LEAP: Optimization Hierarchical Federated Learning on Non-IID Data with Coalition Formation Game
IJCAI 2024(2024)
摘要
Although Hierarchical Federated Learning (HFL) utilizes edge servers (ESs) toalleviate communication burdens, its model performance will be degraded bynon-IID data and limited communication resources. Current works often assumethat data is uniformly distributed, which however contradicts the heterogeneityof IoT. Solutions of additional model training to check the data distributioninevitably increases computational costs and the risk of privacy leakage. Thechallenges in solving these issues are how to reduce the impact of non-IID datawithout involving raw data and how to rationalize the communication resourceallocation for addressing straggler problem. To tackle these challenges, wepropose a novel optimization method based on coaLition formation gamE andgrAdient Projection, called LEAP. Specifically, we combine edge datadistribution with coalition formation game innovatively to adjust thecorrelations between clients and ESs dynamically, which ensures optimalcorrelations. We further capture the client heterogeneity to achieve therational bandwidth allocation from coalition perception and determine theoptimal transmission power within specified delay constraints at client level.Experimental results on four real datasets show that LEAP is able to achieve20.62baselines. Moreover, LEAP effectively reduce transmission energy consumption byat least about 2.24 times.
更多查看译文
关键词
Machine Learning -> ML: Evaluation,Agent-based and Multi-agent Systems -> MAS: Resource allocation,Game Theory and Economic Paradigms -> GTEP: Mechanism design,Machine Learning -> ML: Game Theory
AI 理解论文
溯源树
样例
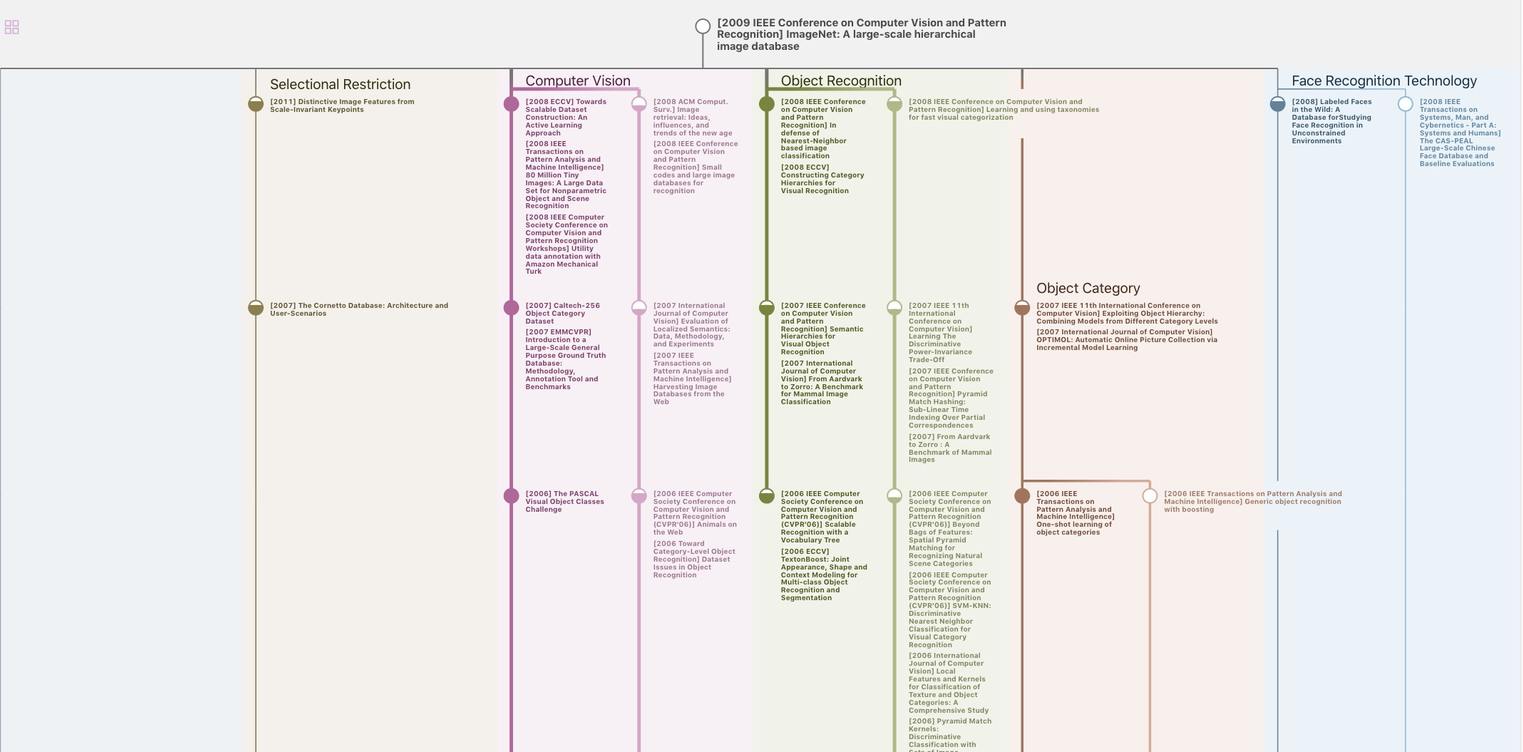
生成溯源树,研究论文发展脉络
Chat Paper
正在生成论文摘要