Constraining the giant radio galaxy population with machine learning and Bayesian inference
arxiv(2024)
摘要
Large-scale sky surveys at low frequencies, like the LOFAR Two-metre Sky
Survey (LoTSS), allow for the detection and characterisation of unprecedented
numbers of giant radio galaxies (GRGs, or 'giants'). In this work, by
automating the creation of radio–optical catalogues, we aim to significantly
expand the census of known giants. We then combine this sample with a forward
model to constrain GRG properties of cosmological interest. In particular, we
automate radio source component association through machine learning and
optical host identification for resolved radio sources. We create a
radio–optical catalogue for the full LoTSS Data Release 2 (DR2) and select all
possible giants. We combine our candidates with an existing catalogue of LoTSS
DR2 crowd-sourced GRG candidates and visually confirm or reject them. To infer
intrinsic GRG properties from GRG observations, we develop further a
population-based forward model that takes into account selection effects and
constrain its parameters using Bayesian inference. We confirm 5,647 previously
unknown giants from the crowd-sourced catalogue and 2,597 previously unknown
giants from the ML-driven catalogue. Our confirmations and discoveries bring
the total number of known giants to at least 11,585. We predict a comoving GRG
number density n_GRG = 13 ± 10 (100 Mpc)^-3, close to
a recent estimate of the number density of luminous non-giant radio galaxies.
We derive a current-day GRG lobe volume-filling fraction V_GRG-CW(z =
0) = 1.4 ± 1.1 · 10^-5 in clusters and filaments of the Cosmic Web.
Our analysis suggests that giants are more common than previously thought.
Moreover, tentative results imply that it is possible that magnetic fields once
contained in giants pervade a significant (≳ 10%) fraction of today's
Cosmic Web.
更多查看译文
AI 理解论文
溯源树
样例
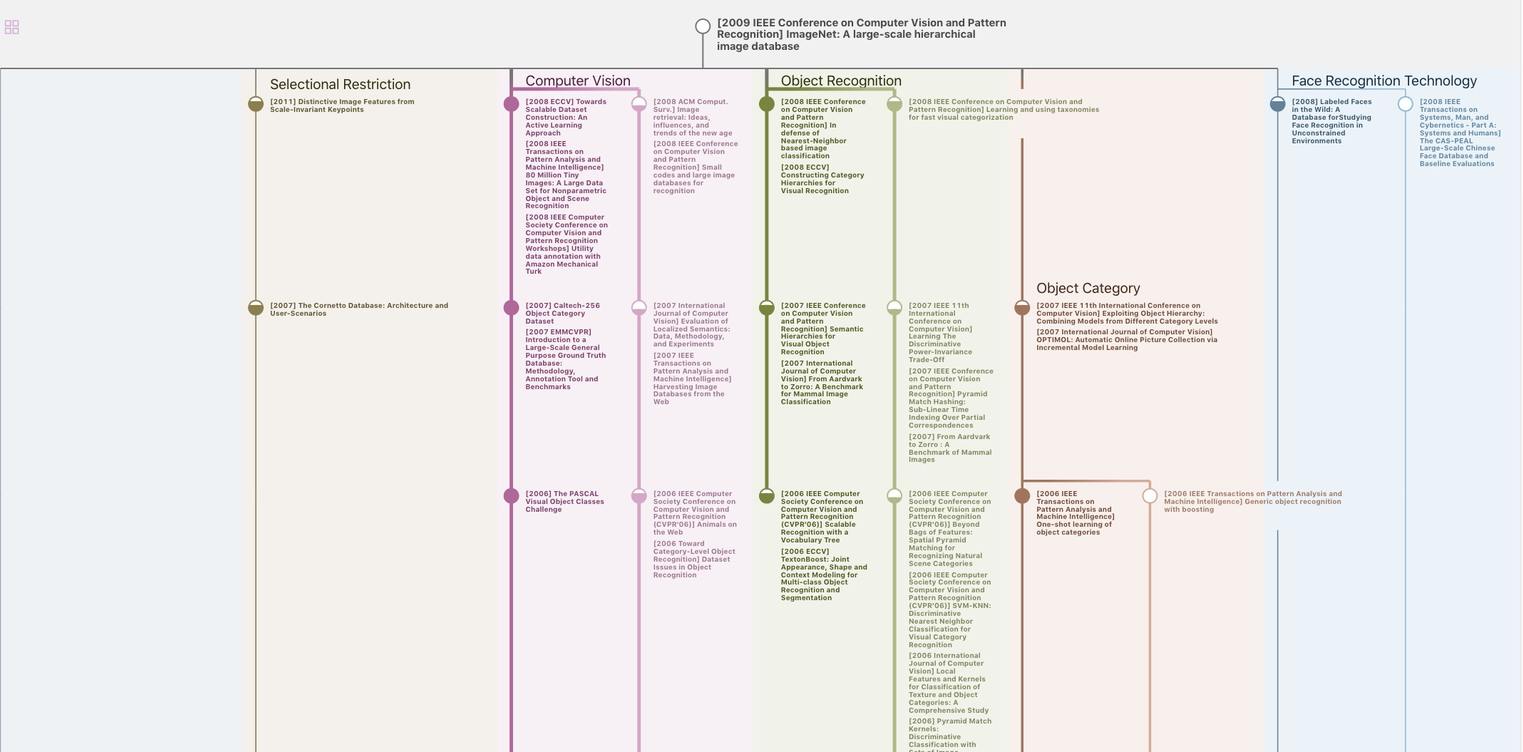
生成溯源树,研究论文发展脉络
Chat Paper
正在生成论文摘要