Variable Selection in Ultra-high Dimensional Feature Space for the Cox Model with Interval-Censored Data
arxiv(2024)
摘要
We develop a set of variable selection methods for the Cox model under
interval censoring, in the ultra-high dimensional setting where the
dimensionality can grow exponentially with the sample size. The methods select
covariates via a penalized nonparametric maximum likelihood estimation with
some popular penalty functions, including lasso, adaptive lasso, SCAD, and MCP.
We prove that our penalized variable selection methods with folded concave
penalties or adaptive lasso penalty enjoy the oracle property. Extensive
numerical experiments show that the proposed methods have satisfactory
empirical performance under various scenarios. The utility of the methods is
illustrated through an application to a genome-wide association study of age to
early childhood caries.
更多查看译文
AI 理解论文
溯源树
样例
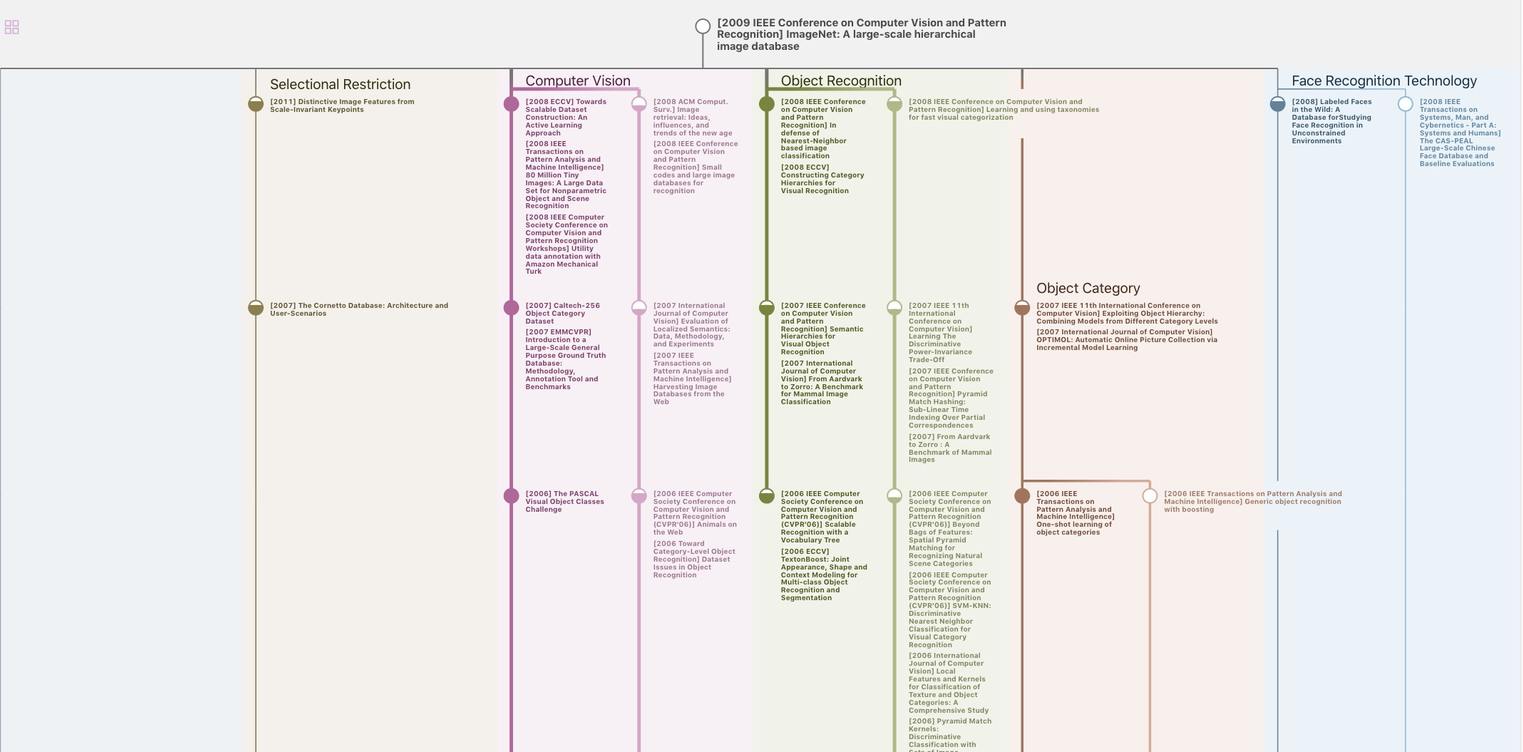
生成溯源树,研究论文发展脉络
Chat Paper
正在生成论文摘要