HMA-SAR: Multi-Agent Search and Rescue for Unknown Located Dynamic Targets in Completely Unknown Environments
IEEE Robotics and Automation Letters(2024)
摘要
Multi-Agent Search and Rescue (MASAR) tasks, challenged by unknown environments and the unpredictable movements of unknown dynamic targets, suffer from inefficiencies in traditional map coverage techniques which require repeated sweeps. Addressing this, our study introduces a novel MASAR framework based on Multi-Agent Reinforcement Learning (MARL), featuring innovative elements like state, reward, and network structure design, alongside a Heterogeneous Curriculum Training algorithm and a hybrid decision mechanism. These components collectively enhance performance in dynamic environments, improve model generalization, and mitigate issues like sparse rewards and policy bias. In grid map simulations, our approach, HMA-SAR (Heterogeneous Multi-Agent Search and Rescue Framework), demonstrated consistent superiority over the traditional frontier-based method and other MARL algorithms, in metrics such as success rate, steps count, and the number of targets fetched. The practical applicability of our approach was further validated through experiments in Gazebo and real-world scenarios. Additionally, scalability tests in grid maps revealed substantial improvements in success rates and task completion times with increased agent deployment.
更多查看译文
关键词
Cooperative Multi-Agent Search and Rescue (MASAR),Dynamic Targets,Multi-Agent Reinforcement Learning,Heterogeneous Curriculum Training Algorithm,Hybrid Decision Mechanism
AI 理解论文
溯源树
样例
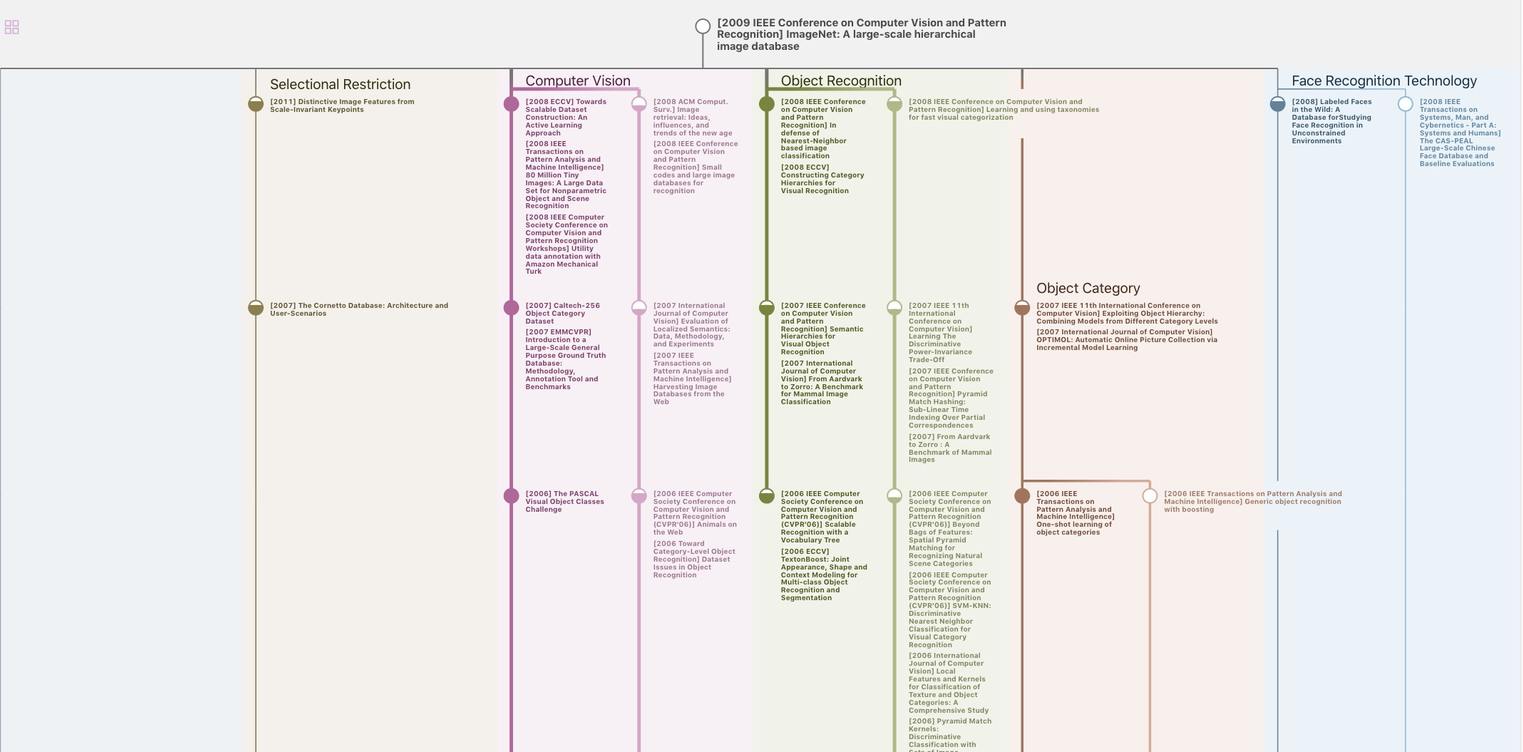
生成溯源树,研究论文发展脉络
Chat Paper
正在生成论文摘要