Efficient Data Generation for Source-grounded Information-seeking Dialogs: A Use Case for Meeting Transcripts
CoRR(2024)
摘要
Existing methods for creating source-grounded information-seeking dialog
datasets are often costly and hard to implement due to their sole reliance on
human annotators. We propose combining large language models (LLMs) prompting
with human expertise for more efficient and reliable data generation. Instead
of the labor-intensive Wizard-of-Oz (WOZ) method, where two annotators generate
a dialog from scratch, role-playing agent and user, we use LLM generation to
simulate the two roles. Annotators then verify the output and augment it with
attribution data. We demonstrate our method by constructing MISeD – Meeting
Information Seeking Dialogs dataset – the first information-seeking dialog
dataset focused on meeting transcripts. Models finetuned with MISeD demonstrate
superior performance on our test set, as well as on a novel fully-manual WOZ
test set and an existing query-based summarization benchmark, suggesting the
utility of our approach.
更多查看译文
AI 理解论文
溯源树
样例
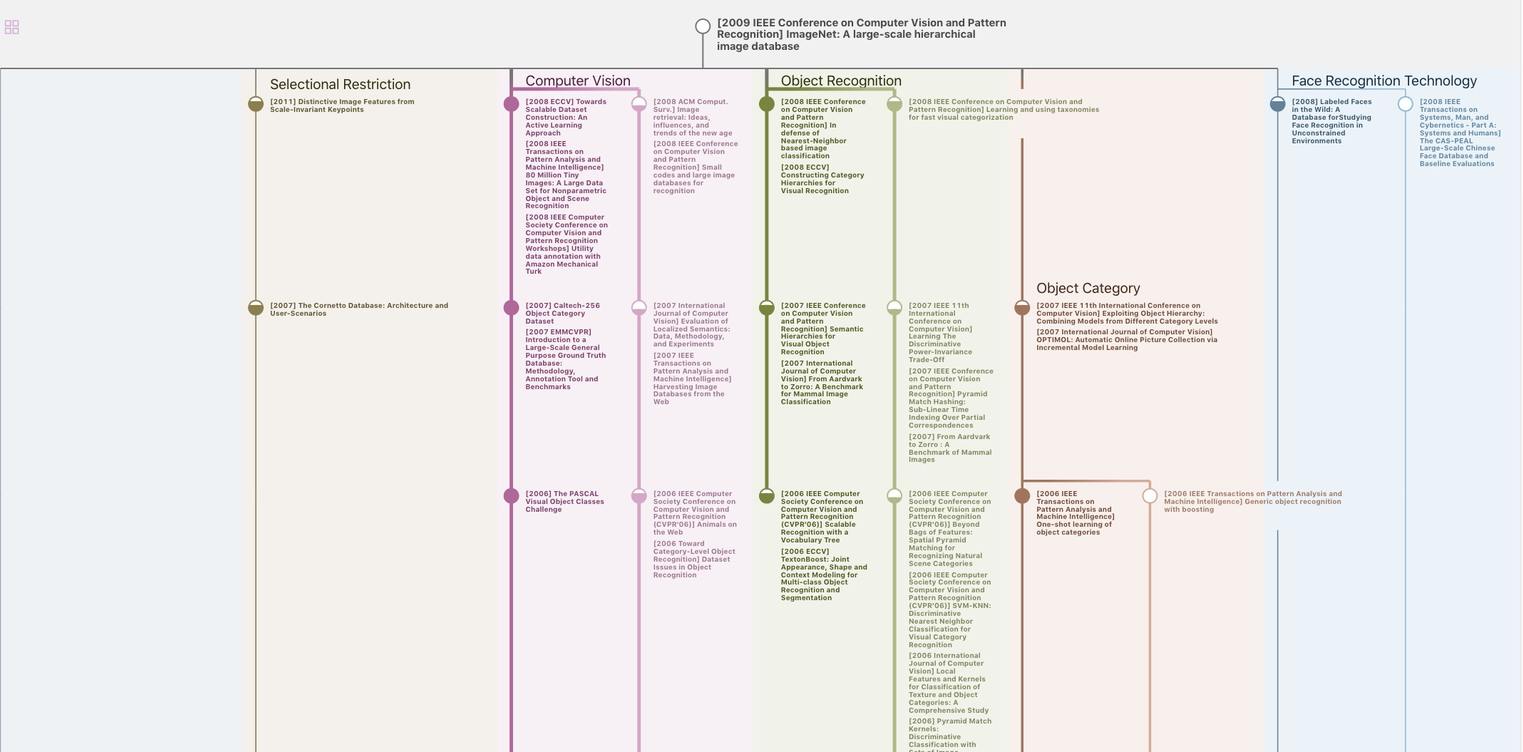
生成溯源树,研究论文发展脉络
Chat Paper
正在生成论文摘要