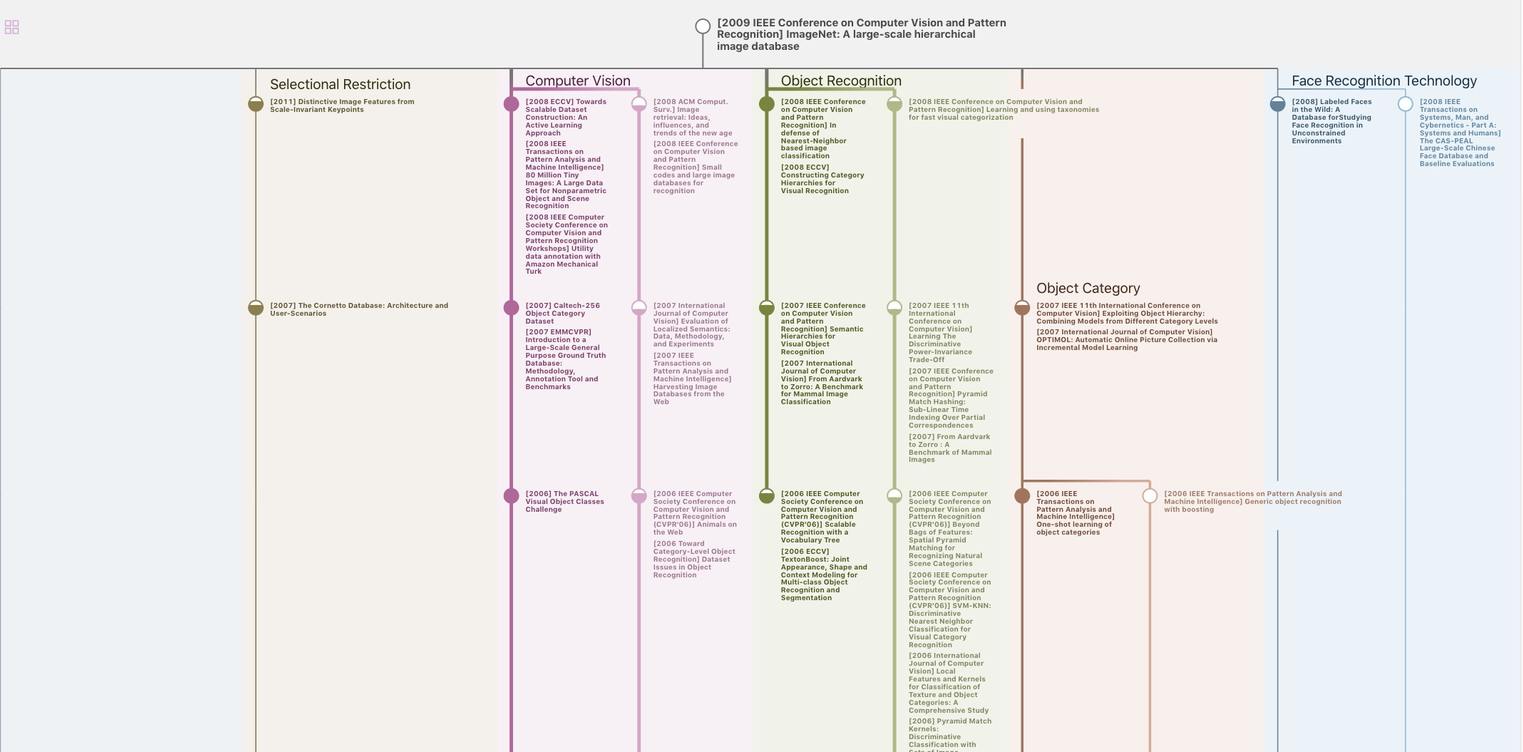
生成溯源树,研究论文发展脉络
Chat Paper
正在生成论文摘要