Estimation of Time-to-Total Knee Replacement Surgery
arxiv(2024)
摘要
A survival analysis model for predicting time-to-total knee replacement (TKR)
was developed using features from medical images and clinical measurements.
Supervised and self-supervised deep learning approaches were utilized to
extract features from radiographs and magnetic resonance images. Extracted
features were combined with clinical and image assessments for survival
analysis using random survival forests. The proposed model demonstrated high
discrimination power by combining deep learning features and clinical and image
assessments using a fusion of multiple modalities. The model achieved an
accuracy of 75.6
surgery. Accurate time-to-TKR predictions have the potential to help assist
physicians to personalize treatment strategies and improve patient outcomes.
更多查看译文
AI 理解论文
溯源树
样例
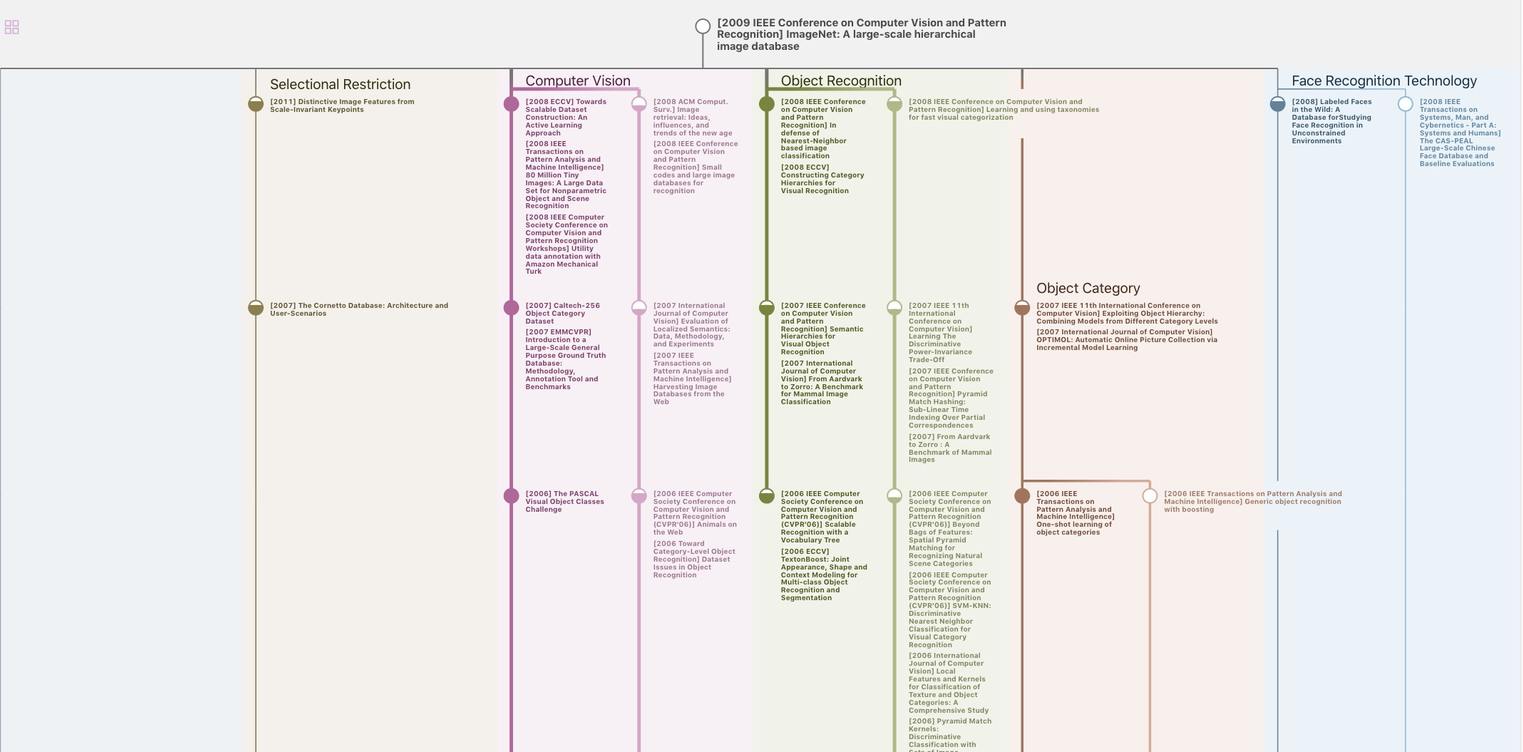
生成溯源树,研究论文发展脉络
Chat Paper
正在生成论文摘要