Prompt Engineering Strategies Improve the Diagnostic Accuracy of GPT-4 Turbo in Neuroradiology Cases
medrxiv(2024)
摘要
Background: Large language models (LLMs) like GPT-4 demonstrate promising capabilities in medical image analysis, but their practical utility is hindered by substantial misdiagnosis rates ranging from 30-50%. Purpose: To improve the diagnostic accuracy of GPT-4 Turbo in neuroradiology cases using prompt engineering strategies, thereby reducing misdiagnosis rates. Materials and Methods: We employed 751 publicly available neuroradiology cases from the American Journal of Neuroradiology Case of the Week Archives. Prompt instructions guided GPT-4 Turbo to analyze clinical and imaging data, generating a list of five candidate diagnoses with confidence levels. Strategies included role adoption as an imaging expert, step-by-step reasoning, and confidence assessment. Results: Without any adjustments, the baseline accuracy of GPT-4 Turbo was 55.1% to correctly identify the top diagnosis, with a misdiagnosis rate of 29.4%. Considering the five candidates' improved applicability, it is 70.6%. Applying a 90% confidence threshold increased the accuracy of the top diagnosis to 72.9% and the applicability of the five candidates to 85.9%, while reducing misdiagnoses to 14.1%, but limited the analysis to half of cases. Conclusion: Prompt engineering strategies with confidence level thresholds demonstrated the potential to reduce misdiagnosis rates in neuroradiology cases analyzed by GPT-4 Turbo. This research paves the way for enhancing the feasibility of AI-assisted diagnostic imaging, where AI suggestions can contribute to human decision-making processes. However, the study lacks analysis of real-world clinical data. This highlights the need for further investigation in various specialties and medical modalities to optimize thresholds that balance diagnostic accuracy and practical utility.
### Competing Interest Statement
The authors have declared no competing interest.
### Funding Statement
This work was supported by the Japan Society for the Promotion of Science (JSPS) KAKENHI under Grant [22K07674]. The authors would like to express their gratitude for the financial support provided, which has been instrumental in the advancement of this research.
### Author Declarations
I confirm all relevant ethical guidelines have been followed, and any necessary IRB and/or ethics committee approvals have been obtained.
Yes
I confirm that all necessary patient/participant consent has been obtained and the appropriate institutional forms have been archived, and that any patient/participant/sample identifiers included were not known to anyone (e.g., hospital staff, patients or participants themselves) outside the research group so cannot be used to identify individuals.
Yes
I understand that all clinical trials and any other prospective interventional studies must be registered with an ICMJE-approved registry, such as ClinicalTrials.gov. I confirm that any such study reported in the manuscript has been registered and the trial registration ID is provided (note: if posting a prospective study registered retrospectively, please provide a statement in the trial ID field explaining why the study was not registered in advance).
Yes
I have followed all appropriate research reporting guidelines, such as any relevant EQUATOR Network research reporting checklist(s) and other pertinent material, if applicable.
Yes
All data produced in the present study are available upon reasonable request to the authors
更多查看译文
AI 理解论文
溯源树
样例
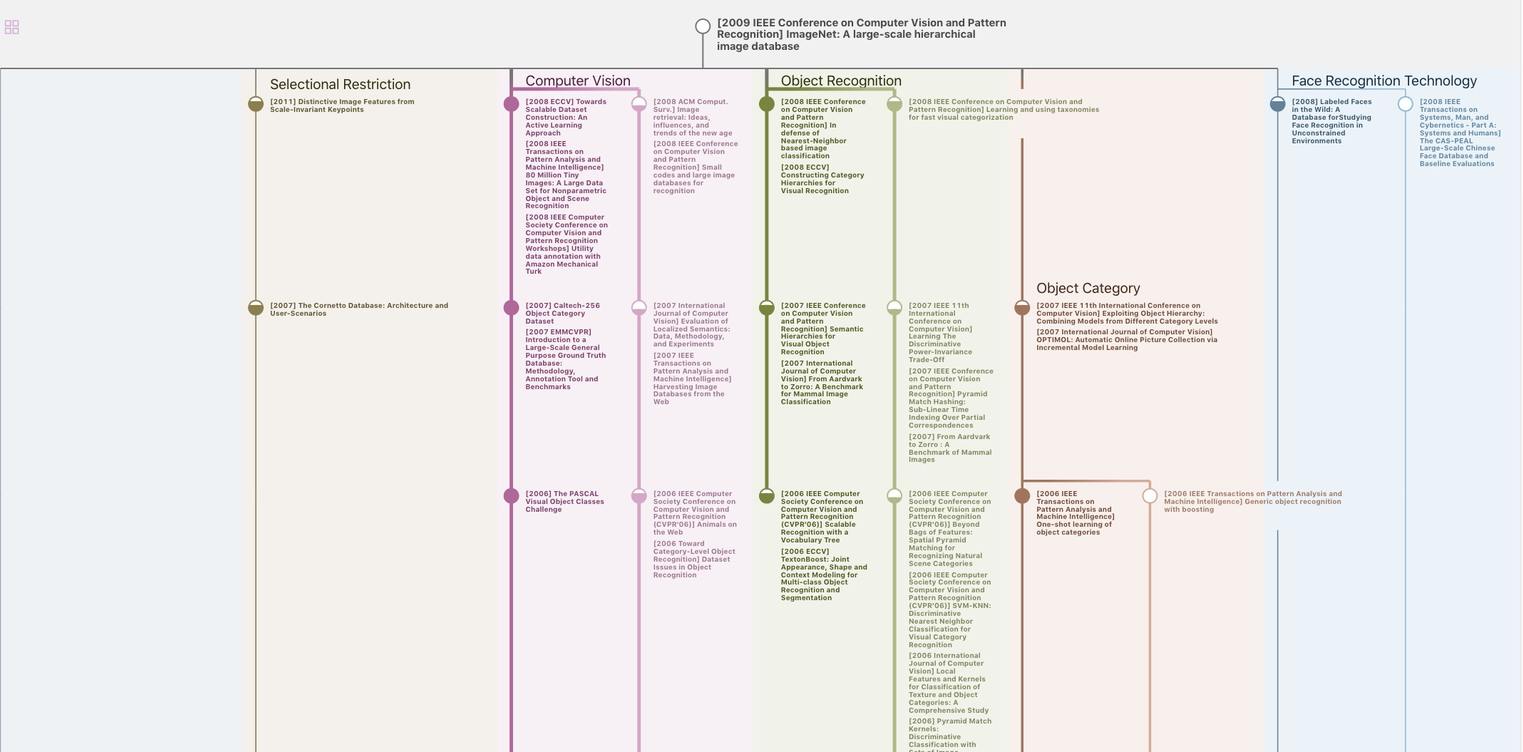
生成溯源树,研究论文发展脉络
Chat Paper
正在生成论文摘要