Myocardial infarction detection method based on the continuous T-wave area feature and multi-lead-fusion deep features.
Physiological measurement(2024)
摘要
OBJECTIVE:Myocardial infarction (MI) is one of the most threatening cardiovascular diseases. This paper aims to explore a method for using an algorithm to autonomously classify myocardial infarction based on the electrocardiogram (ECG).
APPROACH:A detection method of MI that fuses continuous T-wave area (C_TWA) feature and ECG deep features is proposed. This method consists of three main parts: (1) The onset of MI is often accompanied by changes in the shape of the T-wave in the ECG, thus the area of the T-wave displayed on different heartbeats will be quite different. The adaptive sliding window method is used to detect the start and end of the T-wave, and calculate the C_TWA on the same ECG record. Additionally, the coefficient of variation (CV) of C_TWA is defined as the C_TWA feature of the ECG. (2) The multi lead fusion convolutional neural network (Multi-lead-fusion CNN) was implemented to extract the deep features of the ECG. (3) The C_TWA feature and deep features of the ECG were fused by soft attention, and then inputted into the multi-layer perceptron to obtain the detection result.
RESULTS:According to the interpatient paradigm, the proposed method reached a 97.67% accuracy, 96.59% precision, and 98.96% recall on the PTB dataset, whereas the proposed method reached 93.15% accuracy, 93.20% precision, and 95.14% recall on the clinical dataset.
SIGNIFICANCE:The proposed method accurately extracts the feature of the C_TWA, and combines the deep features of the signal, thereby improving the detection accuracy and achieving ideal results on clinical datasets.
更多查看译文
AI 理解论文
溯源树
样例
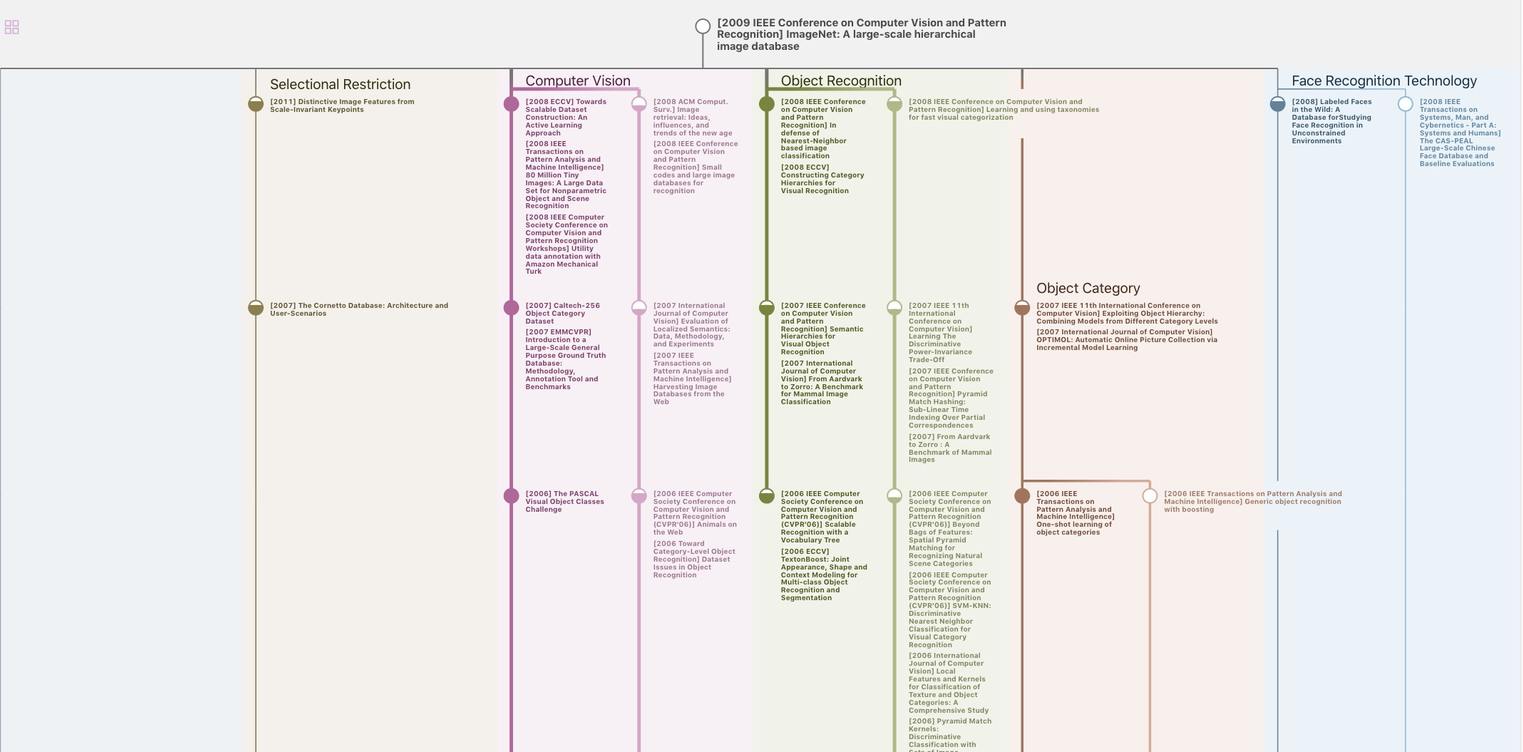
生成溯源树,研究论文发展脉络
Chat Paper
正在生成论文摘要