Performance evaluation of 2D LiDAR SLAM algorithms in simulated orchard environments
Computers and Electronics in Agriculture(2024)
摘要
Accurate localization is a prerequisite for developing autonomous mobile orchard robots. Simultaneous localization and mapping (SLAM) is an efficient technique for localizing robots in global navigation satellite system (GNSS)-denied scenarios. Light detection and ranging (LiDAR) is one of the most crucial sensors used for environment perception. Two-dimensional (2D) LiDAR SLAM has been successfully applied in various indoor scenes to construct flat maps and estimate robot trajectories. In this paper, the performances of three representative 2D LiDAR SLAM algorithms, namely, Hector, GMapping, and Cartographer, in semi-structured orchard environments simulated in Gazebo are analysed. A hierarchical terrain modelling method is proposed to generate scalable orchard terrain with adjustable roughness. The adaptability of the three algorithms to terrain roughness, LiDAR, and orchard size is evaluated in terms of the localization error, mapping error, CPU usage, and memory usage. The experimental results show that Cartographer has the highest location and mapping accuracy, followed by GMapping and Hector. However, Hector requires the least computational resources, followed by Cartographer and GMapping. In a 50 m × 50 m orchard with an elevation difference of 15 cm, Cartographer achieved a localization error of 8.14 cm and a mapping error of 8.43 cm at a 4 cm map resolution. In addition, Hector has the highest requirements for the maximum range and field-of-view (FOV) of LiDAR, and GMapping is most susceptible to severe uneven terrain conditions and has the worst scalability for large-scale orchards.
更多查看译文
关键词
2D LiDAR SLAM,Orchard SLAM,Hector,GMapping,Cartographer,Gazebo,Terrain model
AI 理解论文
溯源树
样例
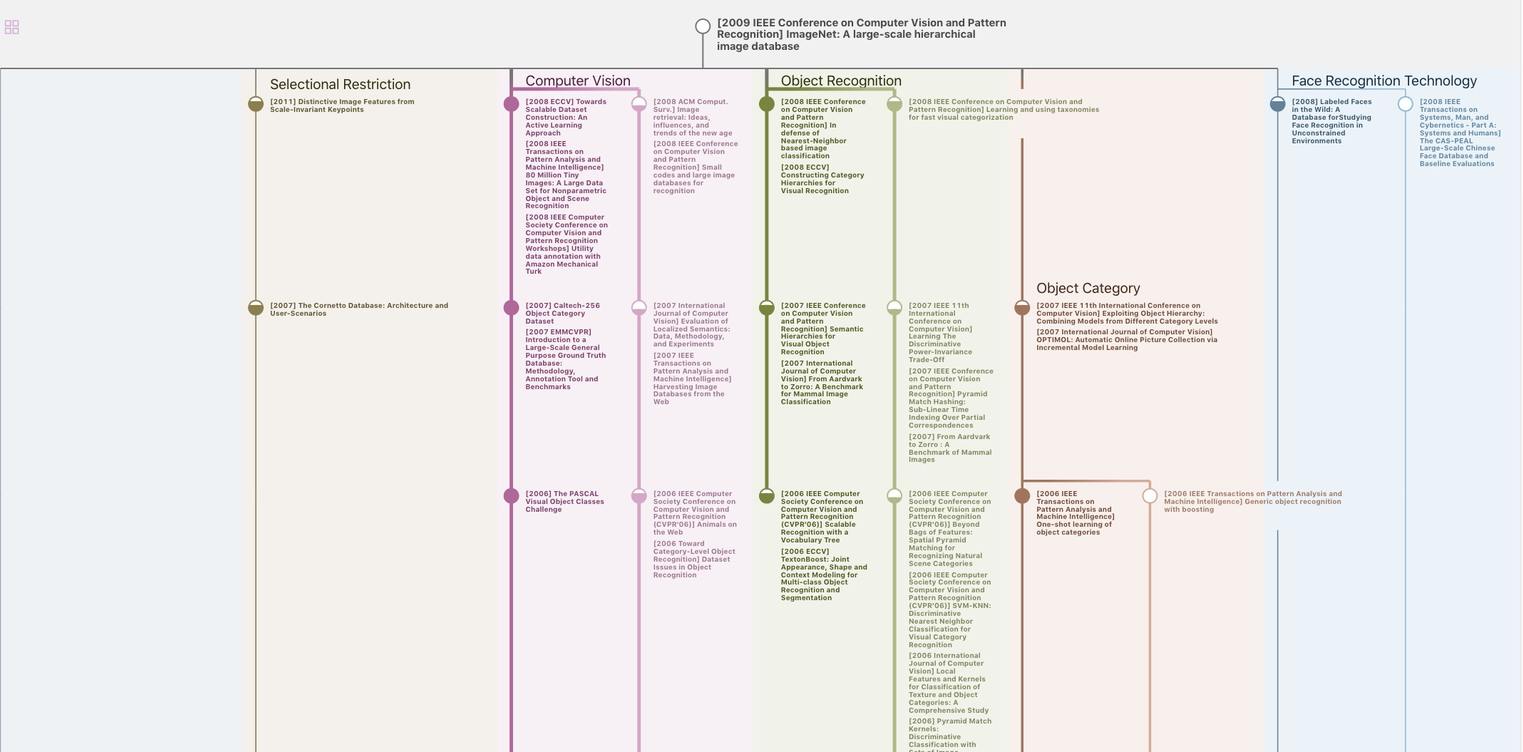
生成溯源树,研究论文发展脉络
Chat Paper
正在生成论文摘要