WHALE-FL: Wireless and Heterogeneity Aware Latency Efficient Federated Learning over Mobile Devices via Adaptive Subnetwork Scheduling
arxiv(2024)
摘要
As a popular distributed learning paradigm, federated learning (FL) over
mobile devices fosters numerous applications, while their practical deployment
is hindered by participating devices' computing and communication
heterogeneity. Some pioneering research efforts proposed to extract subnetworks
from the global model, and assign as large a subnetwork as possible to the
device for local training based on its full computing and communications
capacity. Although such fixed size subnetwork assignment enables FL training
over heterogeneous mobile devices, it is unaware of (i) the dynamic changes of
devices' communication and computing conditions and (ii) FL training progress
and its dynamic requirements of local training contributions, both of which may
cause very long FL training delay. Motivated by those dynamics, in this paper,
we develop a wireless and heterogeneity aware latency efficient FL (WHALE-FL)
approach to accelerate FL training through adaptive subnetwork scheduling.
Instead of sticking to the fixed size subnetwork, WHALE-FL introduces a novel
subnetwork selection utility function to capture device and FL training
dynamics, and guides the mobile device to adaptively select the subnetwork size
for local training based on (a) its computing and communication capacity, (b)
its dynamic computing and/or communication conditions, and (c) FL training
status and its corresponding requirements for local training contributions. Our
evaluation shows that, compared with peer designs, WHALE-FL effectively
accelerates FL training without sacrificing learning accuracy.
更多查看译文
AI 理解论文
溯源树
样例
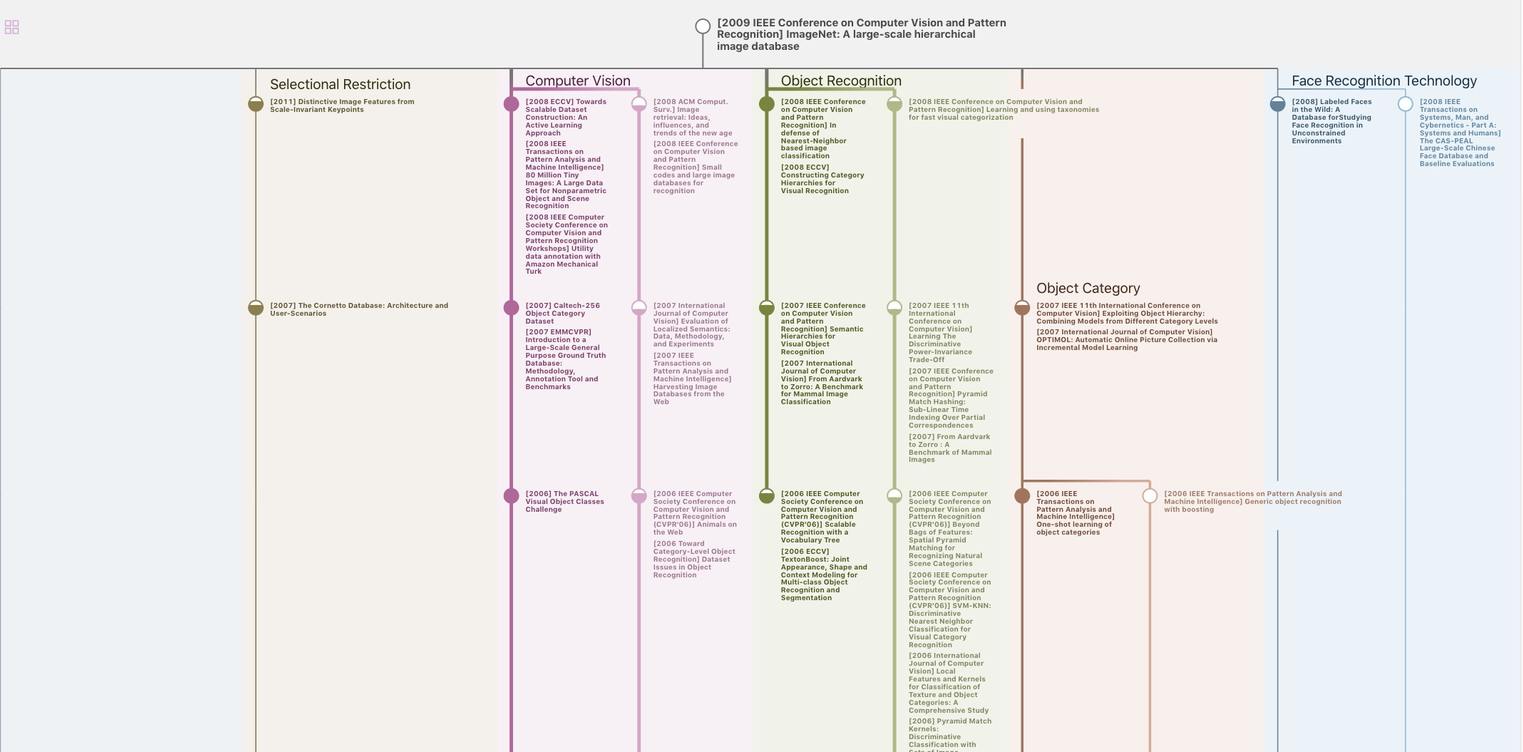
生成溯源树,研究论文发展脉络
Chat Paper
正在生成论文摘要