The Noisy Encoding of Disparity Model Predicts Perception of the McGurk Effect in Native Japanese Speakers
crossref(2024)
摘要
The McGurk effect is an illusion that demonstrates the influence of information from the face of the talker on the perception of auditory speech. The diversity of human languages has prompted many intercultural studies of the effect, including in native Japanese speakers. Studies of large samples of native English speakers have shown that the McGurk effect is characterized by high variability, both in the susceptibility of different individuals to the illusion and in the frequency with which different experimental stimuli induce the illusion. The noisy encoding of disparity (NED) model of the McGurk effect uses Bayesian principles to account for this variability by separately estimating the susceptibility and sensory noise for each individual and the strength of each stimulus. To test whether the NED model could account for McGurk perception in a non-Western culture, we applied it to data collected from 80 native Japanese-speaking participants. Fifteen different McGurk stimuli were presented, along with audiovisual congruent stimuli. The McGurk effect was highly variable across stimuli and participants, with the percentage of illusory fusion responses ranging from 3% to 78% across stimuli and from 0% to 91% across participants. Despite this variability, the NED model accurately predicted perception, predicting fusion rates for individual stimuli with 2.1% error and for individual participants with 2.4% error. Stimuli containing the unvoiced pa/ka pairing evoked more fusion responses than the voiced ba/ga pairing. Model estimates of sensory noise was correlated with participant age, with greater sensory noise in older participants. The NED model of the McGurk effect offers a principled way to account for individual and stimulus differences when examining the McGurk effect within and across cultures.
### Competing Interest Statement
The authors have declared no competing interest.
更多查看译文
AI 理解论文
溯源树
样例
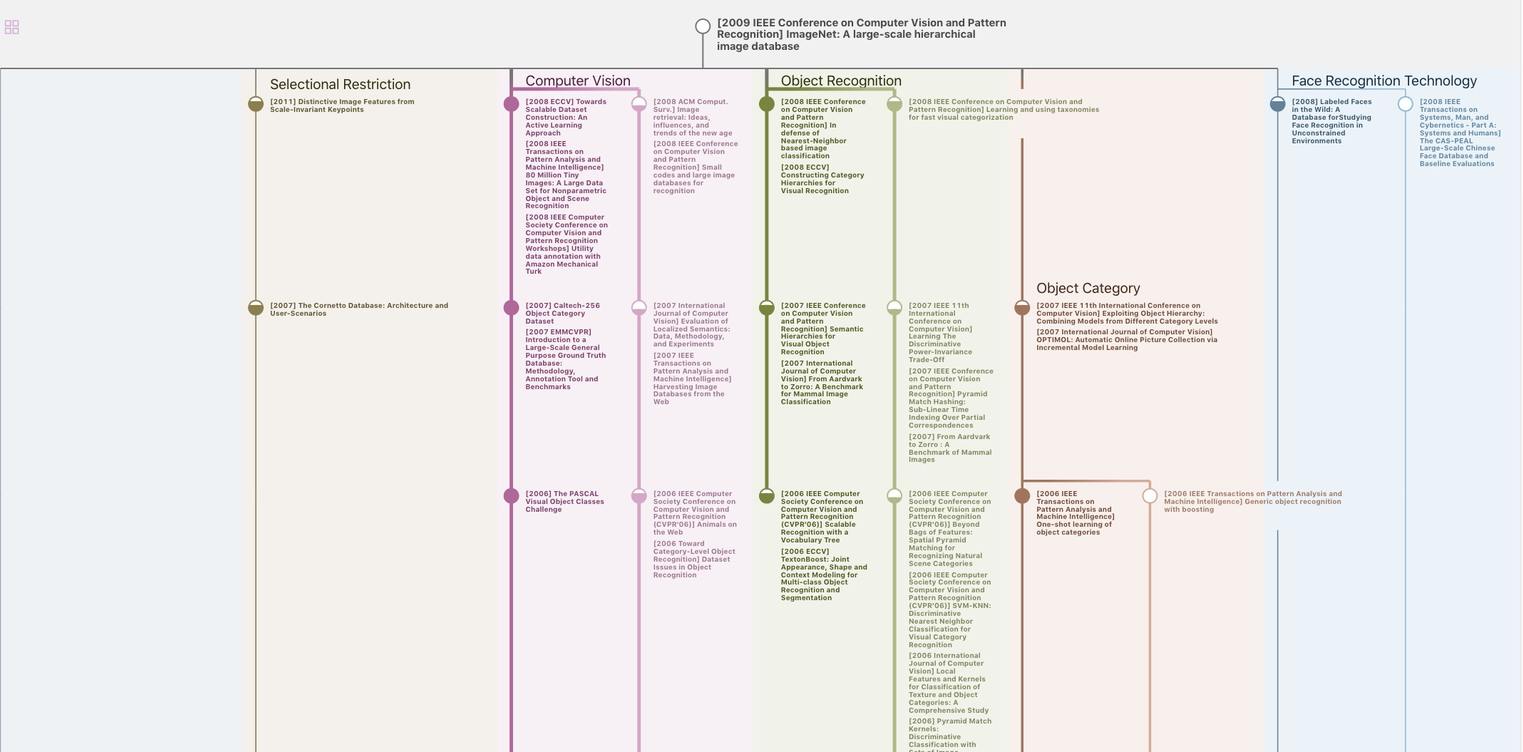
生成溯源树,研究论文发展脉络
Chat Paper
正在生成论文摘要