A Nested Deep Learning Framework for the Steady-State Modeling of Power Converters in Time Domain
2024 IEEE Applied Power Electronics Conference and Exposition (APEC)(2024)
摘要
Artificial-intelligence-based data-driven methods have attracted widespread attention in the areas of power electronics modeling, attributable to their merits of the capability of automatically growing intelligence from data, easy scalability for different applications, plug-and-play feature for various scenarios, inherent protection of intellectual property from different manufactures, etc. For the time-domain modeling of power converters in steady state, existing data-driven methods configure them as time-series prediction tasks using recurrent structures like long-short-term-memory network, exhibiting nontrivial challenges. First, how to provide the initial state values for the recurrent structure is the main concern. Second, how to model cyclic waveforms in steady state requires investigation. Third, the gradient vanishing and explosion issues should be handled properly. Aiming at these challenges, this paper presents the "NestT", a nested (artificial intelligence) AI framework for the steady-state modeling of power converters in the time domain. NestT utilizes an extreme gradient boosting for initial status estimation and a gated-recurrent-unit net with layer normalization for temporal modeling. Synchronization and reset processes are included to improve training and enable cyclic modeling. The proposed NestT framework is validated through 1-kW hardware experiments. NestT strives to pave the way for increasing the penetration of AI in the modeling of power converters.
更多查看译文
关键词
artificial intelligence,deep learning,modeling,steady-state,time-series prediction
AI 理解论文
溯源树
样例
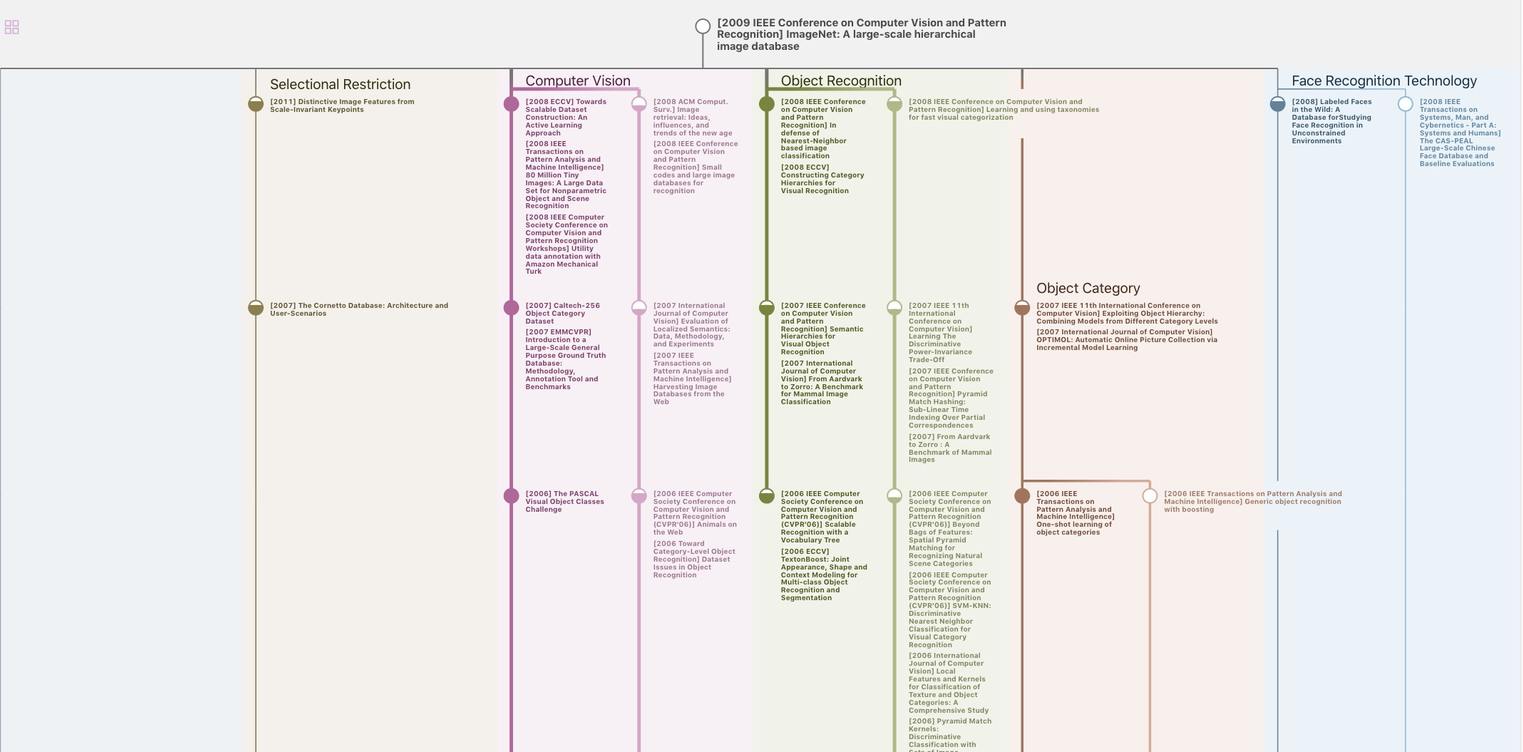
生成溯源树,研究论文发展脉络
Chat Paper
正在生成论文摘要