Developing a Machine-Learning Predictive Model for Retention of Posterior Cruciate Ligament in Patients Undergoing Total Knee Arthroplasty.
Orthopaedic surgery(2024)
摘要
OBJECTIVE:Predicting whether the posterior cruciate ligament (PCL) should be preserved during total knee arthroplasty (TKA) procedures is a complex task in the preoperative phase. The choice to either retain or excise the PCL has a substantial effect on the surgical outcomes and biomechanical integrity of the knee joint after the operation. To enhance surgeons' ability to predict the removal and retention of the PCL in patients before TKA, we developed machine learning models. We also identified significant feature factors that contribute to accurate predictions during this process.
METHODS:Patients' data on TKA continuously performed by a single surgeon who had intended initially to undergo implantation of cruciate-retaining (CR) prostheses was collected. During the sacrifice of PCL, we utilized anterior-stabilized (AS) tibial bearings. The dataset was split into CR and AS categories to form distinct groups. Relevant information regarding age, gender, body mass index (BMI), the affected side, and preoperative diagnosis was extracted by reviewing the medical records of the patients. To ensure the authenticity of the research, an initial step involved capturing X-ray images before the surgery. These images were then analyzed to determine the height of the medial condyle (MMH) and lateral condyle (LMH), as well as the ratios between MLW and MMH and MLW and LMH. Additionally, the insall-salvati index (ISI) was calculated, and the severity of any varus or valgus deformities was assessed. Eight machine-learning methods were developed to predict the retention of PCL in TKA. Risk factor analysis was performed using the SHApley Additive exPlanations method.
RESULTS:A total of 307 knee joints from 266 patients were included, among which there were 254 females and 53 males. A stratified random sampling technique was used to split patients in a 70:30 ratio into a training dataset and a testing dataset. Eight machine-learning models were trained using data feeding. Except for the AUC of the LGBM Classifier, which is 0.70, the AUCs of other machine learning models are all lower than 0.70. In importance-based analysis, ISI, MMH, LMH, deformity, and age were confirmed as important predictive factors for PCL retention in operations.
CONCLUSION:The LGBM Classifier model achieved the best performance in predicting PCL retention in TKA. Among the potential risk factors, ISI, MMH, LMH, and deformity played essential roles in the prediction of PCL retention.
更多查看译文
AI 理解论文
溯源树
样例
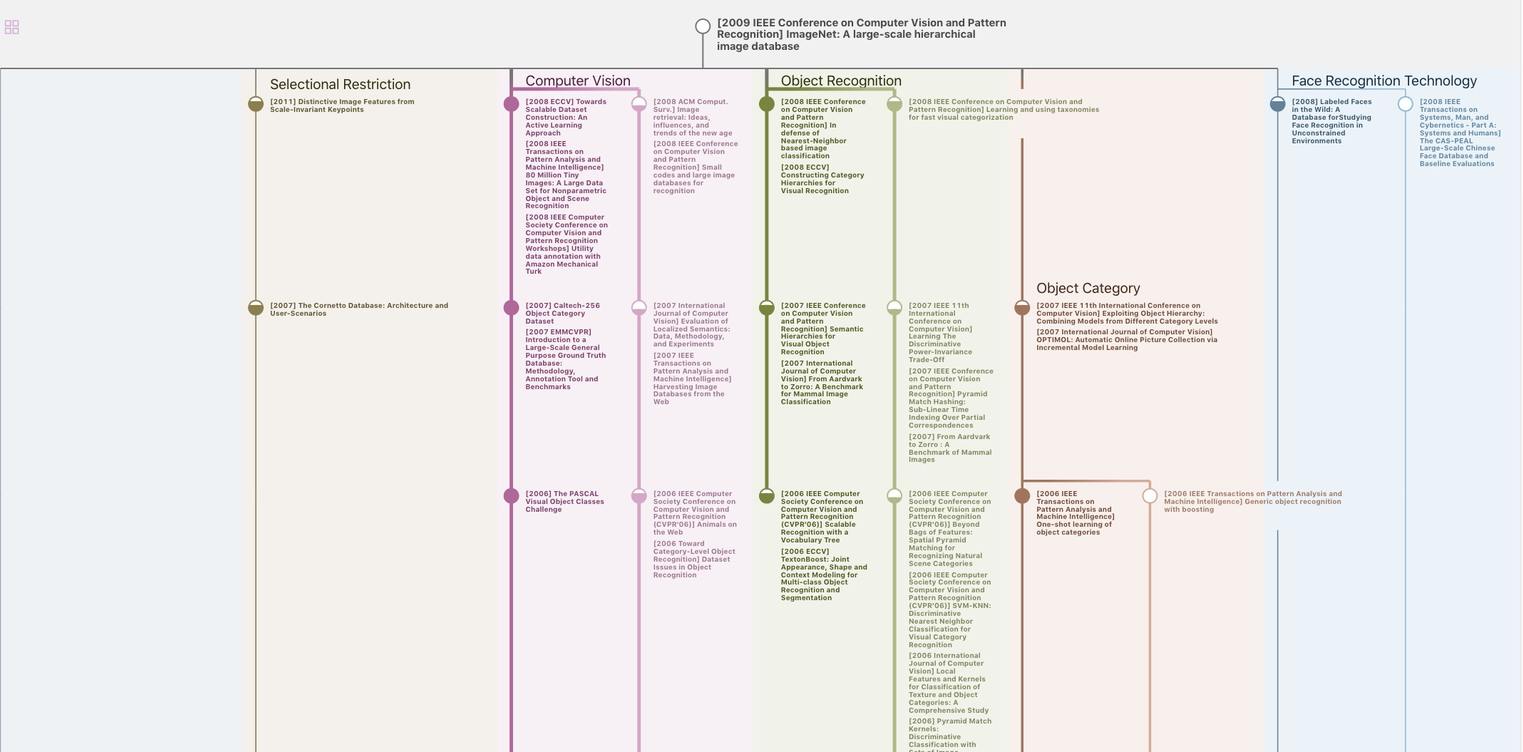
生成溯源树,研究论文发展脉络
Chat Paper
正在生成论文摘要