Distributed No-regret Learning in Aggregative Games with Residual Bandit Feedback
IEEE Transactions on Control of Network Systems(2024)
摘要
This paper investigates distributed no-regret learning in repeated aggregative games with bandit feedback. The players lack an explicit model of the game and can only learn their actions based on the sole available feedback of payoff values. Additionally, they cannot directly access the aggregate term that contains global information, while each player shares information with its neighbors without revealing its own strategy. We present a novel no-regret learning algorithm named Distributed Online Gradient Descent with Residual Bandit (DOGD-ResiBan). In the algorithm, each player maintains a local estimate of the aggregate and adaptively adjusts its next action through the residual bandit mechanism and the online gradient descent method. We first provide regret analysis for aggregative games where the player-specific problem is convex, showing crucial associations between the regret bound, network connectivity, and game structure. Then, we prove that when the game is also strictly monotone, the action sequence generated by the algorithm converges to the Nash equilibrium almost surely. Finally, we demonstrate the algorithm performance through numerical simulations on the Cournot game.
更多查看译文
关键词
Online learning,no-regret learning,distributed algorithms,aggregative games,bandit feedback
AI 理解论文
溯源树
样例
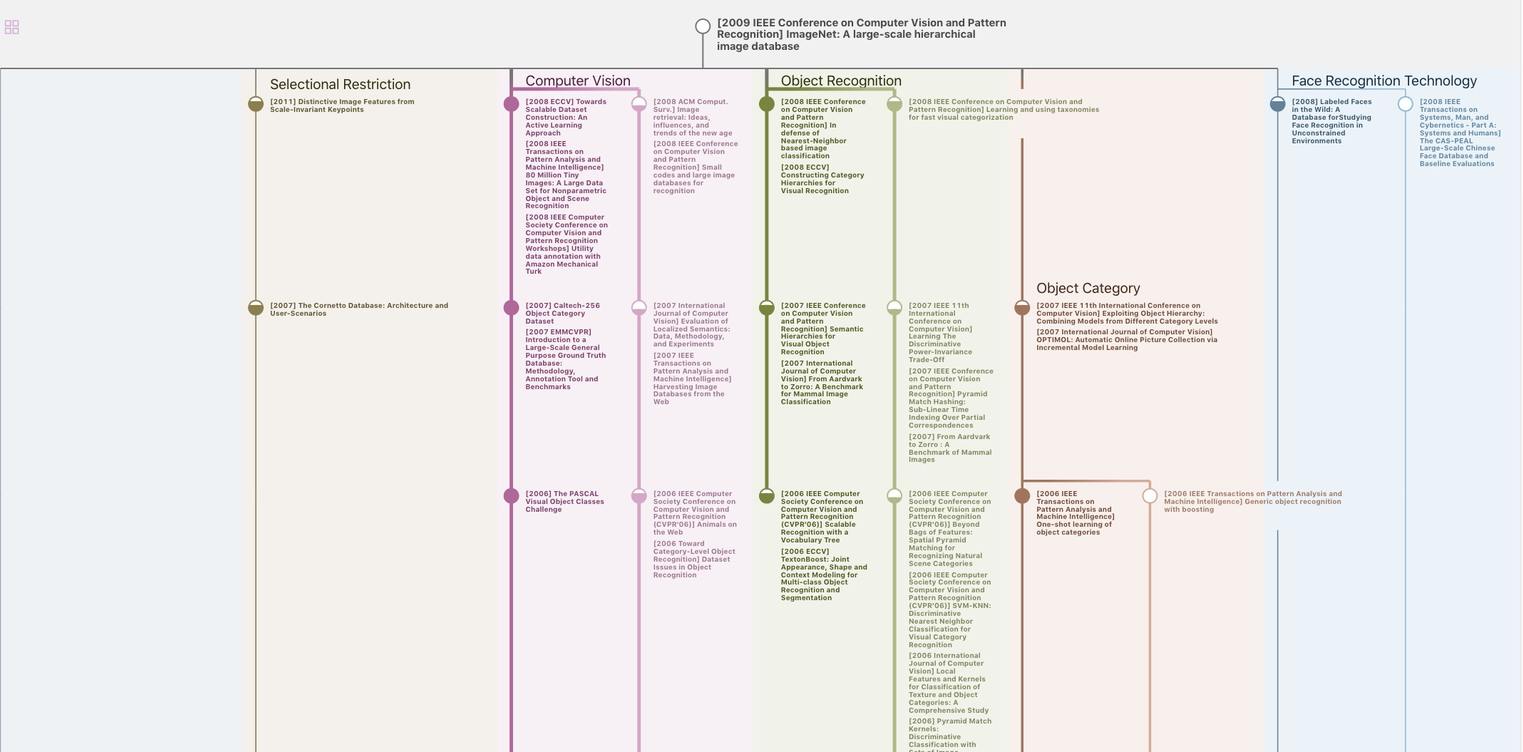
生成溯源树,研究论文发展脉络
Chat Paper
正在生成论文摘要